The probability flow ODE is provably fast
NeurIPS(2023)
摘要
We provide the first polynomial-time convergence guarantees for the probability flow ODE implementation (together with a corrector step) of score-based generative modeling. Our analysis is carried out in the wake of recent results obtaining such guarantees for the SDE-based implementation (i.e., denoising diffusion probabilistic modeling or DDPM), but requires the development of novel techniques for studying deterministic dynamics without contractivity. Through the use of a specially chosen corrector step based on the underdamped Langevin diffusion, we obtain better dimension dependence than prior works on DDPM ($O(\sqrt{d})$ vs. $O(d)$, assuming smoothness of the data distribution), highlighting potential advantages of the ODE framework.
更多查看译文
关键词
probability flow ode
AI 理解论文
溯源树
样例
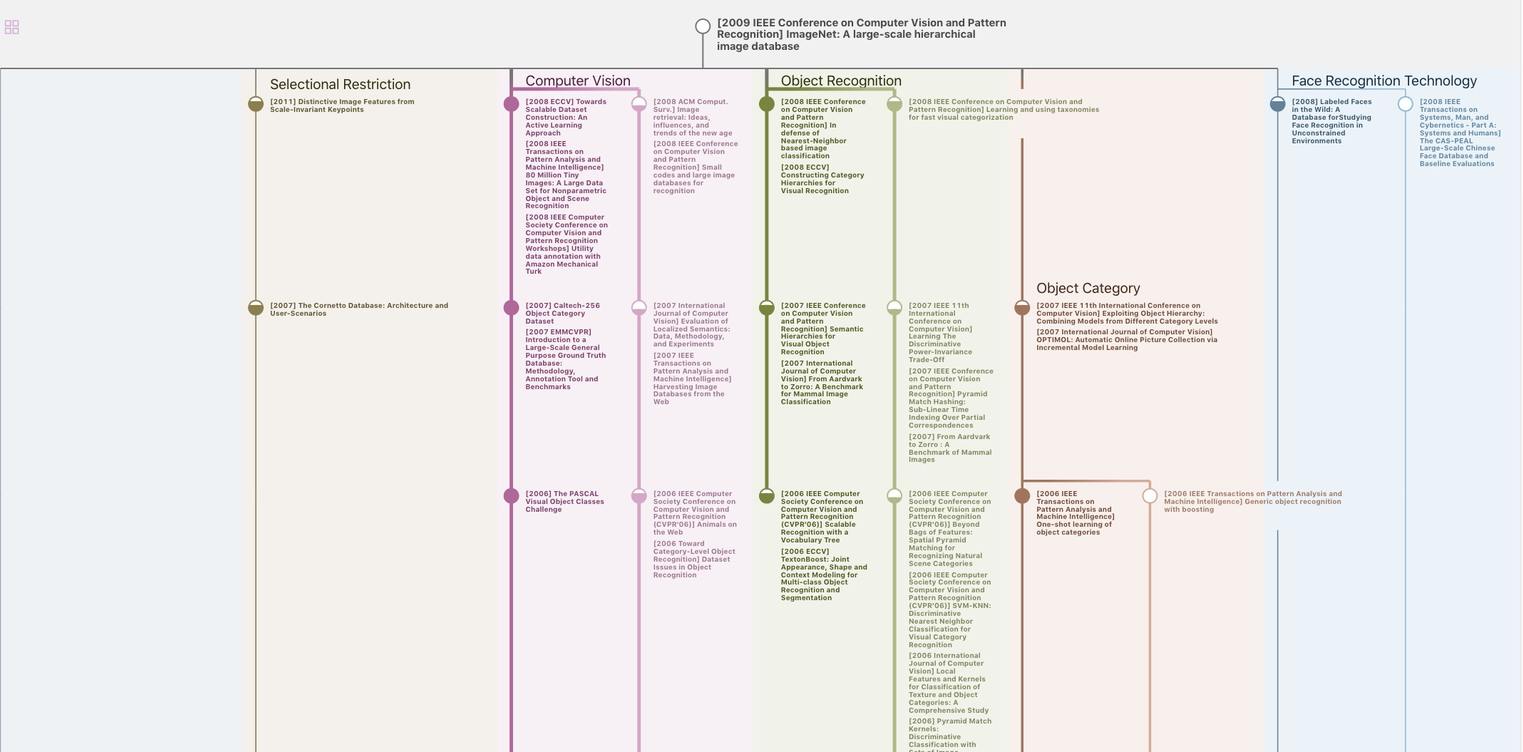
生成溯源树,研究论文发展脉络
Chat Paper
正在生成论文摘要