Differentially Private Adapters for Parameter Efficient Acoustic Modeling
CoRR(2023)
摘要
In this work, we devise a parameter-efficient solution to bring differential privacy (DP) guarantees into adaptation of a cross-lingual speech classifier. We investigate a new frozen pre-trained adaptation framework for DP-preserving speech modeling without full model fine-tuning. First, we introduce a noisy teacher-student ensemble into a conventional adaptation scheme leveraging a frozen pre-trained acoustic model and attain superior performance than DP-based stochastic gradient descent (DPSGD). Next, we insert residual adapters (RA) between layers of the frozen pre-trained acoustic model. The RAs reduce training cost and time significantly with a negligible performance drop. Evaluated on the open-access Multilingual Spoken Words (MLSW) dataset, our solution reduces the number of trainable parameters by 97.5% using the RAs with only a 4% performance drop with respect to fine-tuning the cross-lingual speech classifier while preserving DP guarantees.
更多查看译文
关键词
private adapters,parameter efficient acoustic
AI 理解论文
溯源树
样例
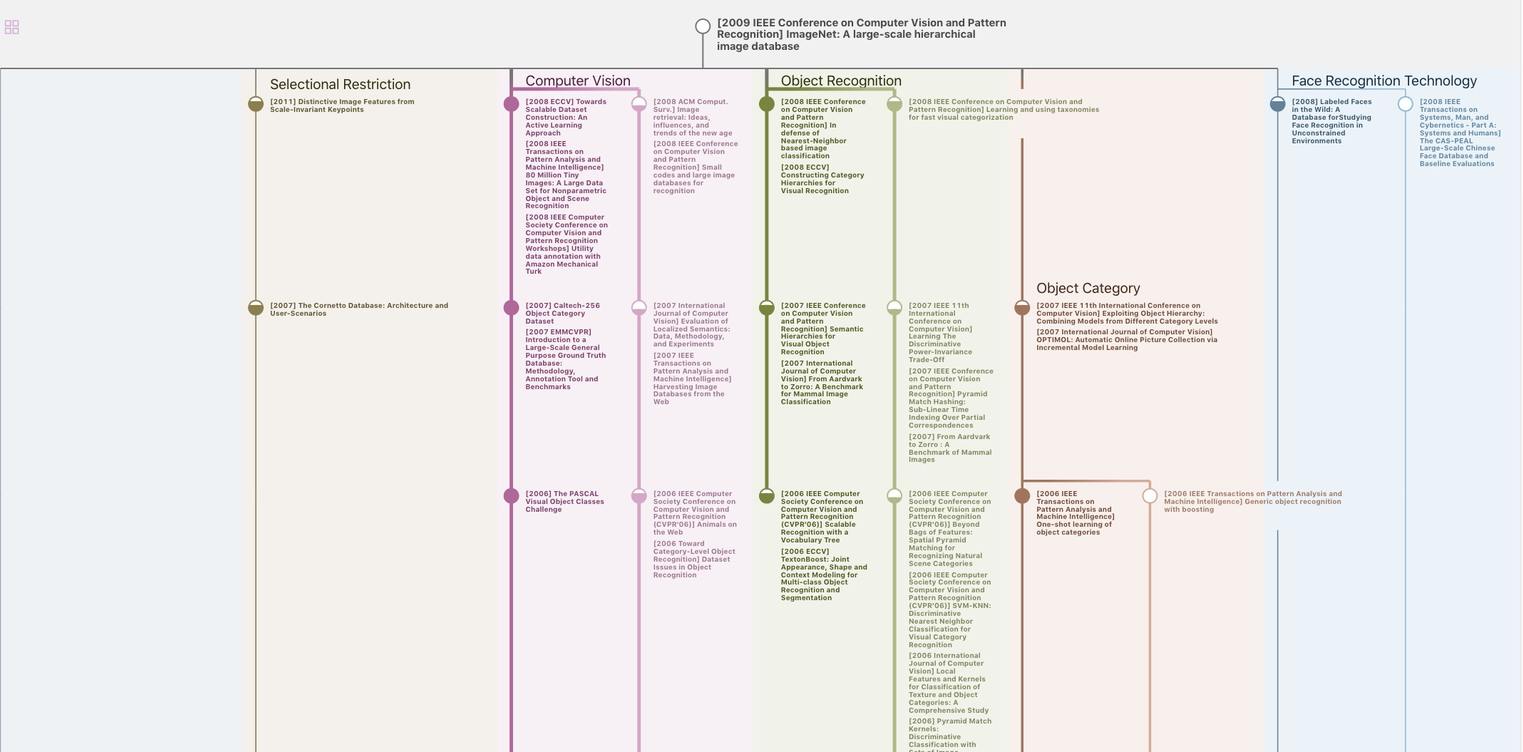
生成溯源树,研究论文发展脉络
Chat Paper
正在生成论文摘要