Classification of upper limb impairment in acute stroke patients using resting-state EEG markers and machine learning
2023 11th International IEEE/EMBS Conference on Neural Engineering (NER)(2023)
摘要
Motor impairment after stroke is typically assessed using clinical observational scales. However, these scales are often prone to intrinsic variability and are time consuming to administer. Electroencephalography (EEG) may be a useful complementary approach to objectively assess motor impairment after stroke, provided that explanatory markers are extracted from the raw signals. The present work assesses the capability of several EEG metrics in discriminating levels of upper limb impairment in a cross-validated machine learning framework. EEG signals were recorded from 28 acute stroke survivors within 72 hours after the stroke, along with the motor sub-score of the Fugl-Meyer assessment scale of the upper extremity (FMAUE). We extracted 221 features from the spectral and connectivity domains of the EEG signals and selected the most predictive features based on two feature ranking algorithms (ReliefF and maximum relevance minimum redundancy). Combinations of the best-performing features were fed to a support vector machine classifier, optimizing its hyperparameters. The best-performing model achieved a cross-validated accuracy higher than 85% in classifying participants into high- and low-FMAUE. The brain symmetry index and its derivatives were the most important predictors of patients' motor status. This result will pave the way for EEG-based automatic scoring of motor impairment after stroke.
更多查看译文
关键词
EEG,machine learning,stroke,upper limb impairment
AI 理解论文
溯源树
样例
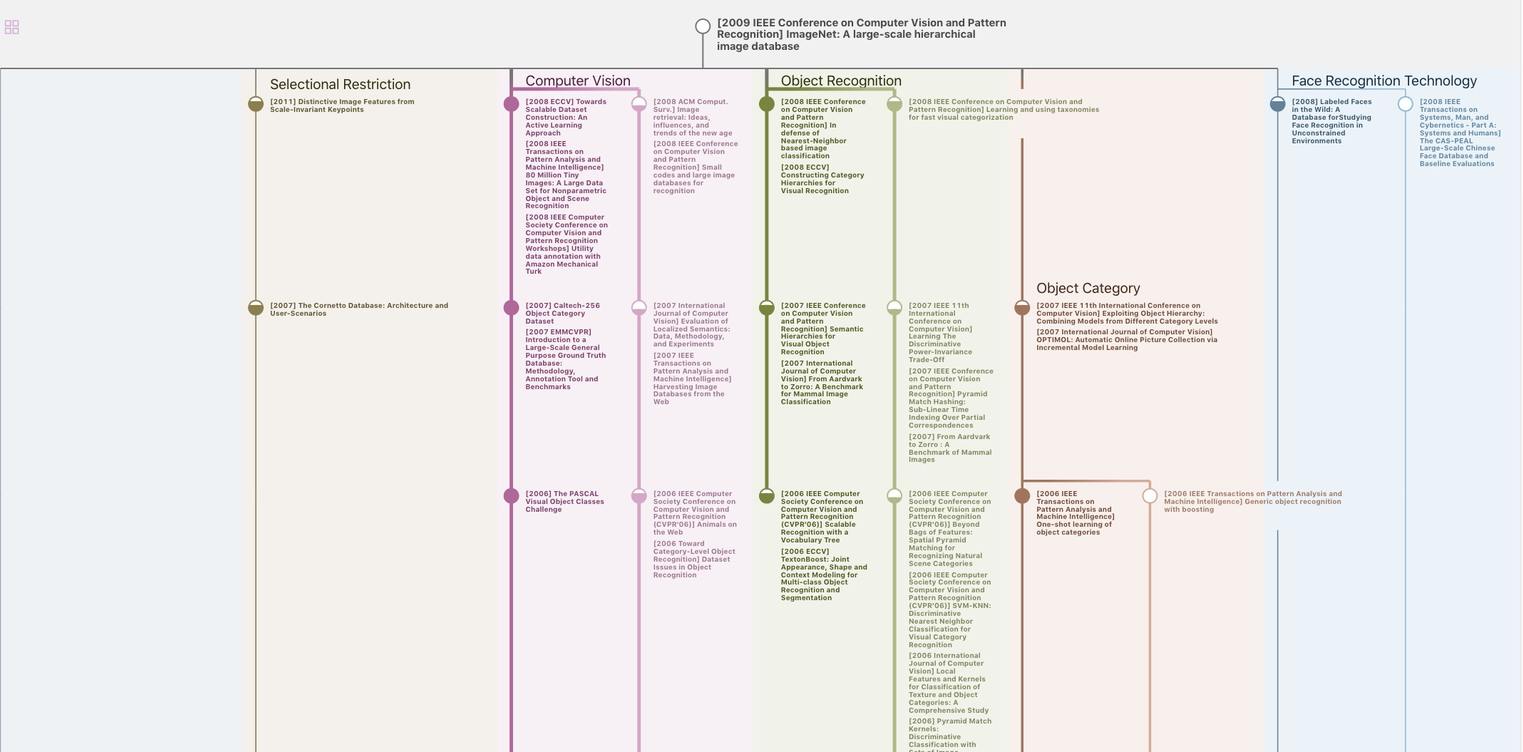
生成溯源树,研究论文发展脉络
Chat Paper
正在生成论文摘要