Energy-Efficient User Association in mmWave/THz Ultra-Dense Network via Multi-Agent Deep Reinforcement Learning
IEEE Transactions on Green Communications and Networking(2023)
摘要
As a key enabler for 5G and 6G wireless communications, millimeter-wave (mmWave) and terahertz (THz) ultra-dense network (UDN) has received a great deal of attention recently. The mmWave/THz UDN can greatly improve the capacity of cellular network by exploiting the reduced path loss of densified network and the wide range of frequency spectrum. In order to maximize the capacity gain of UDN, the associations between small base stations (SBSs) and users should be designed deliberately. However, since computational complexity and signaling overhead increase exponentially with the numbers of SBSs and users, it is very difficult to apply the conventional centralized approaches in the UDN. In this paper, we propose a decentralized user association technique based on multi-agent actor-critic (AC) to maximize the energy efficiency of UDN. In our technique, actor network determines the user association of the SBS using the local observation and critic network informs the energy-efficient user association decision to the actor network. In doing so, the DRL agent in each SBS can find out the user association decision maximizing the network energy efficiency. From the extensive simulations, we demonstrate that the proposed scheme achieves more than 50% average energy efficiency gain over the conventional user association techniques.
更多查看译文
关键词
mmWave,THz,UDN,energy efficiency,user association,multi-agent DRL,decentralized mechanism,cooper-ative training
AI 理解论文
溯源树
样例
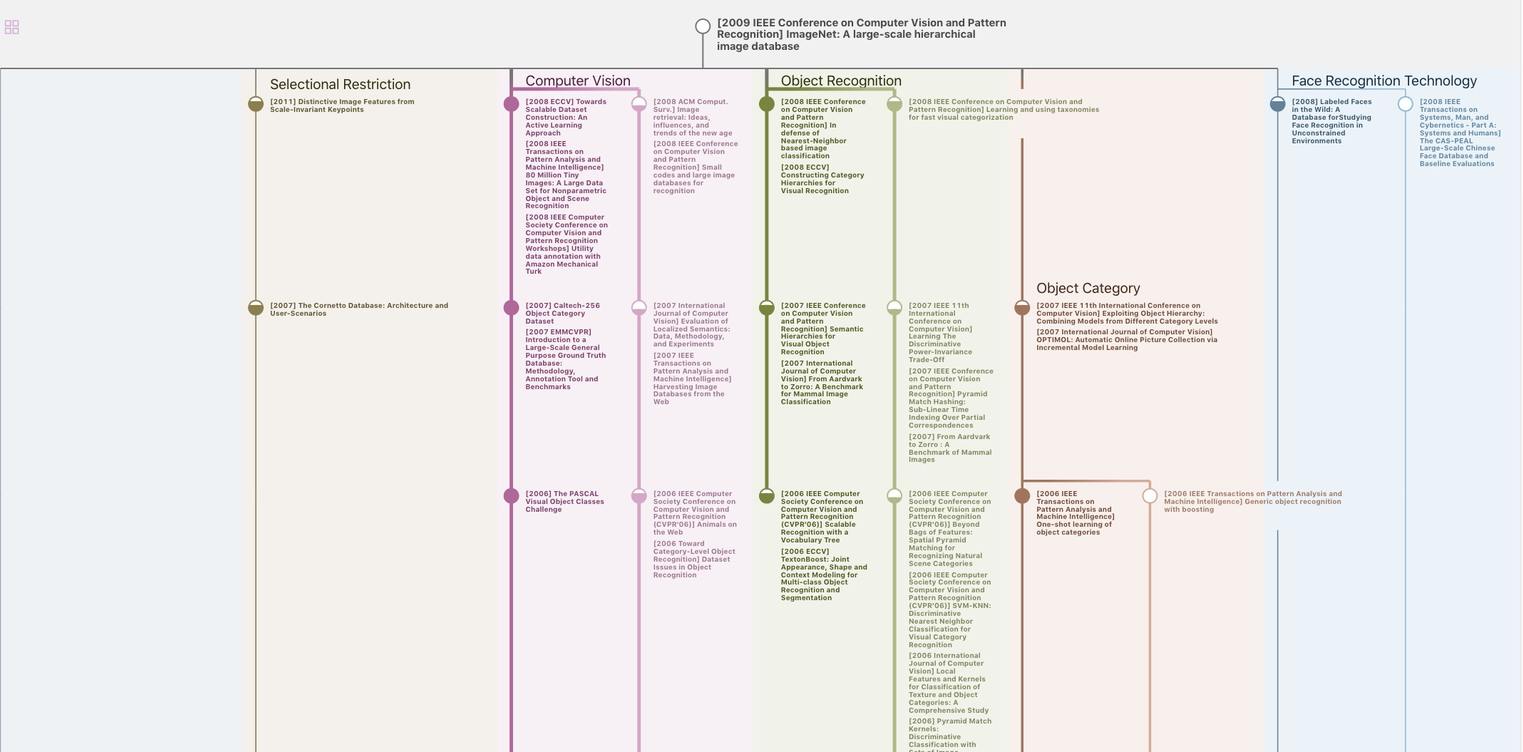
生成溯源树,研究论文发展脉络
Chat Paper
正在生成论文摘要