An Online Metric Learning-Based Open-Switch and Current Sensor Fault Diagnosis for MSPMSM Systems
IEEE Transactions on Power Electronics(2023)
摘要
Rapid and accurate removal of faulty sectors is the conventional fault tolerance method for highly secure multisector permanent magnet synchronous motors (MSPMSMs). In this article, a new fault diagnosis method for MSPMSMs based on metric learning (ML) is proposed. Its advantage is that it can complete fault detection and faulty sector location within 0.1 current fundamental cycle, which is faster than previous methods. Meanwhile, for the faulty sector, 28 types of open switch faults and current sensor faults can be accurately identified. In addition, since the fault diagnosis is based on structural characteristics, the proposed method is independent of system parameters and naturally robust to the speed and current variation. Particularly, using the consistent structural characteristics of MSPMSM sectors, an amplitude-trend distance (ATD) is presented to obviously reflect the difference of the same phase currents between healthy and faulty sectors. Subsequently, to ensure the speed of fault detection and high false alarm immunity, the ATD value under different fault states is theoretically analyzed, and a reasonable threshold is set. Compared to traditional ML methods, which requires complex computational classification methods, four characteristic parameters sector, polar, close to zero, and sensor flag are developed to locate the faulty sector and the faulty switch or current sensor. The experiment results on four-sector permanent magnet synchronous motor (PMSM) verify the effectiveness of the proposed fault diagnosis method.
更多查看译文
关键词
Amplitude-trend distance (ATD), current sensor fault, fault diagnosis, metric learning (ML), multisector permanent magnet synchronous motor (MSPMSM), open-switch (OS) fault
AI 理解论文
溯源树
样例
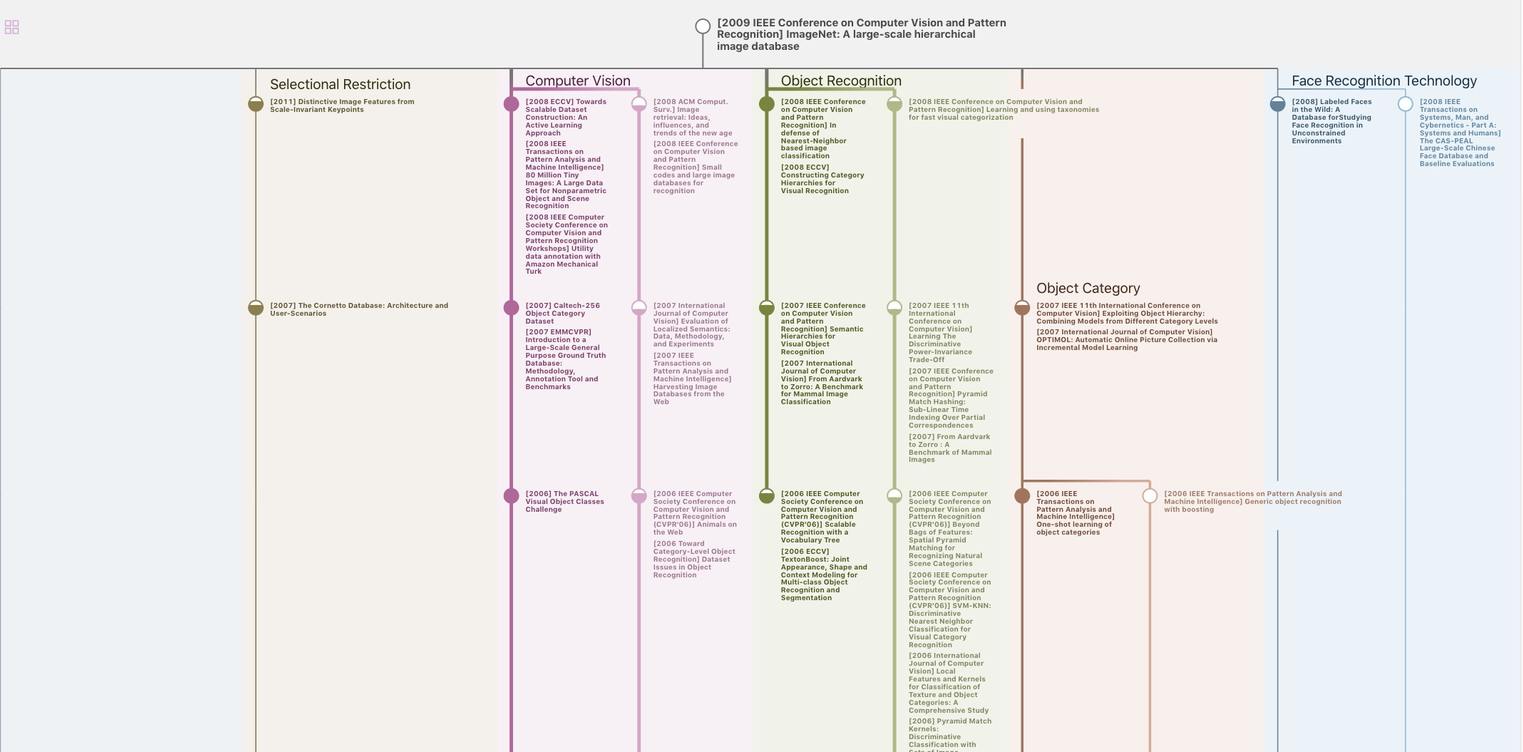
生成溯源树,研究论文发展脉络
Chat Paper
正在生成论文摘要