Augmenting aspect-level sentiment classification with distance-related local context input
The Journal of Supercomputing(2023)
摘要
Aspect-level sentiment classification is a fine-grained sentiment classification task to predict sentiment polarities of different aspects within sentences. Recently, attention mechanisms and graph neural networks over dependency tree have been explored to capture semantic and syntactic correlations between aspects and context words. More recently, some methods have been applied for modeling connections between aspects and their local context. However, these local context-based approaches heavily rely on specifically designed sophisticated networks. In this paper, we propose a simple yet effective local context input method to better leverage local context. In particular, two ways of generating local context input are explored: relative distance (Rel) and dependency distance (Dep) . Moreover, we utilize dual context input for the ALSC task, namely global context and local context, to integrate global context information and local context information. With a simple BERT + attention network, the experimental results on Restaurant and Laptop datasets of SemEval 2014 illustrate that our proposed model outperforms a range of BERT-based state-of-the-art models. Besides, we apply the dual context input to six widely used ALSC models, validating the generality and robustness of the proposed dual context input method.
更多查看译文
关键词
Aspect-level sentiment classification,Attention mechanism,Dependency tree,Local context input
AI 理解论文
溯源树
样例
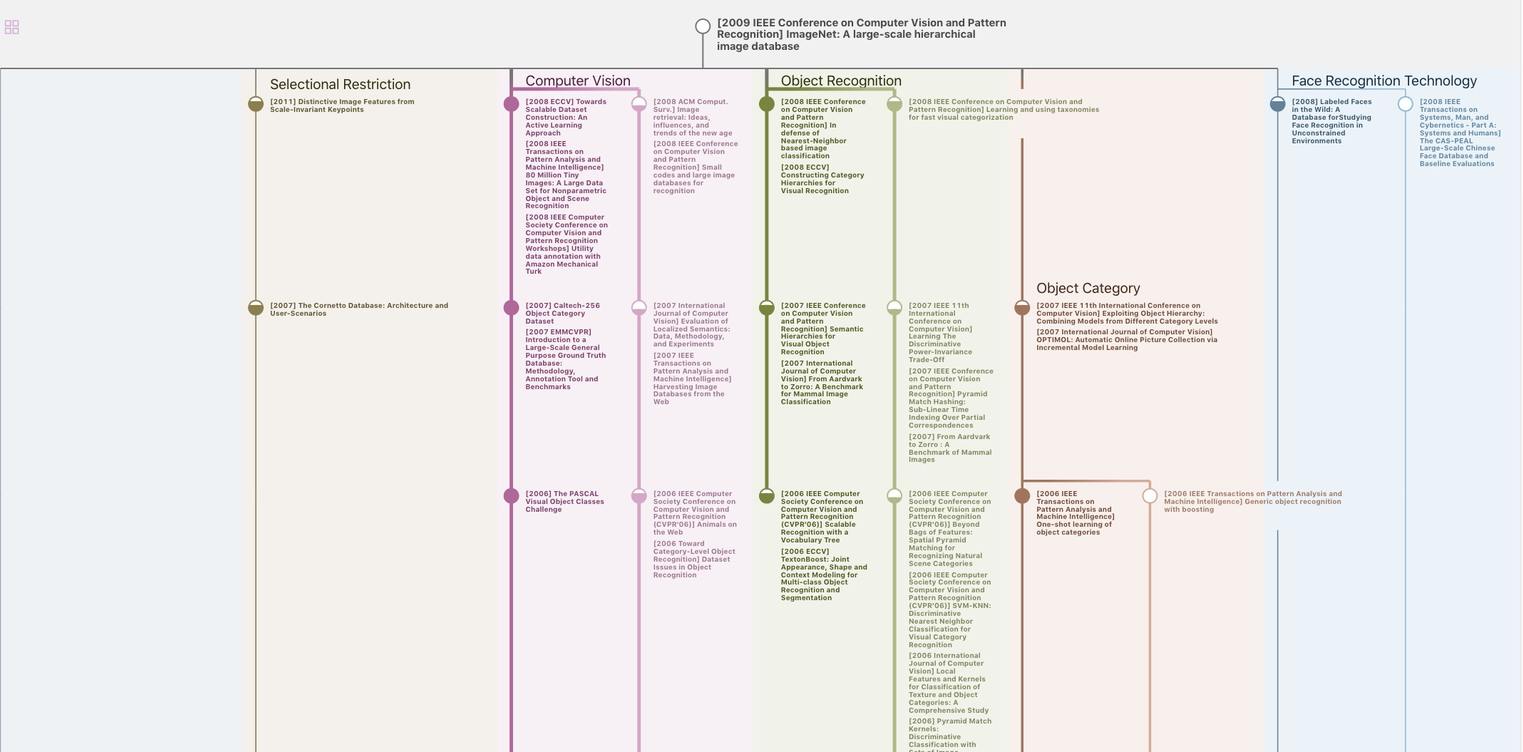
生成溯源树,研究论文发展脉络
Chat Paper
正在生成论文摘要