A survey of deep learning-based 3D shape generation
Computational Visual Media(2023)
摘要
Deep learning has been successfully used for tasks in the 2D image domain. Research on 3D computer vision and deep geometry learning has also attracted attention. Considerable achievements have been made regarding feature extraction and discrimination of 3D shapes. Following recent advances in deep generative models such as generative adversarial networks, effective generation of 3D shapes has become an active research topic. Unlike 2D images with a regular grid structure, 3D shapes have various representations, such as voxels, point clouds, meshes, and implicit functions. For deep learning of 3D shapes, shape representation has to be taken into account as there is no unified representation that can cover all tasks well. Factors such as the representativeness of geometry and topology often largely affect the quality of the generated 3D shapes. In this survey, we comprehensively review works on deep-learning-based 3D shape generation by classifying and discussing them in terms of the underlying shape representation and the architecture of the shape generator. The advantages and disadvantages of each class are further analyzed. We also consider the 3D shape datasets commonly used for shape generation. Finally, we present several potential research directions that hopefully can inspire future works on this topic.
更多查看译文
关键词
3D representations,geometry learning,generative models,deep learning
AI 理解论文
溯源树
样例
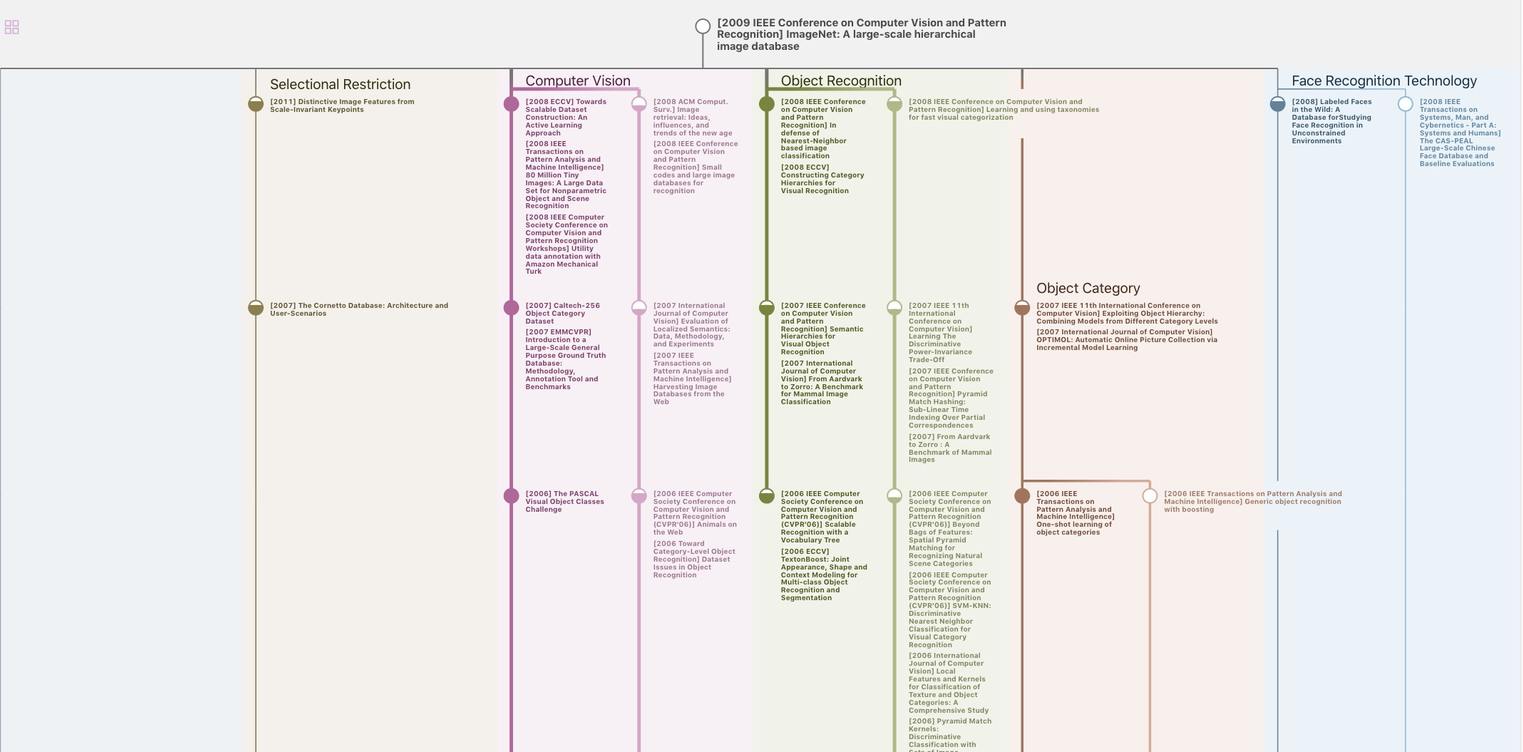
生成溯源树,研究论文发展脉络
Chat Paper
正在生成论文摘要