Label enhancement via joint implicit representation clustering
IJCAI 2023(2023)
摘要
Label distribution is an effective label form for representing label polysemy (i.e., the cases where an instance can be described by multiple labels simultaneously). However, the expensive annotating cost of label distributions limits their application to a wide range of practical tasks. Hence, LE (label enhancement) techniques have been extensively studied to solve this problem. Existing LE algorithms mostly estimate label distributions by the instance relation or label relation. However, they suffer from biased instance relations, limited model capabilities, or suboptimal local label correlations. Therefore, in this paper, we propose a deep generative model called JRC to simultaneously learn and cluster the joint implicit representations of both features and labels, which can be used to improve any existing LE algorithm involving the instance relation or local label correlations. Besides, we develop a novel label distribution recovery module, and then integrate it with JRC model, thus constituting a novel generative label enhancement model that utilizes the learned joint implicit representations and instance clusters in a principled manner. Finally, extensive experiments validate our proposal.
更多查看译文
AI 理解论文
溯源树
样例
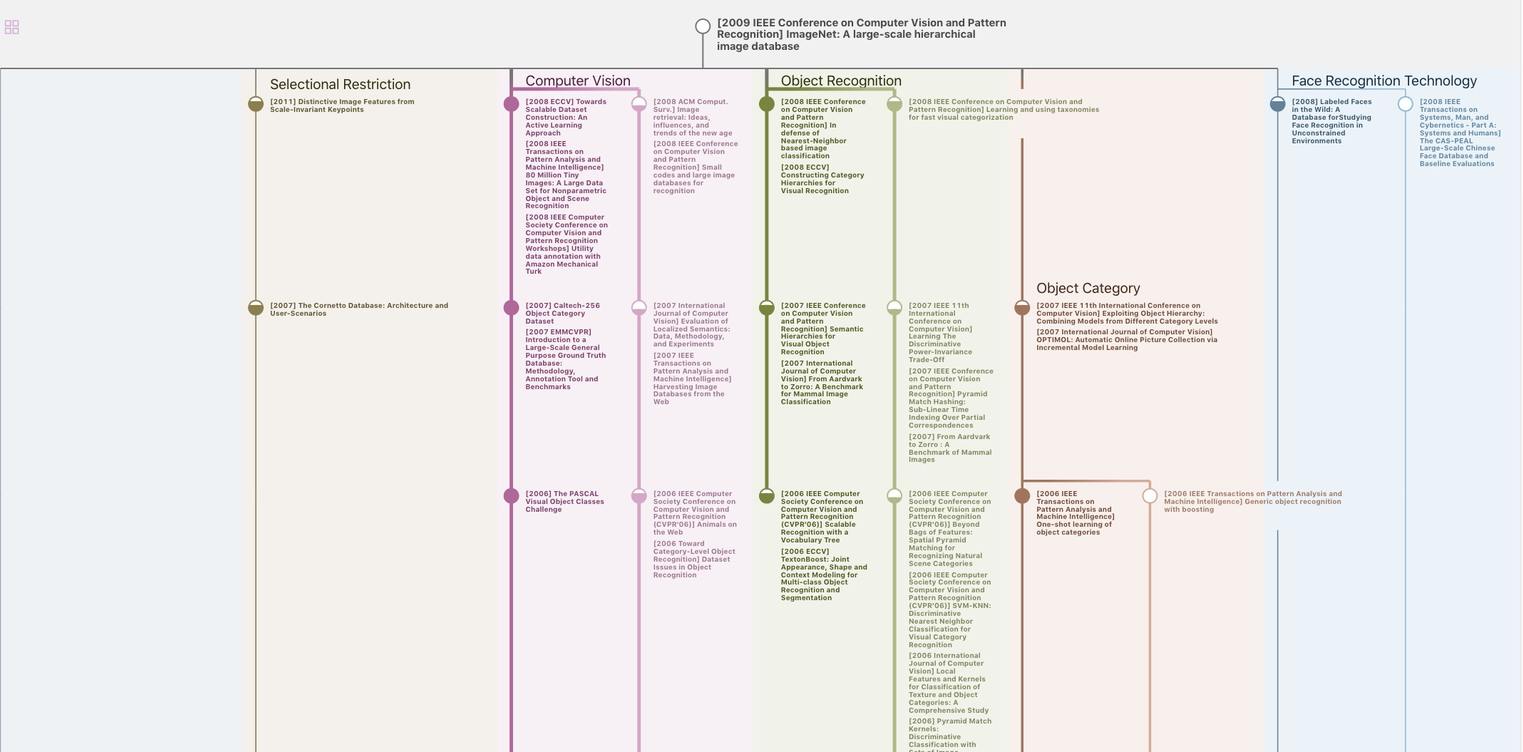
生成溯源树,研究论文发展脉络
Chat Paper
正在生成论文摘要