Generating Space-based SDO/HMI-like Solar Magnetograms from Ground-based H Images by Deep Learning
The Astrophysical Journal Supplement Series(2023)
摘要
Recently, the method of estimating magnetic field through monochromatic images by deep learning has been proposed, demonstrating good morphological similarity but somewhat poor magnetic polarity consistency relative to real observation. In this paper, we propose to estimate magnetic field from H alpha images by using a conditional generative adversarial network (cGAN) as the basic framework. The H alpha images from the Global Oscillation Network Group are used as the inputs and the line-of-sight magnetograms of the Helioseismic Magnetic Imager (HMI) are used as the targets. First, we train a cGAN model (Model A) with shuffling training data. However, the estimated magnetic polarities are not very consistent with real observations. Second, to improve the accuracy of estimated magnetic polarities, we train a cGAN model (Model B) with the chronological H alpha and HMI images, which can implicitly exploit the magnetic polarity constraint of time-series observation to generate more accurate magnetic polarities. We compare the generated magnetograms with the target HMI magnetograms to evaluate the two models. It can be observed that Model B has better magnetic polarity consistency than Model A. To quantitatively measure this consistency, we propose a new metric called pixel-to-pixel polarity accuracy (PPA). With respect to PPA, Model B is superior to Model A. This work gives us an insight that the time-series constraint can be implicitly exploited through organizing training data chronologically, and this conclusion also can be applied to other similar tasks related to time-series data.
更多查看译文
关键词
Solar magnetic fields,Convolutional neural networks
AI 理解论文
溯源树
样例
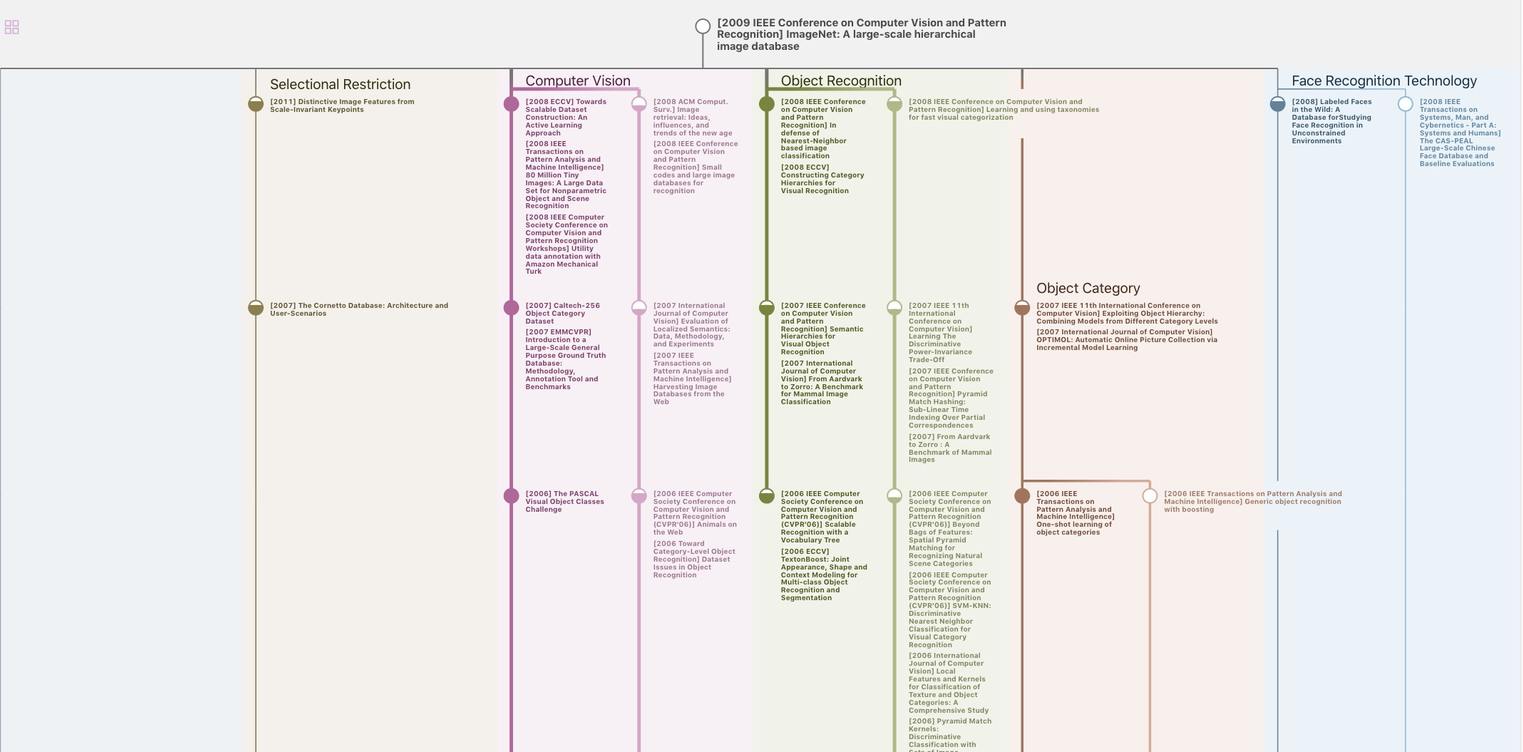
生成溯源树,研究论文发展脉络
Chat Paper
正在生成论文摘要