Small noise analysis for Tikhonov and RKHS regularizations
CoRR(2023)
摘要
Regularization plays a pivotal role in ill-posed machine learning and inverse problems. However, the fundamental comparative analysis of various regularization norms remains open. We establish a small noise analysis framework to assess the effects of norms in Tikhonov and RKHS regularizations, in the context of ill-posed linear inverse problems with Gaussian noise. This framework studies the convergence rates of regularized estimators in the small noise limit and reveals the potential instability of the conventional L2-regularizer. We solve such instability by proposing an innovative class of adaptive fractional RKHS regularizers, which covers the L2 Tikhonov and RKHS regularizations by adjusting the fractional smoothness parameter. A surprising insight is that over-smoothing via these fractional RKHSs consistently yields optimal convergence rates, but the optimal hyper-parameter may decay too fast to be selected in practice.
更多查看译文
关键词
rkhs regularizations,small noise analysis,tikhonov
AI 理解论文
溯源树
样例
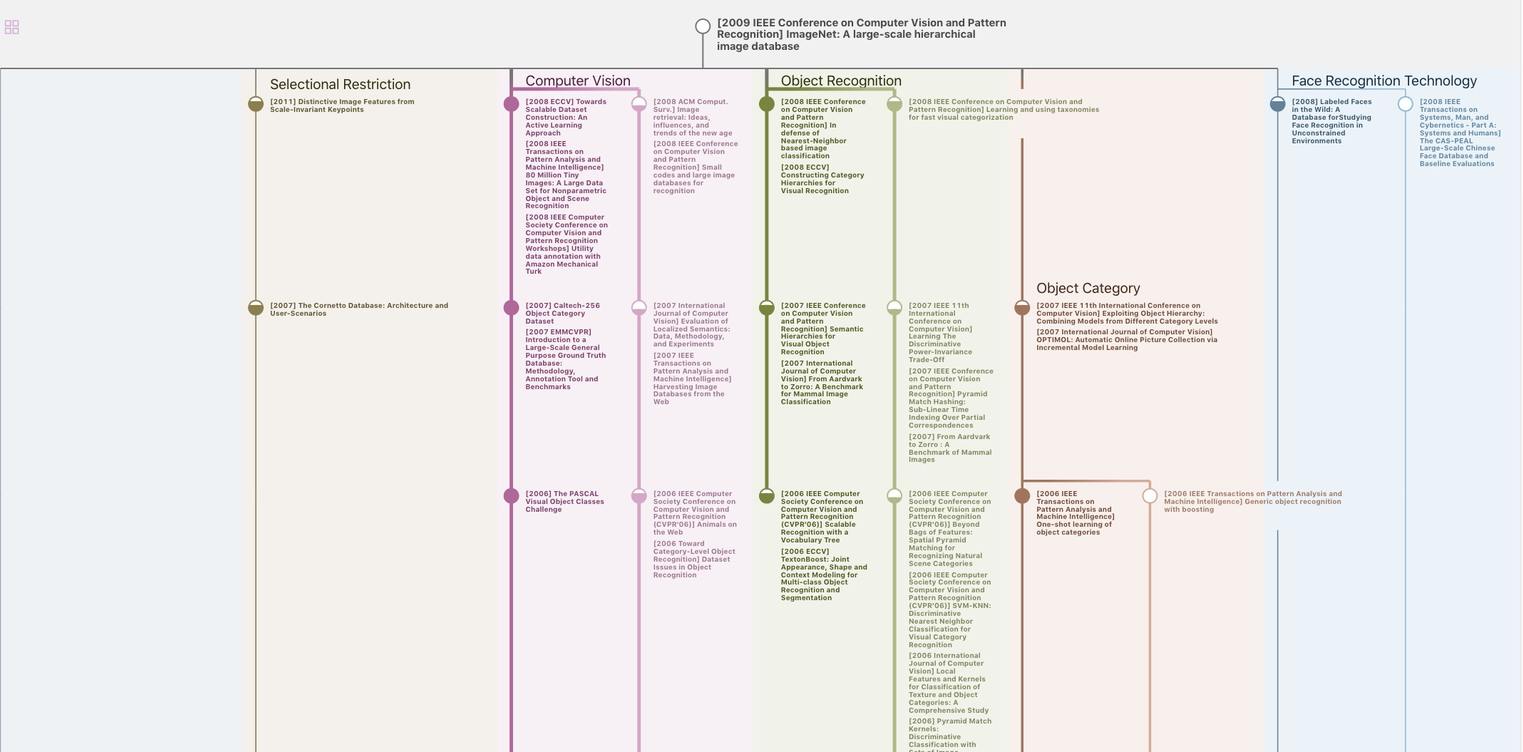
生成溯源树,研究论文发展脉络
Chat Paper
正在生成论文摘要