Generalised likelihood profiles for models with intractable likelihoods
STATISTICS AND COMPUTING(2024)
摘要
Likelihood profiling is an efficient and powerful frequentist approach for parameter estimation, uncertainty quantification and practical identifiablity analysis. Unfortunately, these methods cannot be easily applied for stochastic models without a tractable likelihood function. Such models are typical in many fields of science, rendering these classical approaches impractical in these settings. To address this limitation, we develop a new approach to generalising the methods of likelihood profiling for situations when the likelihood cannot be evaluated but stochastic simulations of the assumed data generating process are possible. Our approach is based upon recasting developments from generalised Bayesian inference into a frequentist setting. We derive a method for constructing generalised likelihood profiles and calibrating these profiles to achieve desired frequentist coverage for a given coverage level. We demonstrate the performance of our method on realistic examples from the literature and highlight the capability of our approach for the purpose of practical identifability analysis for models with intractable likelihoods.
更多查看译文
关键词
Generalised likelihood,Intractable likelihood,Simulation-based inference,Profiling,Parametric bootstrap
AI 理解论文
溯源树
样例
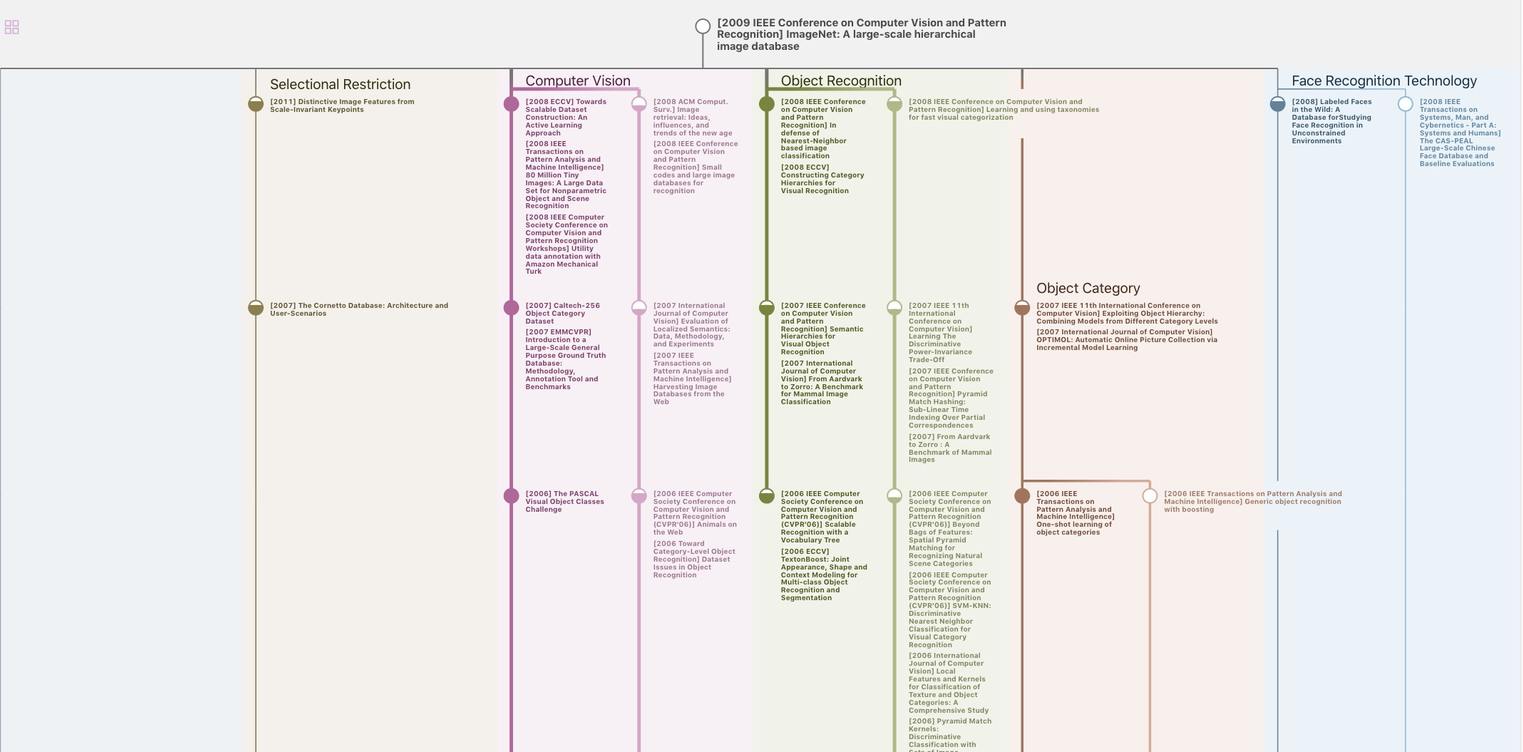
生成溯源树,研究论文发展脉络
Chat Paper
正在生成论文摘要