Generalization Bounds for Neural Belief Propagation Decoders
CoRR(2023)
摘要
Machine learning based approaches are being increasingly used for designing decoders for next generation communication systems. One widely used framework is neural belief propagation (NBP), which unfolds the belief propagation (BP) iterations into a deep neural network and the parameters are trained in a data-driven manner. NBP decoders have been shown to improve upon classical decoding algorithms. In this paper, we investigate the generalization capabilities of NBP decoders. Specifically, the generalization gap of a decoder is the difference between empirical and expected bit-error-rate(s). We present new theoretical results which bound this gap and show the dependence on the decoder complexity, in terms of code parameters (blocklength, message length, variable/check node degrees), decoding iterations, and the training dataset size. Results are presented for both regular and irregular parity-check matrices. To the best of our knowledge, this is the first set of theoretical results on generalization performance of neural network based decoders. We present experimental results to show the dependence of generalization gap on the training dataset size, and decoding iterations for different codes.
更多查看译文
关键词
Machine Learning,neural belief propagation,generalization gap,decoder complexity,code parameters,regular and irregular parity-check matrices
AI 理解论文
溯源树
样例
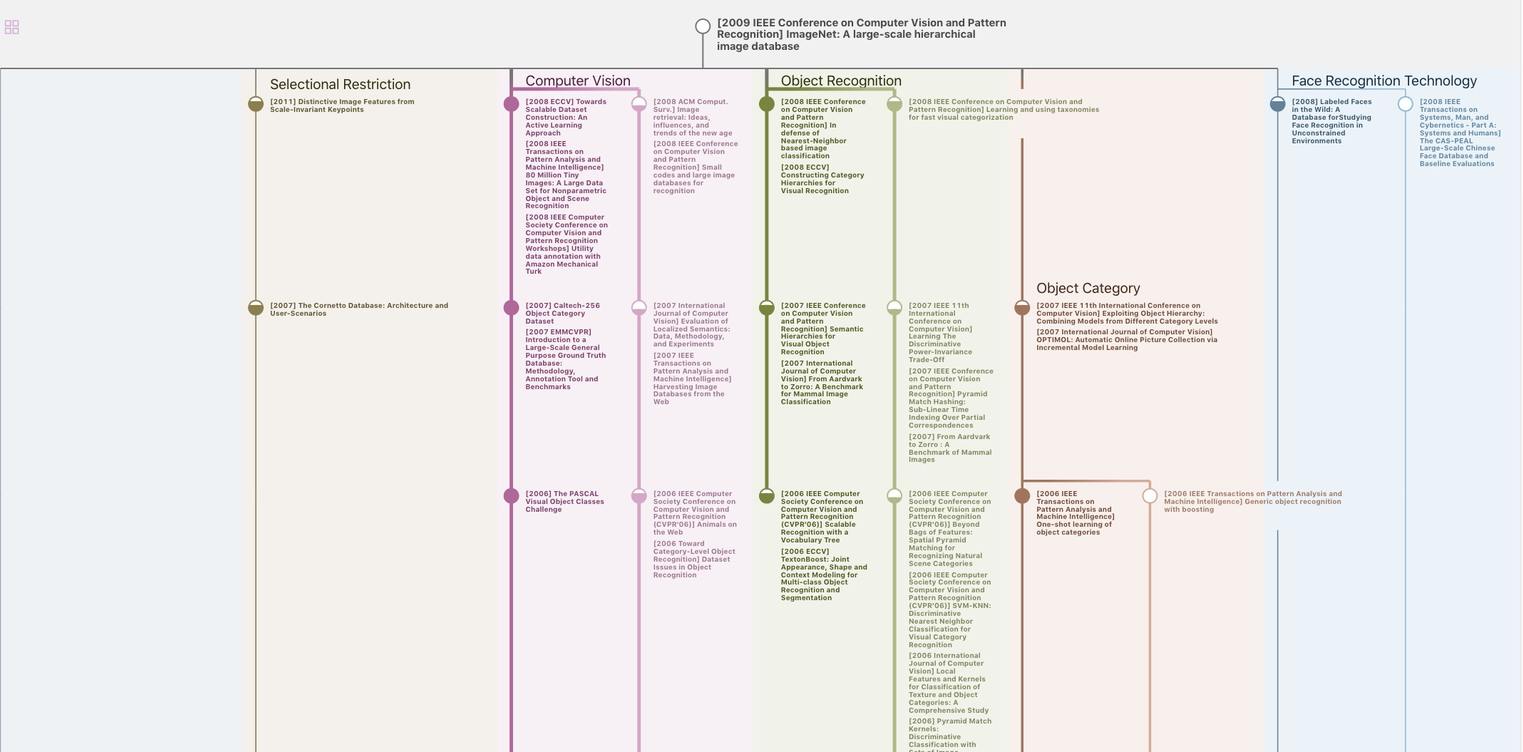
生成溯源树,研究论文发展脉络
Chat Paper
正在生成论文摘要