DCLL—A Deep Network for Possible Real-Time Decoding of Imagined Words
International Symposium on Intelligent Informatics(2023)
摘要
We present a novel architecture for classifying imagined words from electroencephalogram (EEG) captured during speech imagery. The proposed architecture employs a sliding window with overlap for data augmentation (DA) and common spatial pattern (CSP) in order to derive the features. The dimensionality of features is reduced using linear discriminant analysis (LDA). Long short-term memory (LSTM) along with majority voting is used as the classifier. We call the proposed architecture the DCLL (DA-CSP-LDA-LSTM) architecture. On a publicly available imagined word dataset, the DCLL architecture achieves an accuracy of 85.2% for classifying the imagined words “in” and “cooperate”. Although this is around 7% less than the best result in the literature on this dataset, the DCLL architecture is roughly 300 times faster than the latter, making it a potential candidate for imagined word-based online BCI systems where the EEG signal needs to be classified in real time.
更多查看译文
关键词
deep network,words,real-time
AI 理解论文
溯源树
样例
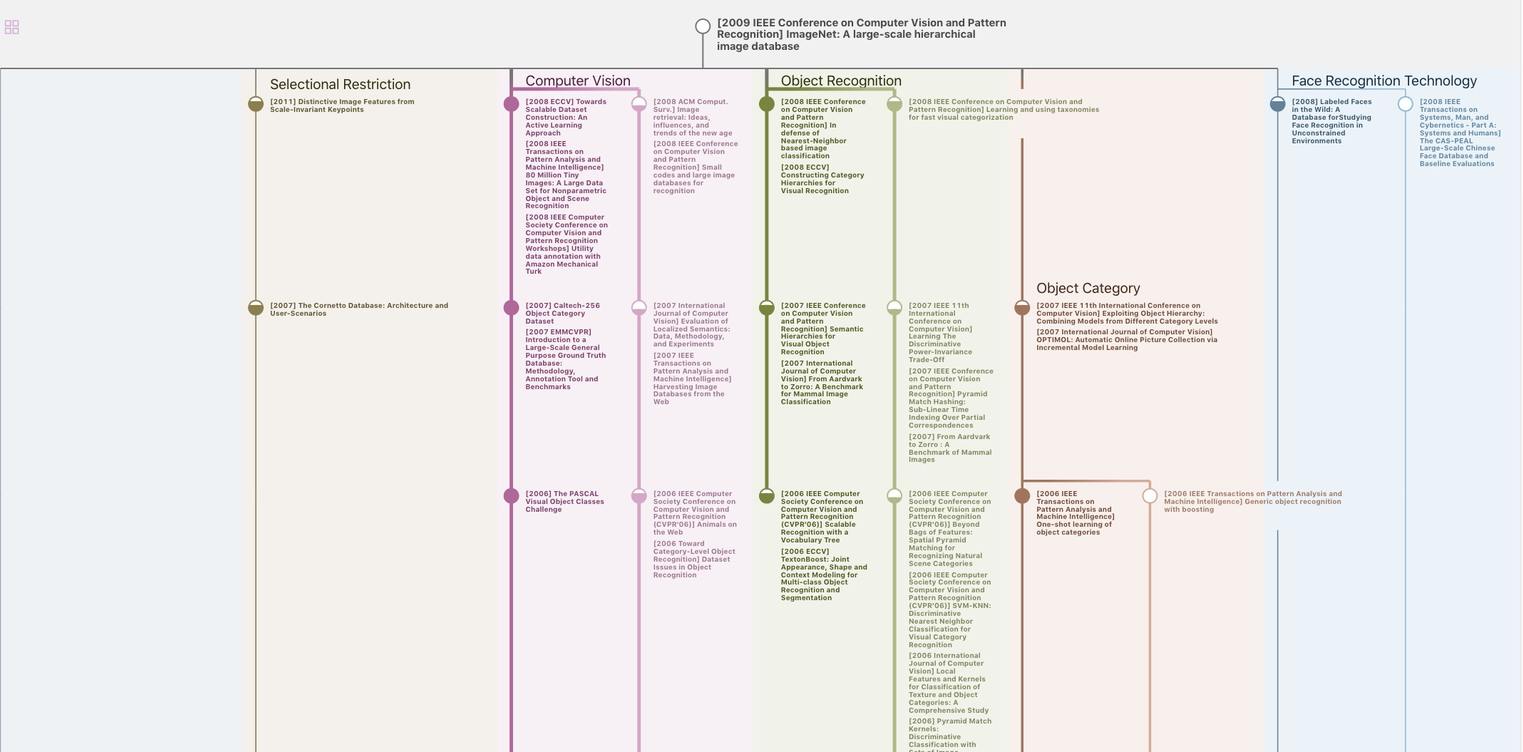
生成溯源树,研究论文发展脉络
Chat Paper
正在生成论文摘要