A Reconfigurable 2D-Convolution Accelerator for DNNs Quantized with Mixed-Precision
Applications in Electronics Pervading Industry, Environment and Society(2023)
摘要
Mixed-precision uses in each layer of a Deep Neural Network the minimum bit-width that preserves accuracy. In this context, our new Reconfigurable 2D-Convolution Module (RCM) computes N = 1, 2 or 4 Multiply-and-Accumulate operations in parallel with configurable precision from 1 to 16/N bits. Our design-space exploration via high-level synthesis obtains the best points in the latency vs area space, varying the size of the tensor tile handled by our RCM and its parallelism. A comparison with a non-configurable module on a 28-nm technology shows many reconfigurable Pareto points for low bit-width configurations, making our RCM a promising mixed-precision accelerator for inference.
更多查看译文
关键词
2D-Convolution, Reconfigurable Accelerator, Mixed-Precision
AI 理解论文
溯源树
样例
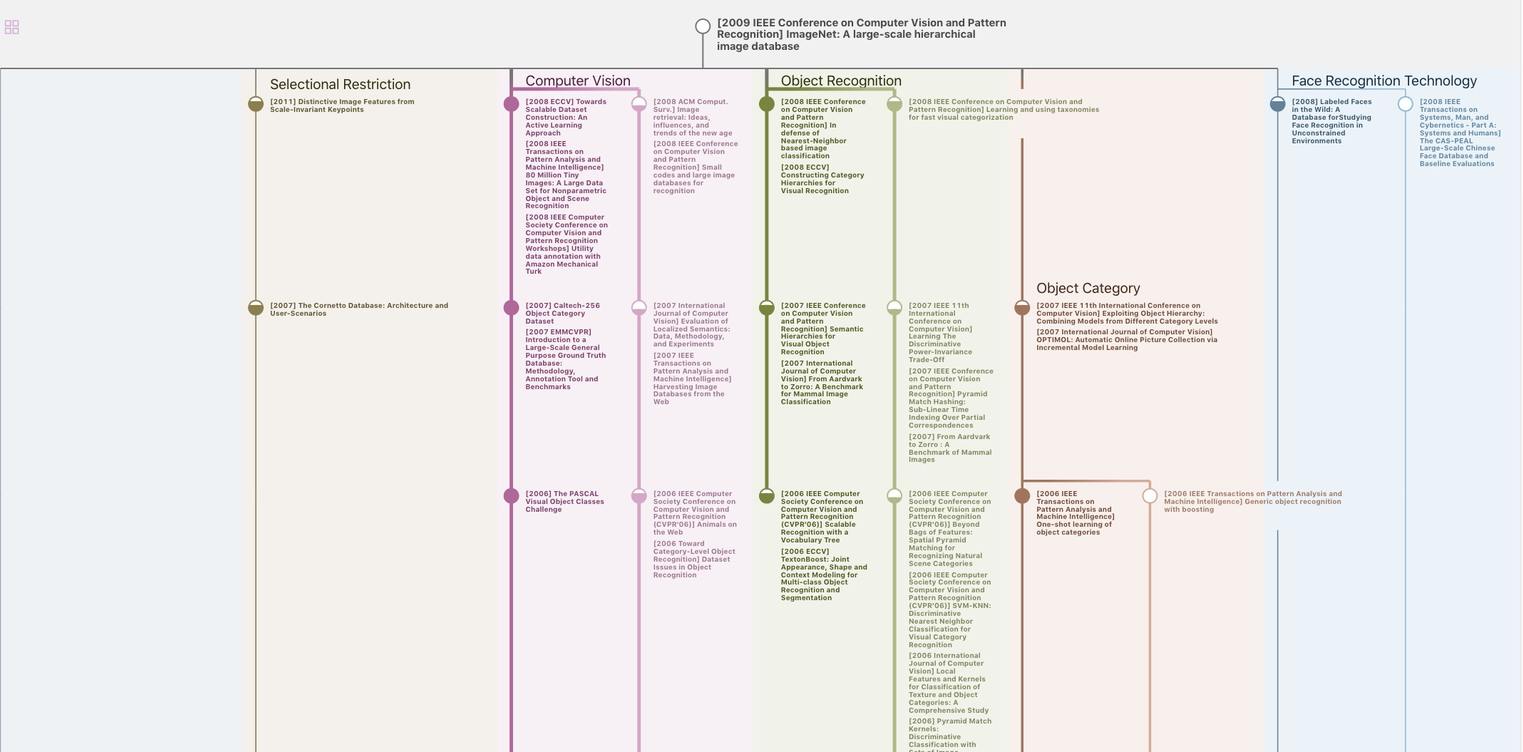
生成溯源树,研究论文发展脉络
Chat Paper
正在生成论文摘要