Feature Selection Using Hybrid Black Hole Genetic Algorithm in Multi-label Datasets
Soft Computing for Problem Solving(2023)
摘要
In multi-label classification, each instance is assigned to a group of labels. Due to its expanding use in applications across domains, multi-label classification has gained prominence in recent years. Feature selection is the most common and important preprocessing step in all machine learning and data mining tasks. Removal of highly correlated, irrelevant, and noisy features increases the performance of an algorithm and reduces the computational time. Recently, a Black Hole metaheuristic algorithm, inspired by the phenomenon of Black Holes, has been developed. In this study, we present a modified standalone Black Hole algorithm by hybridizing the standard Black Hole algorithm with two genetic algorithm operators, namely crossover and mutation operators. We carried out experiments on several benchmarking datasets across domains. The modified feature selection algorithm was also employed on a multi-label epitope dataset. The results were compared with the standalone Black Hole and other most common evolutionary algorithms such as Ant Colony, Advance Ant Colony, genetic algorithms, and Binary Gravitational Search Algorithm. The results showed that our improved hybrid algorithm outperforms the existing evolutionary algorithms on most datasets. The synergistic combination of Black Hole and Genetic Algorithms can be used to solve multi-label classification problems in different domains.
更多查看译文
关键词
Black hole, Genetic algorithms, Feature selection
AI 理解论文
溯源树
样例
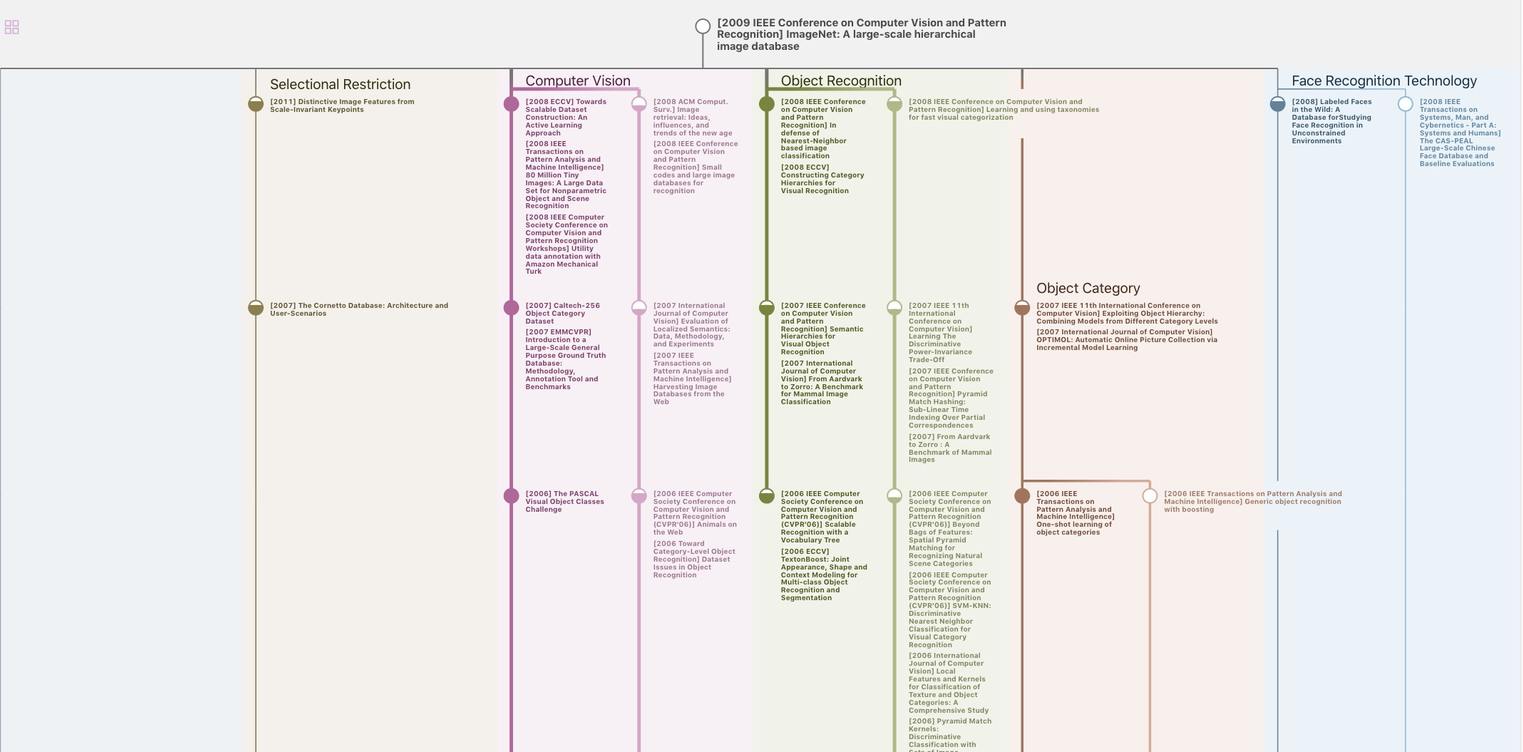
生成溯源树,研究论文发展脉络
Chat Paper
正在生成论文摘要