K-fold Semi-supervised Self-learning Technique for Image Disease Localization
Computational Vision and Bio-Inspired Computing(2023)
摘要
The unlabeled data can be annotated with the help of semi-supervised learning (SSL) algorithms like self-learning SSL algorithms, graph-based SSL algorithms, or the low-density separations. In this article, we have applied a self-learning semi-supervised technique with k-fold iterative training mechanism to localize cardiomegaly from the chest X-ray images. The purpose of using the iterative training method instead of the traditional self-learning semi-supervised algorithm was to generate more labeled images, increase the robustness of the algorithm and induce faster learning. The model was trained over a single-shot detector bounding box algorithm with a VGG-16 backbone for predicting the bounding box coordinates. This method generates a significant improvement in validation loss with an increase in the labeled dataset size by around 42 times compared to the traditionally used self-learning semi-supervised technique trained for the same number of epochs.
更多查看译文
关键词
image disease localization,k-fold,semi-supervised,self-learning
AI 理解论文
溯源树
样例
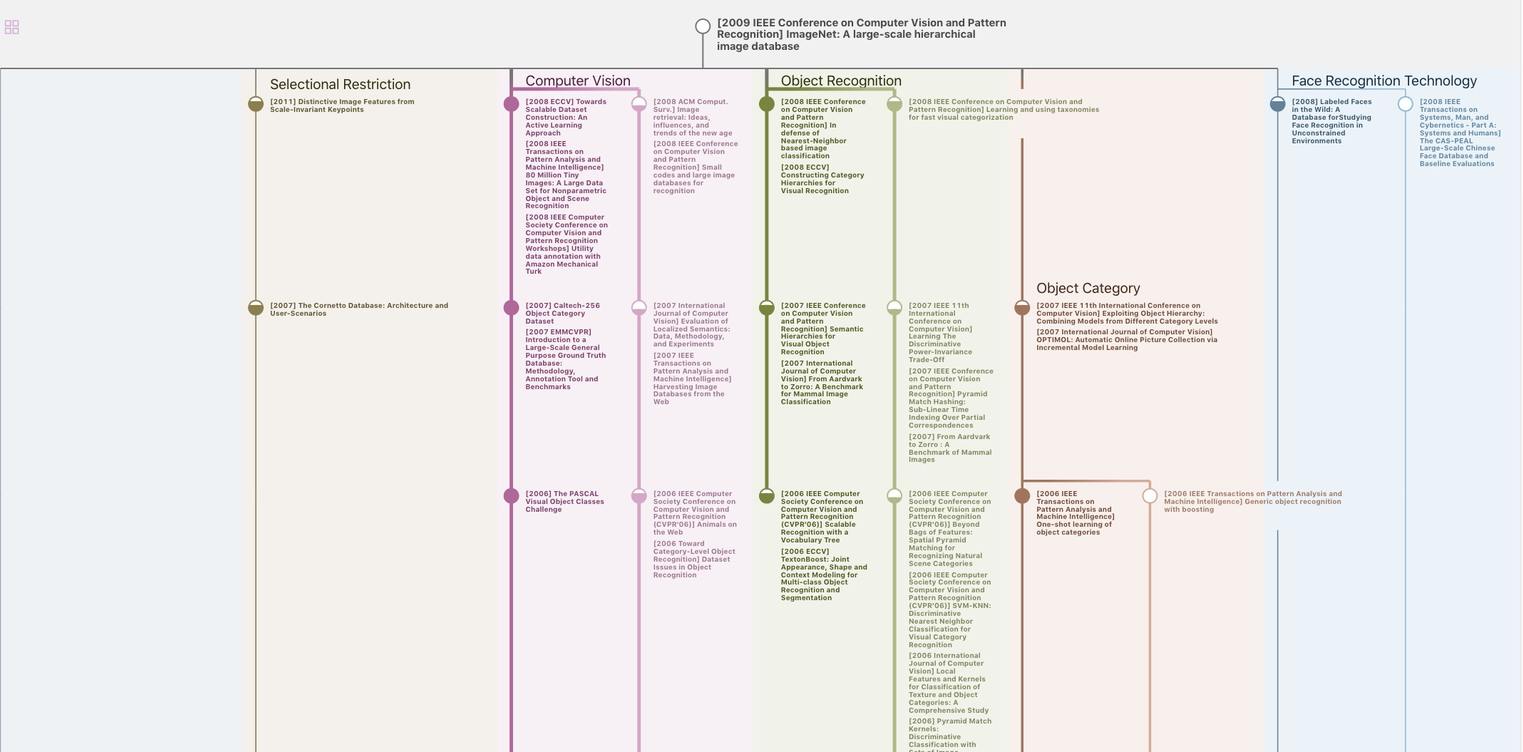
生成溯源树,研究论文发展脉络
Chat Paper
正在生成论文摘要