Glioma Segmentation in MR Images Using 2D Double U-Net: An Empirical Investigation
Innovations in Computer Science and Engineering(2023)
摘要
Glioma develops inside the brain. Automatic glioma segmentation reduces computational time and increases survival owing to previous treatment preparations—2D double U-Net segments glioblastoma from multi-modal MRI images (MRI). 2D brain models need fewer computational resources than 3D models. Residual U-Net (RU-Net) and dilated convolution U-Net make up the Double U-Net design (RU-Net). RU-residual Net’s route contains three 33 convolution kernels. DU-Net contains three 33 and 55 convolution kernels with a two dilation rate. RU-Net is more uncomplicated to optimise than deep networks and makes fewer training mistakes than deep networks. DU-Net gathers contextual information and creates details. The suggested model was assessed using the BraTS 2017 training dataset and attained dice similarity coefficients of 0.91, 0.85, and 0.88 for total, enhanced, and tumour core (TC), respectively.
更多查看译文
关键词
Deep learning, Glioma, Tumour, Segmentation, U-Net, Residual, Dilation
AI 理解论文
溯源树
样例
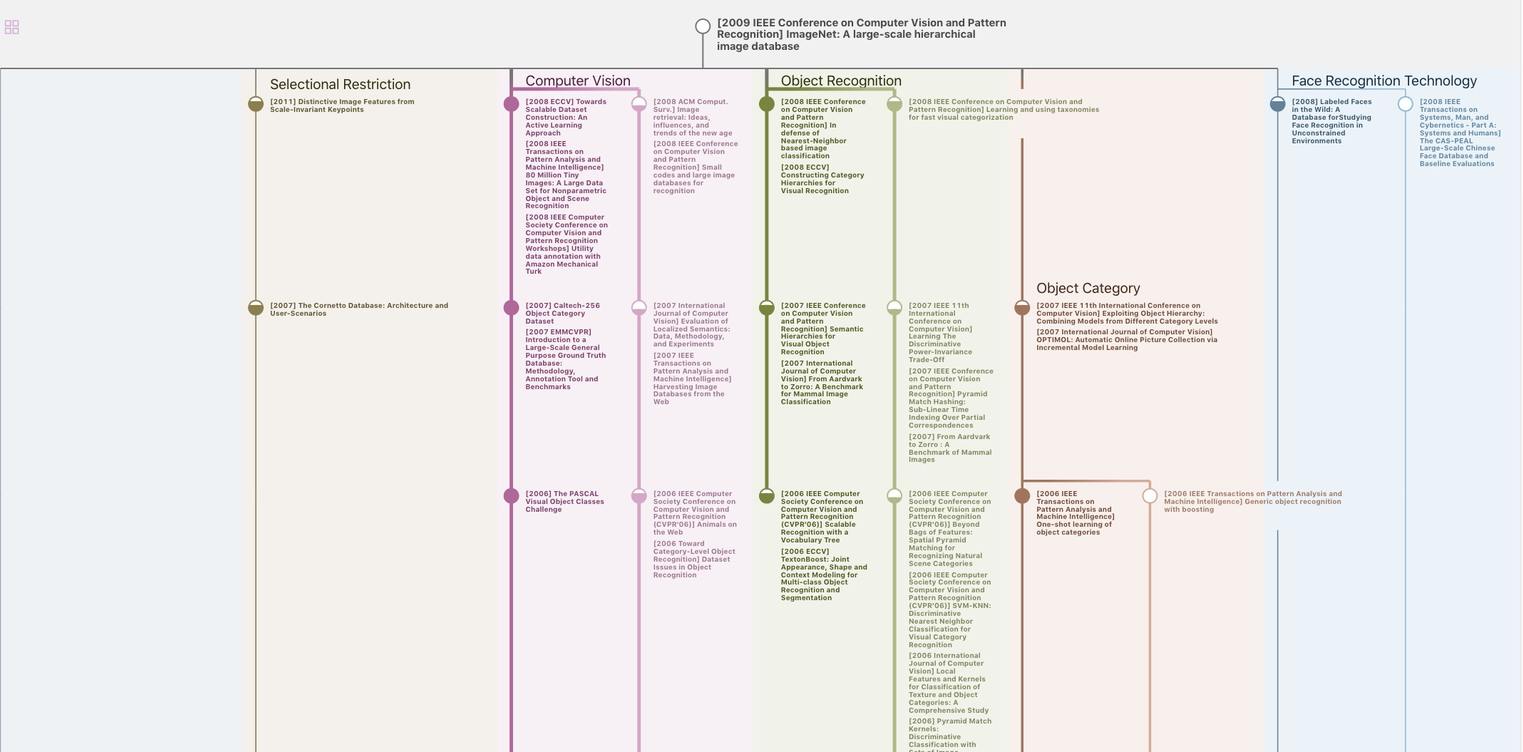
生成溯源树,研究论文发展脉络
Chat Paper
正在生成论文摘要