Self-Training with Label-Feature-Consistency for Domain Adaptation
Database Systems for Advanced Applications(2023)
摘要
Mainstream approaches for unsupervised domain adaptation (UDA) learn domain-invariant representations to address the domain shift. Recently, self-training has been used in UDA, which exploits pseudo-labels for unlabeled target domains. However, the pseudo-labels can be unreliable due to distribution shifts between domains, severely impairing the model performance. To address this problem, we propose a novel self-training framework-Self-Training with Label-Feature-Consistency (ST-LFC), which selects reliable target pseudo-labels via label-level and feature-level voting consistency principle. The former means target pseudo-labels generated by a source-trained classifier and the latter means the nearest source-class to the target in feature space. In addition, ST-LFC reduces the negative effects of unreliable predictions through entropy minimization. Empirical results indicate that ST-LFC significantly improves over the state-of-the-arts on a variety of benchmark datasets.
更多查看译文
关键词
Transfer learning, Domain Adaptation, Self-Training, Label-Feature-Consistency
AI 理解论文
溯源树
样例
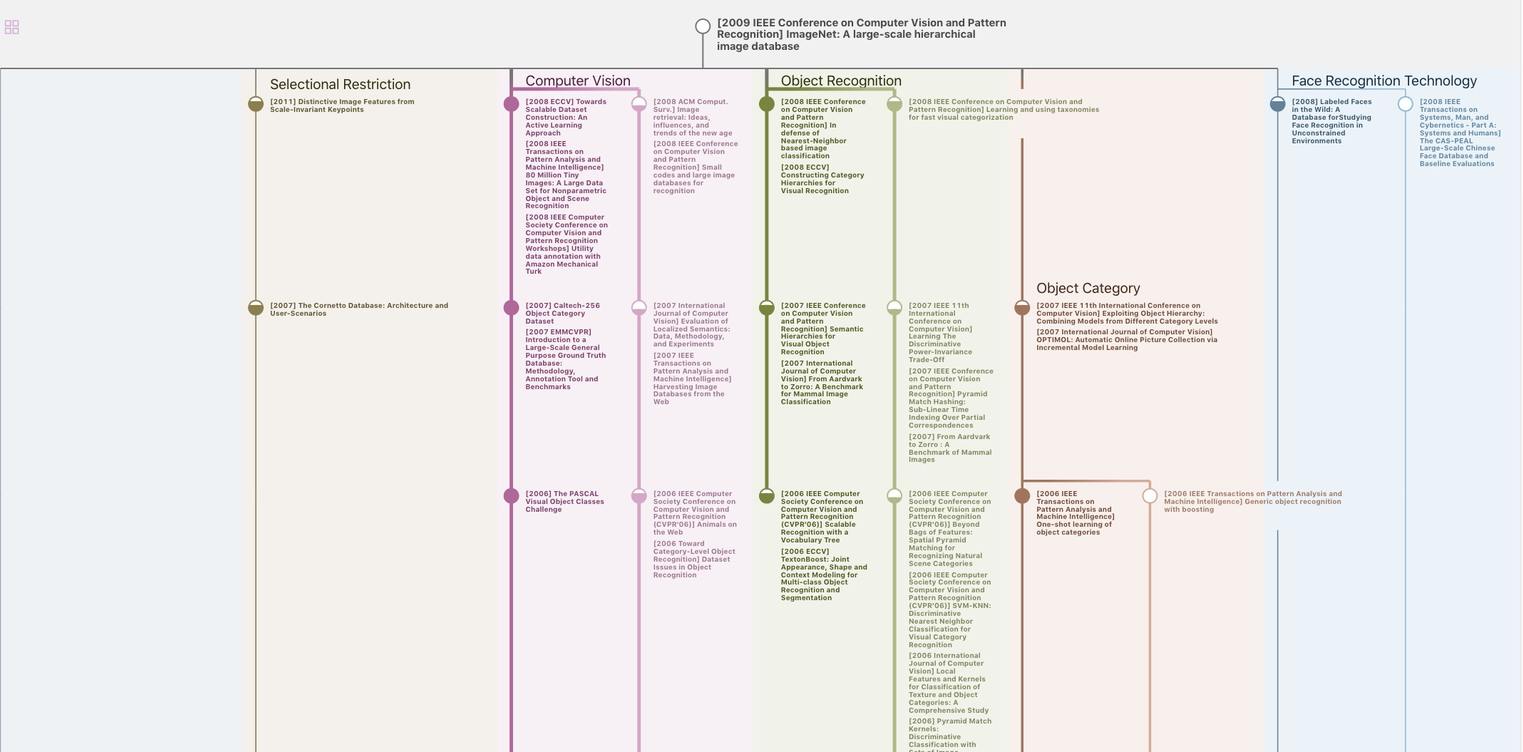
生成溯源树,研究论文发展脉络
Chat Paper
正在生成论文摘要