Explaining Black Box Reinforcement Learning Agents Through Counterfactual Policies
Advances in Intelligent Data Analysis XXI(2023)
摘要
Despite the increased attention to explainable AI, explainability methods for understanding reinforcement learning (RL) agents have not been extensively studied. Failing to understand the agent’s behavior may cause reduced productivity in human-agent collaborations, or mistrust in automated RL systems. RL agents are trained to optimize a long term cumulative reward, and in this work we formulate a novel problem on how to generate explanations on when an agent could have taken another action to optimize an alternative reward. More concretely, we aim at answering the question: What does an RL agent need to do differently to achieve an alternative target outcome? We introduce the concept of a counterfactual policy, as a policy trained to explain in which states a black box agent could have taken an alternative action to achieve another desired outcome. The usefulness of counterfactual policies is demonstrated in two experiments with different use-cases, and the results suggest that our solution can provide interpretable explanations.
更多查看译文
关键词
learning
AI 理解论文
溯源树
样例
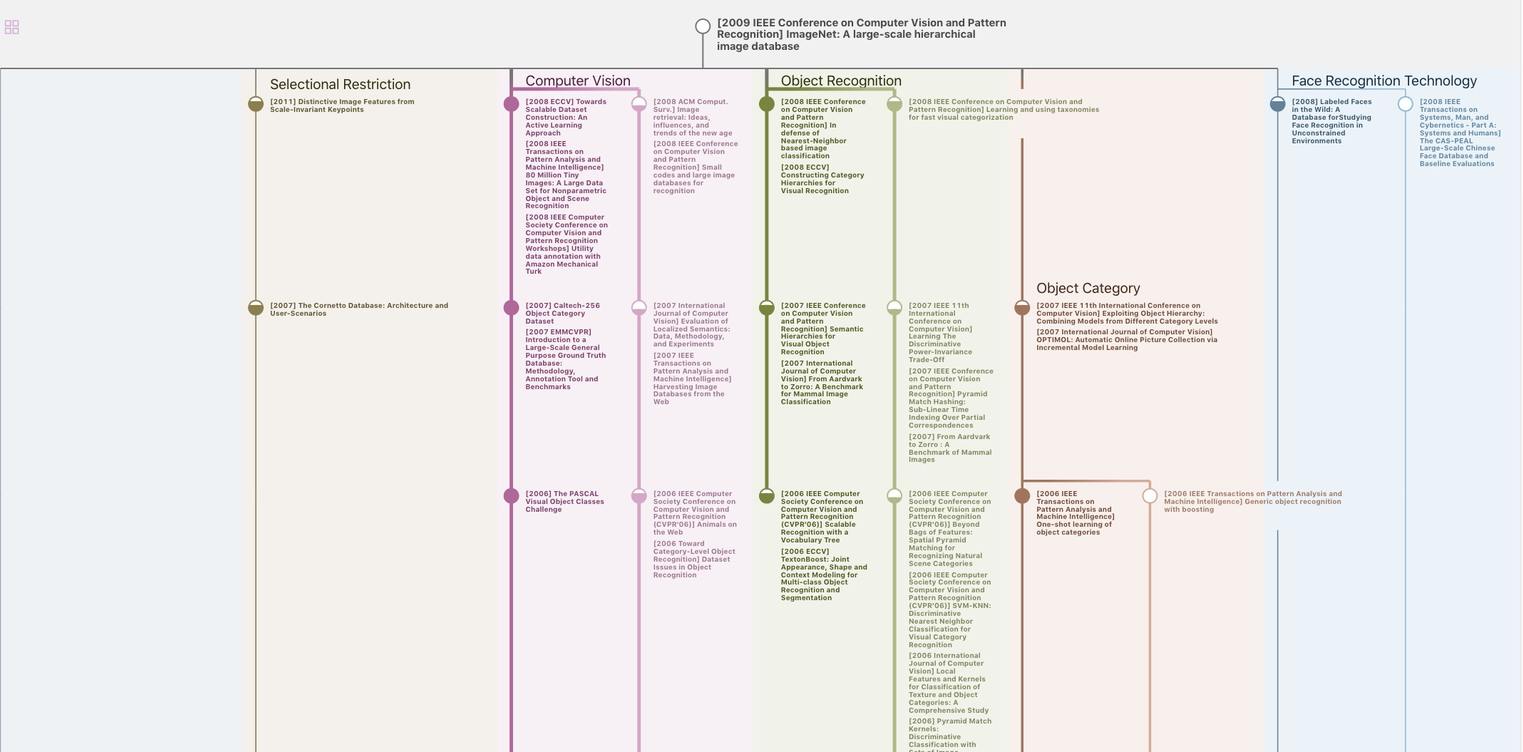
生成溯源树,研究论文发展脉络
Chat Paper
正在生成论文摘要