Graph Laplacian and Neural Networks for Inverse Problems in Imaging: GraphLaNet
Scale Space and Variational Methods in Computer Vision(2023)
摘要
In imaging problems, the graph Laplacian is proven to be a very effective regularization operator when a good approximation of the image to restore is available. In this paper, we study a Tikhonov method that embeds the graph Laplacian operator in a
$$\ell _1$$
–norm penalty term. The novelty is that the graph Laplacian is built upon a first approximation of the solution obtained as the output of a trained neural network. Numerical examples in 2D computerized tomography demonstrate the efficacy of the proposed method.
更多查看译文
关键词
imaging,inverse problems,laplacian,graph,networks
AI 理解论文
溯源树
样例
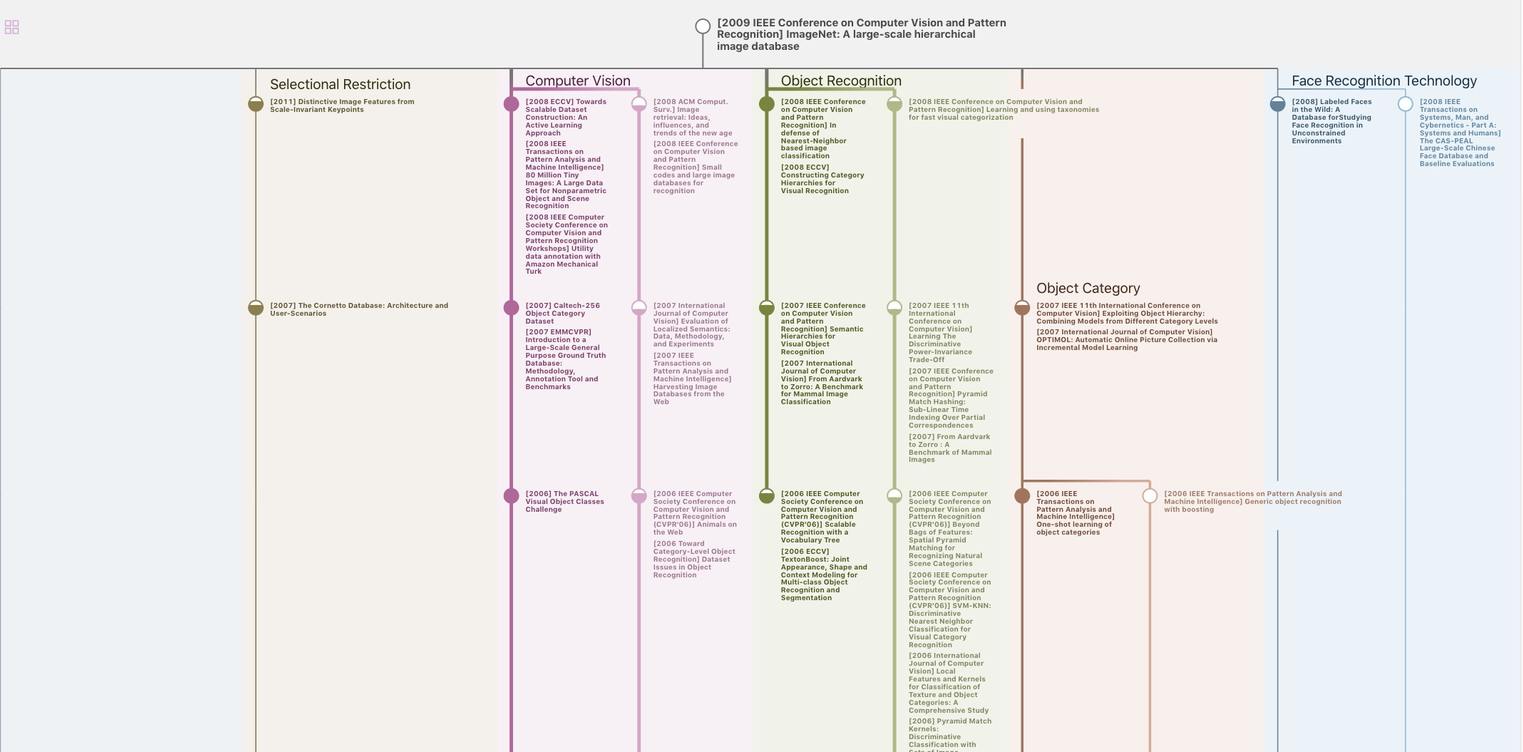
生成溯源树,研究论文发展脉络
Chat Paper
正在生成论文摘要