Faster Robotic Arm Movement Planning via Guided Attenuation Reward Shaping
Advances in Guidance, Navigation and Control(2023)
摘要
The expensive learning cost has become a serious problem in robotic arm movement planning using reinforcement learning method. A significant amount of training is required before convergence, reducing the effectiveness of its application. To address the problems above, we propose a guided attenuation reward shaping (GAR) method that reshapes the reward of the robotic arm movement planning environment. It gradually attenuates from a slightly dense distance reward to a sparse binary reward to speed up the Actor-Critic based reinforcement learning algorithm’s convergence speed, allowing the robot arm to learn the optimal path to reach the target swiftly. We verify the method in the built robotic arm operating environment and conduct comparative experiments. The result shows that our proposed GAR method significantly increases the convergence speed and obtains the shortest path to the target.
更多查看译文
关键词
Reward shaping, Reinforcement learning, Movement planning
AI 理解论文
溯源树
样例
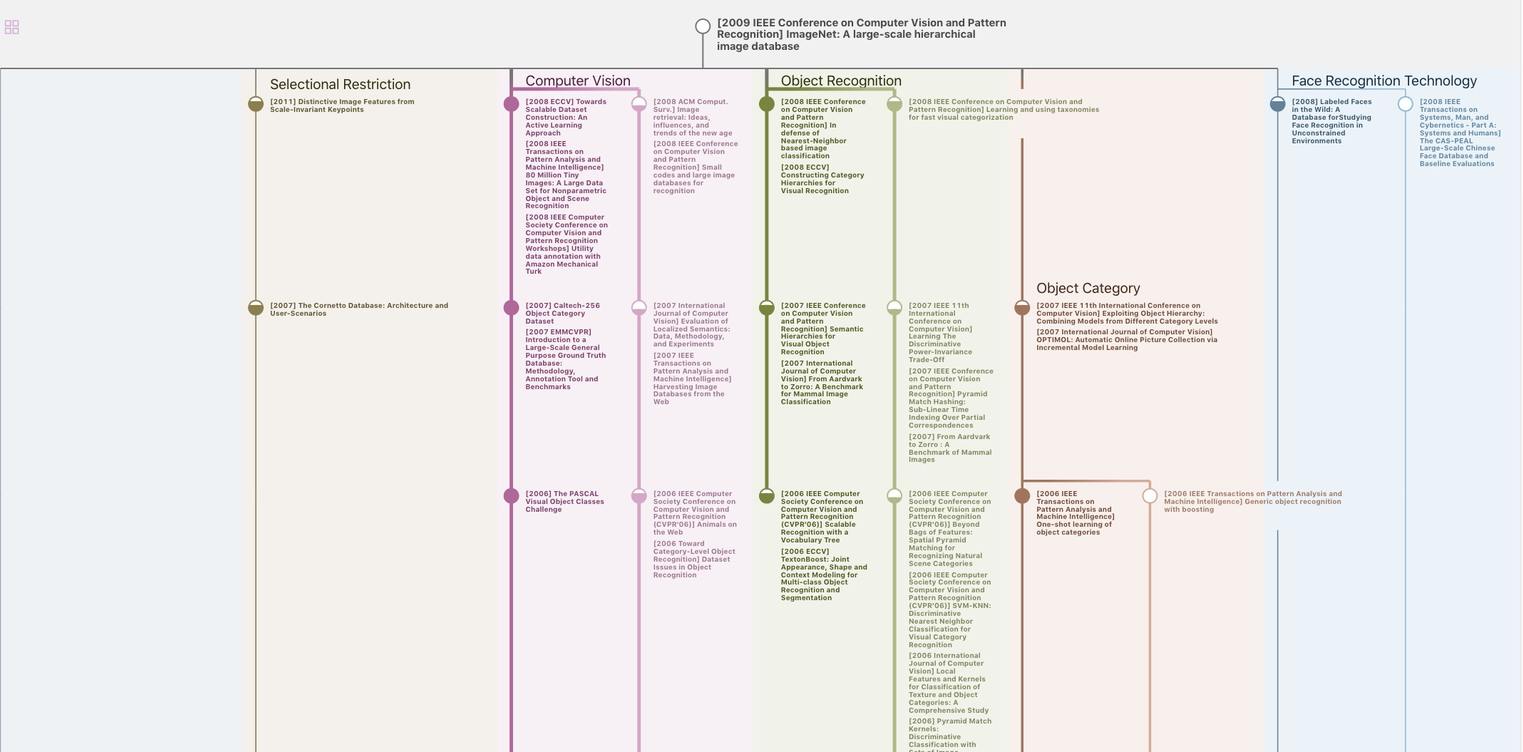
生成溯源树,研究论文发展脉络
Chat Paper
正在生成论文摘要