A Biased Random Walk Scale-Free Network Growth Model with Tunable Clustering
Complex Networks and Their Applications XI(2023)
摘要
Complex networks appear naturally in many real-world situations. A power law is generally a good fit for their degree distribution. The popular Barabasi-Albert model (BA) combines growth and preferential attachment to model the emergence of the power law. One builds a network by adding new nodes that preferentially link to high-degree nodes in the network. One can also exploit random walks. In this case, the network growth is determined by choosing parent vertices by sequential random walks. The BA model’s main drawback is that the sample networks’ clustering coefficient is low, while typical real-world networks exhibit a high clustering coefficient. Indeed, nodes tend to form highly connected groups in real-world networks, particularly social networks. In this paper, we introduce a Biased Random Walk model with two parameters allowing us to tune the degree distribution exponent and the clustering coefficient of the sample networks. This efficient algorithm relies on local information to generate more realistic networks reproducing known real-world network properties.
更多查看译文
关键词
clustering,growth,network,scale-free
AI 理解论文
溯源树
样例
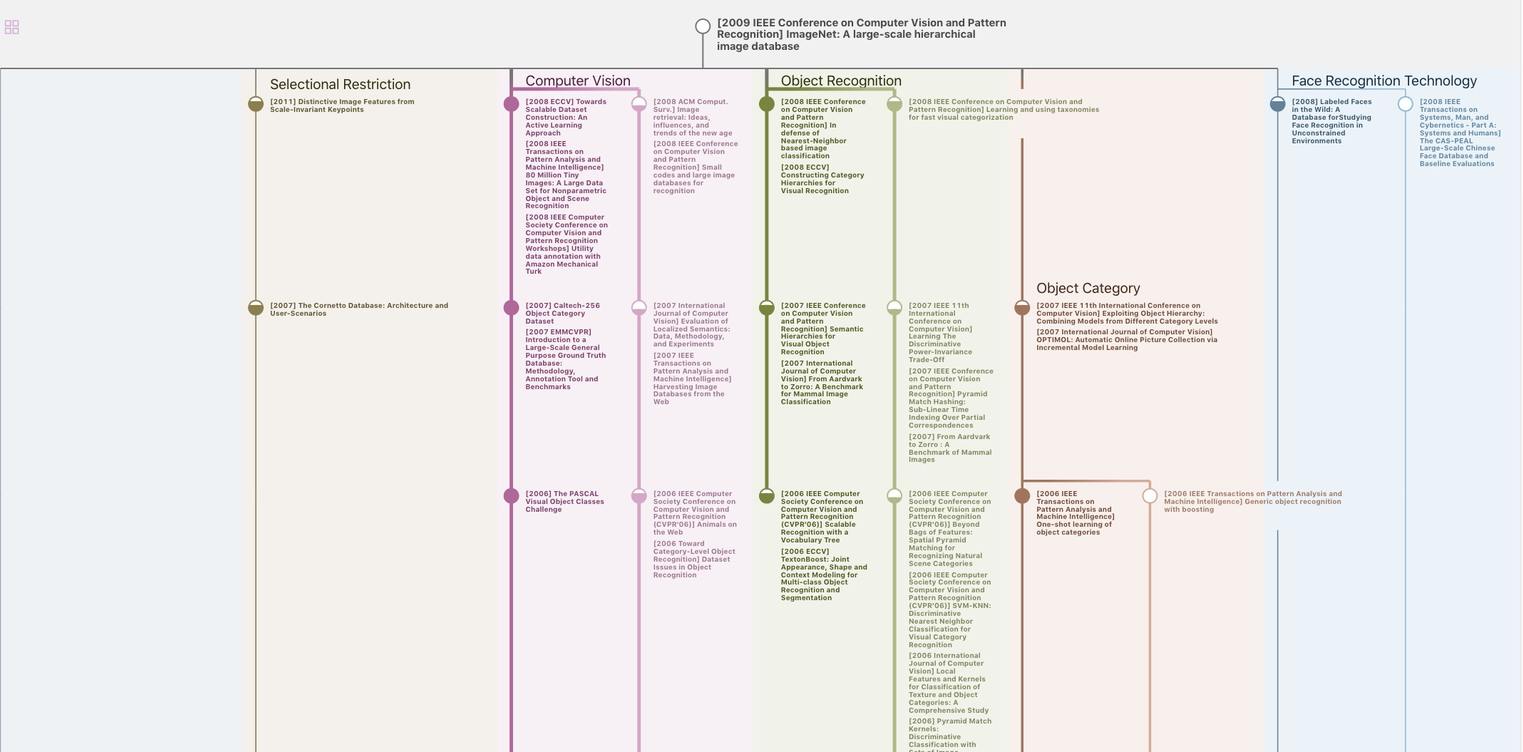
生成溯源树,研究论文发展脉络
Chat Paper
正在生成论文摘要