Application of Reinforcement Learning for the Design and Optimization of Pass Schedules in Hot Rolling
Production at the Leading Edge of Technology(2023)
摘要
About 95% of all steel products are rolled at least once during their production. Thus, any further improvement of the already highly optimized rolling process, for example reduction of energy consumption, has a significant impact. Currently, most rolling processes are designed by experts based on their knowledge and heuristics using fast analytical rolling models (FRM). However, due to the complex interactions between the processing constraints e.g. machine limits, the process parameters as well as the product properties, these manual process designs often focus on a single optimization objective. Here, novel methods such as reinforcement learning (RL) can detect complex correlations between chosen parameters and achieved objectives by interacting with an environment i.e. FRM. Therefore, this contribution demonstrates the potential of coupling RL and a FRM for the design and multiple objective optimization of rolling processes. Using FRM data e.g. the microstructure evolution, the coupled approach learns to map the current state, such as the height, to process parameters in order to maximize a numerical value and thereby optimize the process. For this, an objective function is presented that satisfies all (technical) constraints, leads to desired material properties including microstructural aspects and reduces the energy consumption. Here, two RL algorithms, DQN and DDPG, are used to design and optimize pass schedules for two use cases (different starting and final heights). The resulting pass schedules achieve the desired goals, for example, the desired grain size is achieved within 4 µm on average. These meaningful solutions can prospectively enable further improvements.
更多查看译文
关键词
Hot Rolling, Process Design, Reinforcement Learning
AI 理解论文
溯源树
样例
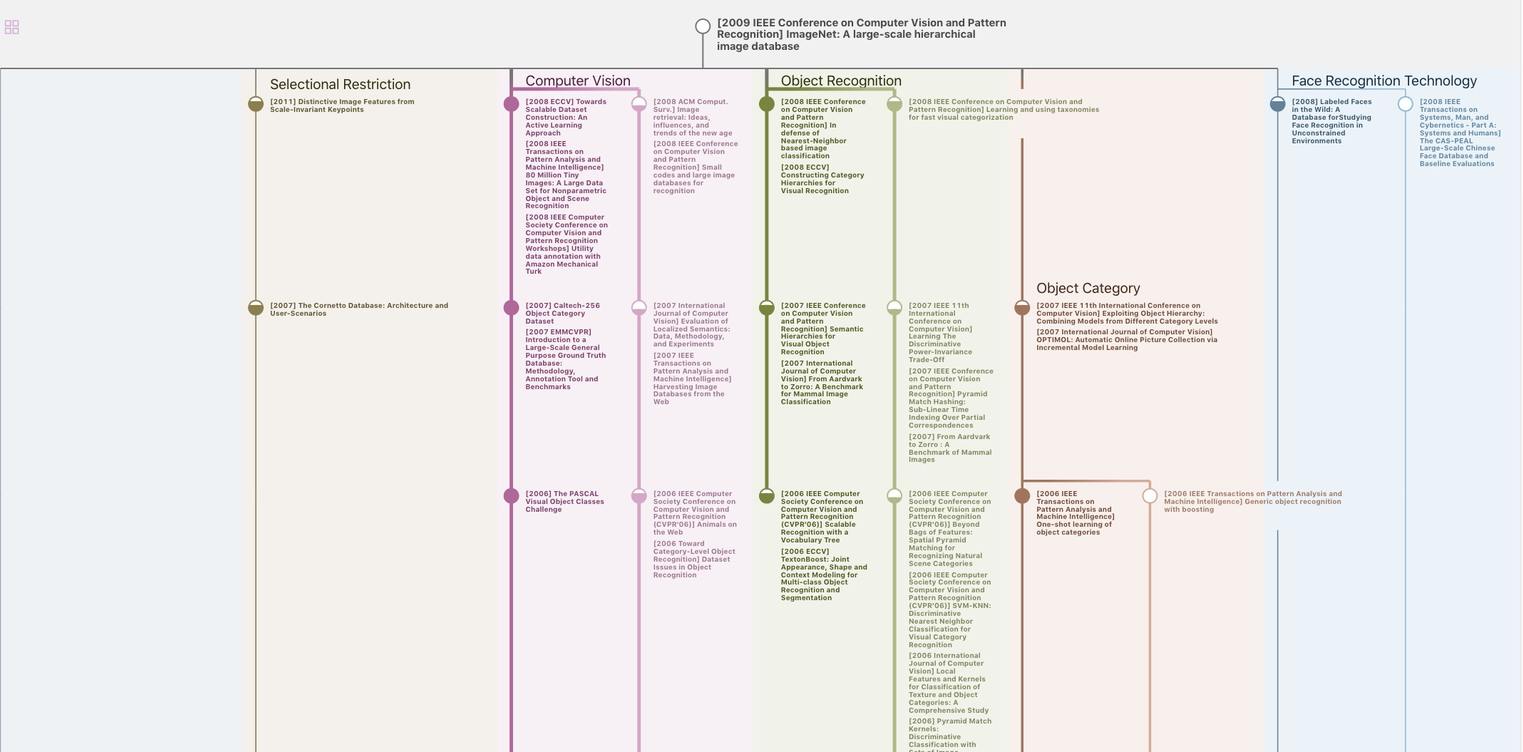
生成溯源树,研究论文发展脉络
Chat Paper
正在生成论文摘要