Finding Best Probabilities in Human Resources Satisfaction by Using Structural Equation Modeling, Bayesian Networks, and Decision Trees
Operational Research in the Era of Digital Transformation and Business Analytics(2023)
摘要
Every company searches, implements, and invests in new, more effective, and more efficient ways to recruit new personnel. They use any methods available, like conducting consecutive interviews, using different kinds of testing environments and programs, searching thoroughly the candidates’ practical and theoretical knowledge, testing hard, soft, and digital skills, etc. This paper investigates the ways that a company should select human resources by using Machine Learning methods. To do this, Structural Equation Modeling, following by the Bayesian Networks, are firstly used. To cross validate the results and paths of the previous methods, the Decision Trees method is used, which is a Machine Learning algorithm that analyzes the data concerning human resources selection and produces a graphic tree about key point decisions that should be made, depending on some specific values and bounds of different kinds of metrics, which actually help the company to decrease its expenses and increase its performance. The main target of this work is to identify the factors that affect the job satisfaction of the personnel. Factors, such as the likelihood of successful training with the minimal cost and in the shortest time period, as well as the possible work performance development, are important issues in this research. It is considered that this work will have a strong impact on how candidate selection should be done. To the best of our knowledge, there has not been, so far, a work to relate Machine Learning with human resources selection.
更多查看译文
关键词
Machine learning, Human resources, Decision trees, Structural equation modeling, Bayesian networks
AI 理解论文
溯源树
样例
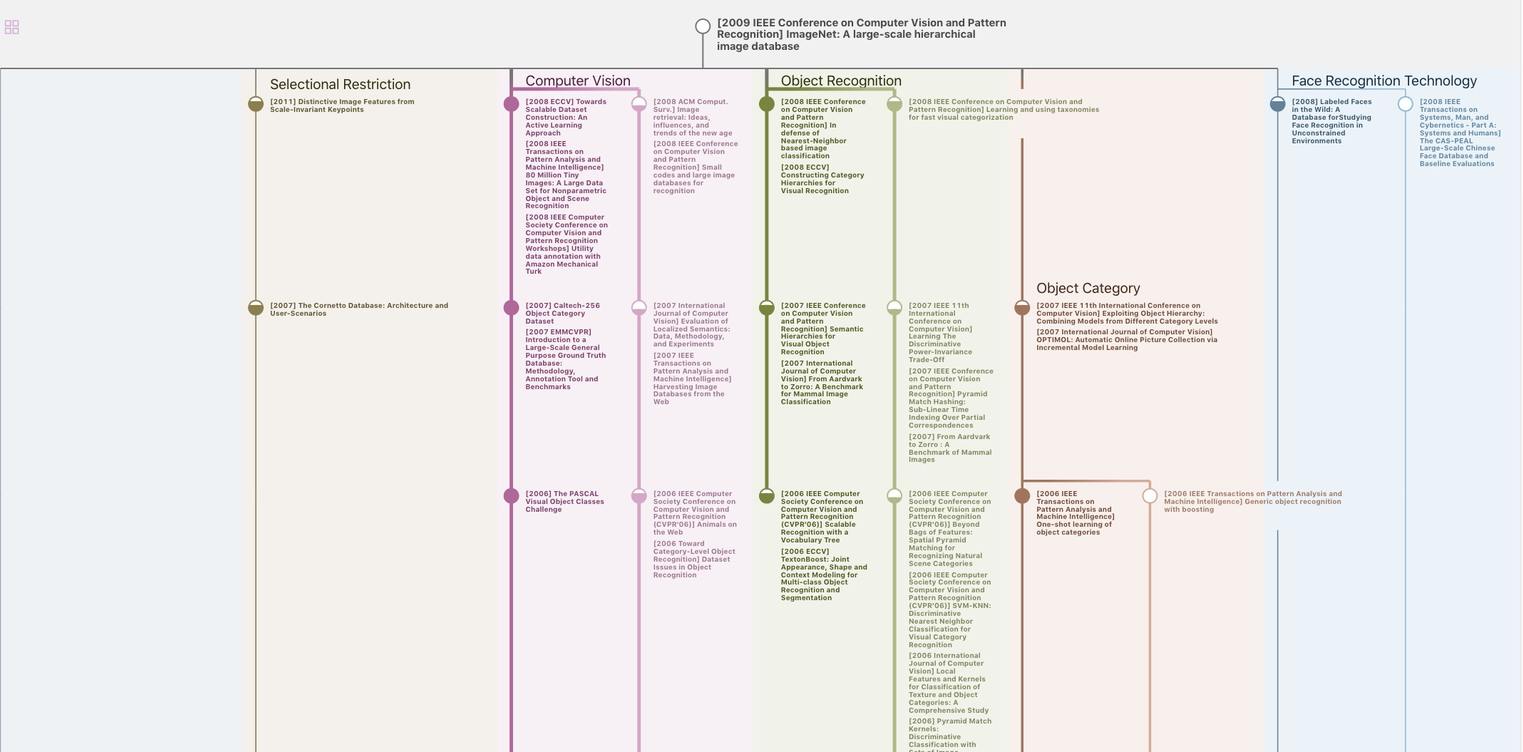
生成溯源树,研究论文发展脉络
Chat Paper
正在生成论文摘要