IGFClust: Clustering Unbalanced and Complex Single-Cell Expression Data by Iteration and Integrating Gini Index and Fano Factor
Computer Science and Education(2023)
摘要
With ScRNAseq, we are able to obtain genome-wide transcriptome data from single cells. However, it is very difficult to identify all cell subpopulations in single cell expression data, especially when these subpopulations are unbalanced and the number of subpopulations is unknown. In this paper, we propose a new clustering algorithm, IGFClust. We design an ensemble method to identify unbalanced subpopulations using Gini index and Fano factor. In addition, we design an iterative clustering framework to avoid the problem that only some subpopulations can be identified during the clustering process. We generated four sets of labeled simulation data and compared IGFClust with existing methods. Afterwards, we analyzed 576 glioblastoma primary tumor cells. We show that IGFClust performs accurately and robustly in identifying complex and unbalanced single-cell expression data.
更多查看译文
关键词
integrating gini index,fano factor,single-cell
AI 理解论文
溯源树
样例
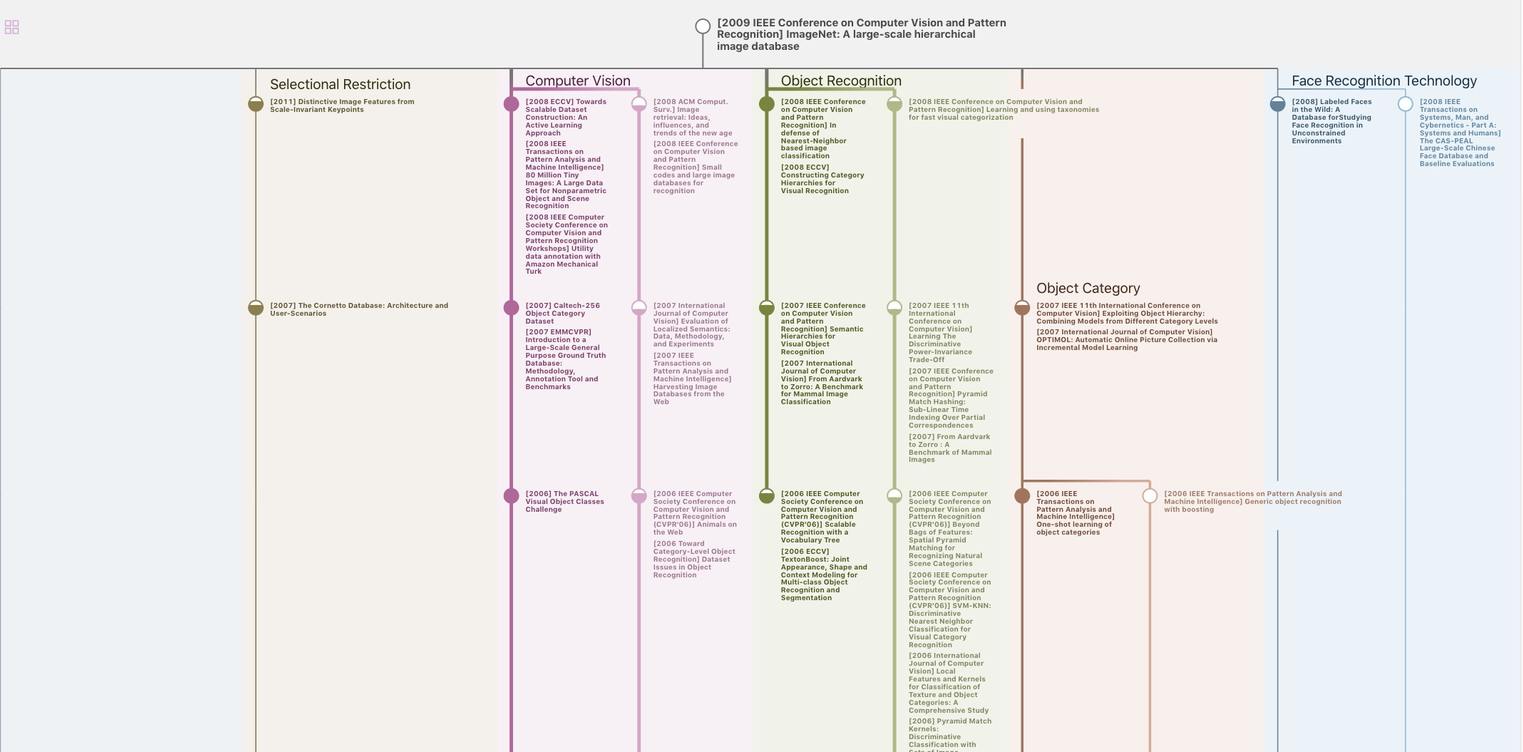
生成溯源树,研究论文发展脉络
Chat Paper
正在生成论文摘要