Unsupervised Handwritten Signature Verification with Extreme Learning Machines
Proceedings of ELM 2021(2023)
摘要
Handwritten signature verification has two approaches based on online or offline data collection, both of them being supervised machine learning tasks. This work investigates the feasibility of unsupervised signature verification. It is inspired by a model-based forged signature generation approach, whose inversion could potentially provide an unsupervised solution for the signature verification task. The model inversion is attempted on a massive collection of image patches taken from samples of a large GPDSS10000 artificial signature verification dataset, pre-processed by a general-purpose deep learning network that extracts 1024 meaningful image features. An Extreme Learning Machine (ELM) solves the inversion problem at a very large scale. The paper proposes practical ways of ELM model structure selection on massive datasets and faster solvers. The results show the feasibility of an unsupervised solution for signature verification.
更多查看译文
关键词
extreme learning machines,verification
AI 理解论文
溯源树
样例
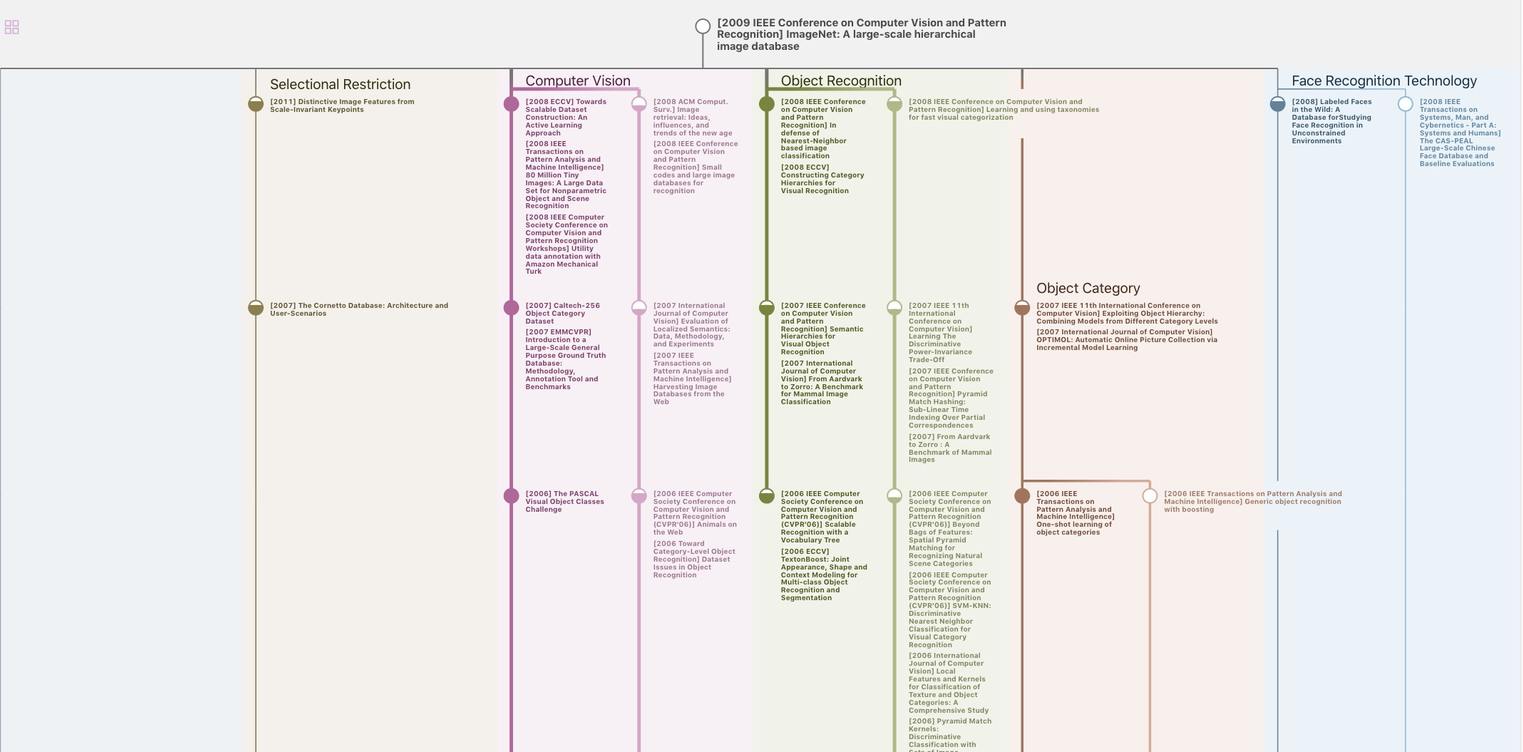
生成溯源树,研究论文发展脉络
Chat Paper
正在生成论文摘要