Predicting Where You Visit in a Surrounding City: A Mobility Knowledge Transfer Framework Based on Cross-City Travelers
Database Systems for Advanced Applications(2023)
摘要
The increasingly built intercity transportation enables people to visit surrounding cities conveniently. Hence it is becoming a hot research topic to predict where a traveler would visit in a surrounding city based on check-in data collected from location-based mobile Apps. However, as most users rarely travel out of hometown, there is a high skew of the quantity of check-in data between hometown and surrounding cities. Suffering from the severe sparsity of user mobility data in surrounding city, existing approaches do not perform well as they can hardly maintain travelers’ intrinsic preference and meanwhile adapt to travelers’ interest drift. To address these concerns, in this paper, taking cross-city travelers as the medium, we propose a novel framework called CityTrans to transfer traveler mobility knowledge from hometown city to surrounding city, which considers both the long-term preference in hometown city and short-term interest drift in surrounding city. Various attention mechanisms are leveraged to obtain traveler representation enriched by long-term and short-term preferences. Besides, we propose to portray POIs through GNN incorporating POI attributes and geographical information. Finally, the traveler and POI representations are combined for prediction. To train the framework, the transfer loss as well as the prediction loss are jointly optimized. Extensive experiments on real-world datasets validate the superiority of our framework over several state-of-the-art approaches.
更多查看译文
关键词
mobility knowledge transfer framework,surrounding city,cross-city
AI 理解论文
溯源树
样例
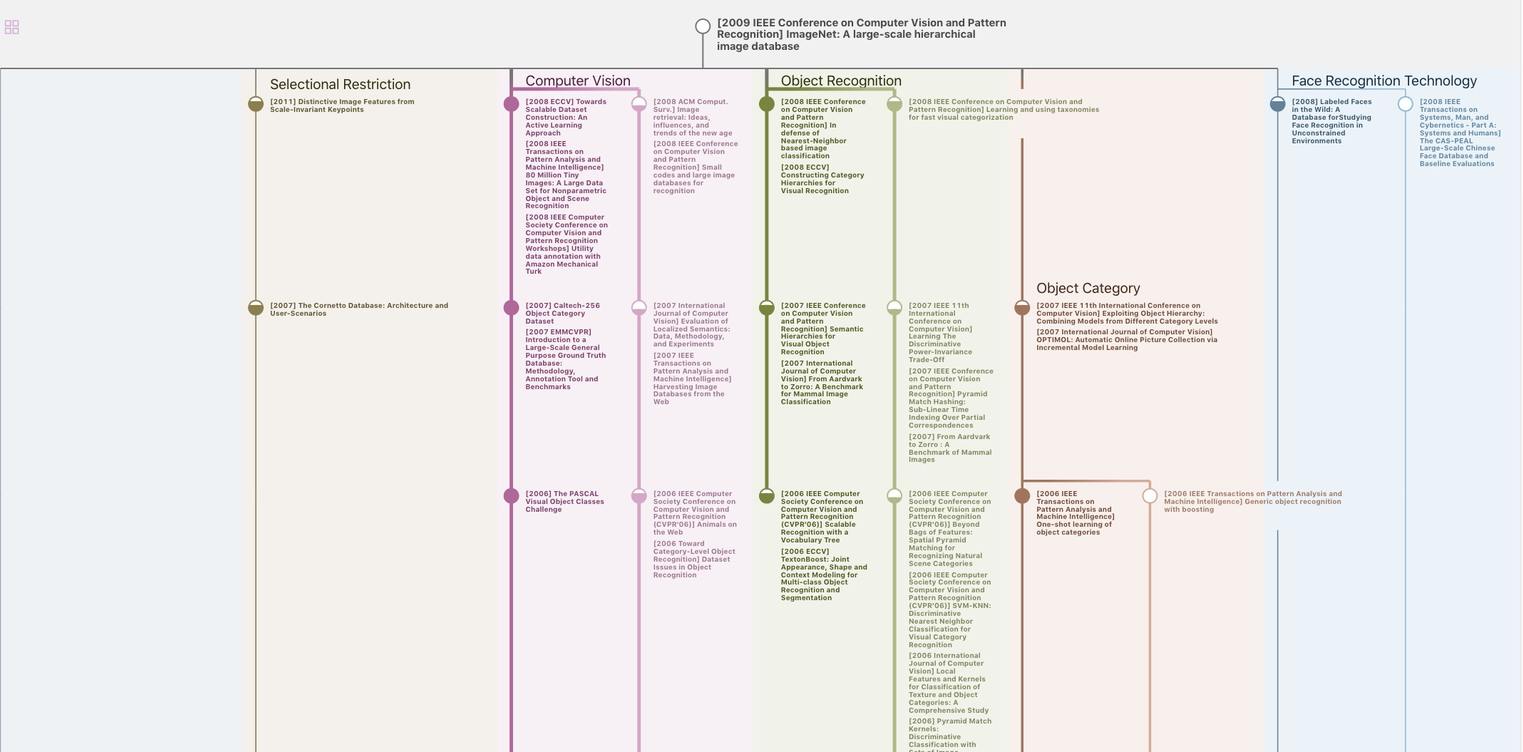
生成溯源树,研究论文发展脉络
Chat Paper
正在生成论文摘要