Retinal OCT Image Classification Based on CNN and Transfer Learning
Proceedings of the 14th International Conference on Soft Computing and Pattern Recognition (SoCPaR 2022)(2023)
摘要
Untreated retinal diseases may result in severe vision loss or even blindness. However, with early diagnosis, some retinal diseases can be treated, while others can be controlled. Nowadays, images obtained using optical coherence tomography (OCT) are used to diagnose retinal diseases, and these are interpreted by ophthalmologists, which is time-consuming. Moreover, there is a shortage of ophthalmologists worldwide. The study aims to classify three types of retinal diseases (diabetic macular edema, drusen, and choroidal neovascularization) and normal eye OCT images. For this reason, a CNN model was designed, and two transfer learning models (ResNet152V2 and DenseNet169) were fine-tuned and finally compared to determine the best model for accurately classifying diseases. These models were assessed using the Mendeley OCT dataset. The test accuracies obtained by the CNN, ResNet152V2, and DenseNet169 are respectively 98.34%, 99.17%, and 99.38%. The proposed approaches outperformed many well-known OCT classification methods, according to the results. Therefore, these models may have a potential effect on retinal disease diagnosis using OCT images.
更多查看译文
关键词
Transfer learning, Retina, Image classification, Optical coherence tomography
AI 理解论文
溯源树
样例
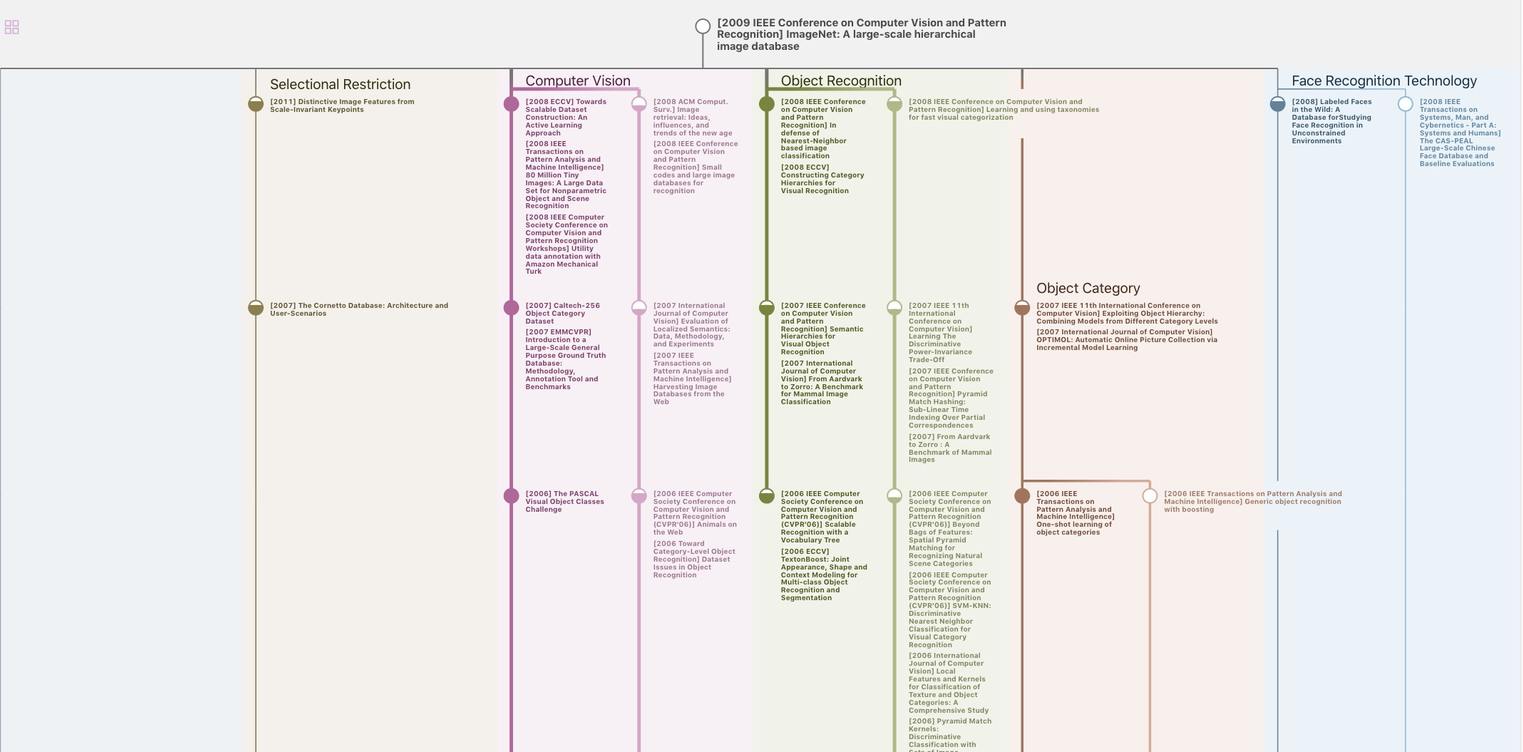
生成溯源树,研究论文发展脉络
Chat Paper
正在生成论文摘要