CDGCN: Conditional de novo Drug Generative Model Using Graph Convolution Networks
Research in Computational Molecular Biology(2023)
摘要
De novo drug design is a crucial part of drug discovery which is a highly expensive and slow process. Many deep learning methods have been proposed to automate and accelerate it. However, most of the current state-of-the-art methods are limited to generating novel drugs specific to proteins that already have known drugs or limited to generating molecules which lack certain desirable drug-like properties like high binding affinity or low binding energy. We introduce our graph generative model, CDGCN (Conditional de novo drug generative model using Graph Convolution Networks), for de novo drug generation for novel proteins, which takes as input a protein sequence of amino acids and generates novel molecular structures having desirable drug-like properties. CDGCN generates desirable molecules for a protein using a sequential decoding scheme by learning the distribution of generation paths of its ligands. We show that CDGCN can quickly generate novel and chemically valid drug-like molecules which have a higher binding affinity with their target proteins as compared to the state-of-the-art methods. The best binding energy between a novel protein and its novel drug-like molecules generated by CDGCN was observed to be at least −7.3 kcal/mol whereas for the state-of-the-art method it was observed to be −6.2 kcal/mol. Availability and implementation: Code and data are available at
https://github.com/mshik/CDGCN
.
更多查看译文
关键词
Graph convolution network, De novo drug design, Graph generation
AI 理解论文
溯源树
样例
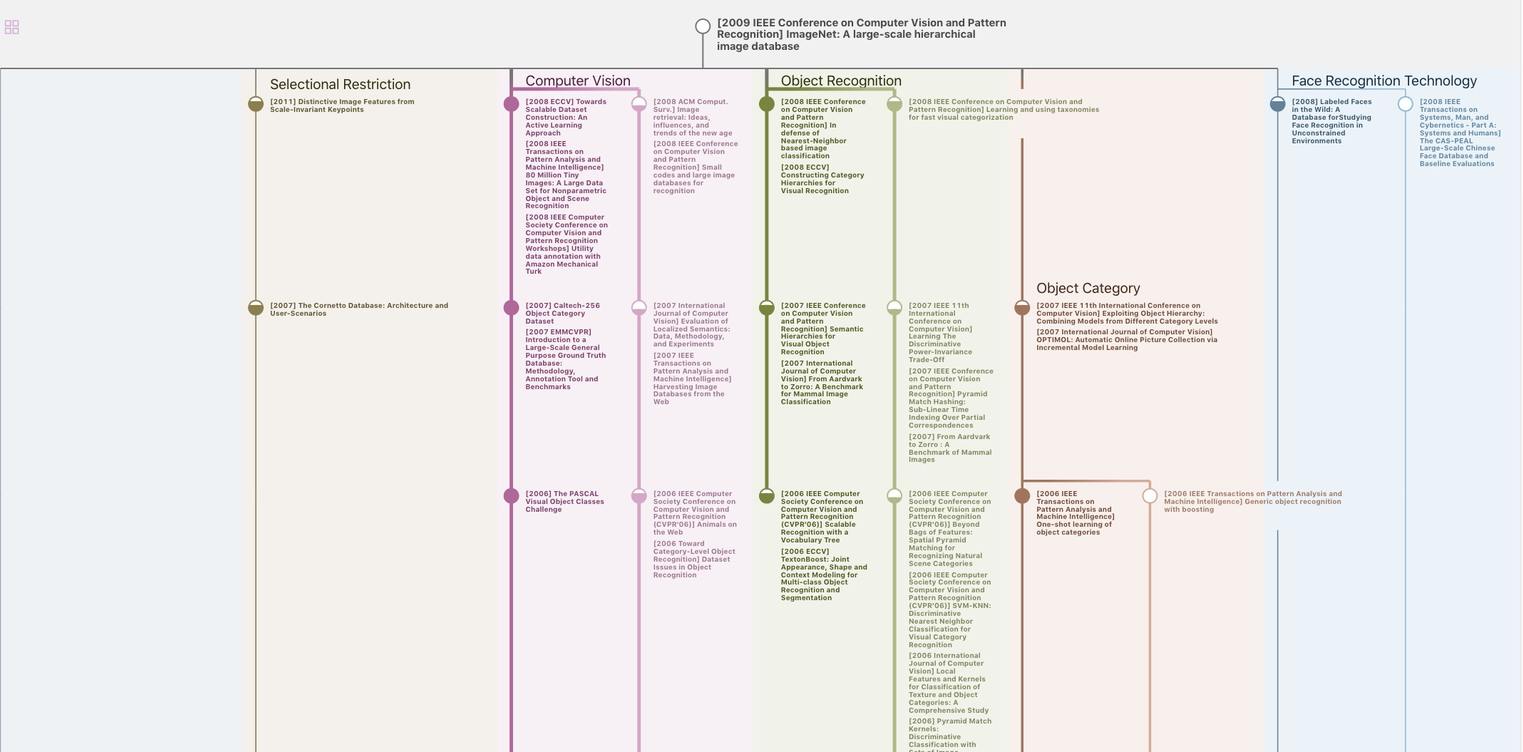
生成溯源树,研究论文发展脉络
Chat Paper
正在生成论文摘要