Discovery of interconnected causal drivers of COVID-19 vaccination intentions in the US using a causal Bayesian network
Scientific Reports(2023)
摘要
Holistic interventions to overcome COVID-19 vaccine hesitancy require a system-level understanding of the interconnected causes and mechanisms that give rise to it. However, conventional correlative analyses do not easily provide such nuanced insights. We used an unsupervised, hypothesis-free causal discovery algorithm to learn the interconnected causal pathways to vaccine intention as a causal Bayesian network (BN), using data from a COVID-19 vaccine hesitancy survey in the US in early 2021. We identified social responsibility, vaccine safety and anticipated regret as prime candidates for interventions and revealed a complex network of variables that mediate their influences. Social responsibility’s causal effect greatly exceeded that of other variables. The BN revealed that the causal impact of political affiliations was weak compared with more direct causal factors. This approach provides clearer targets for intervention than regression, suggesting it can be an effective way to explore multiple causal pathways of complex behavioural problems to inform interventions.
更多查看译文
关键词
interconnected causal drivers,vaccination,intentions,discovery
AI 理解论文
溯源树
样例
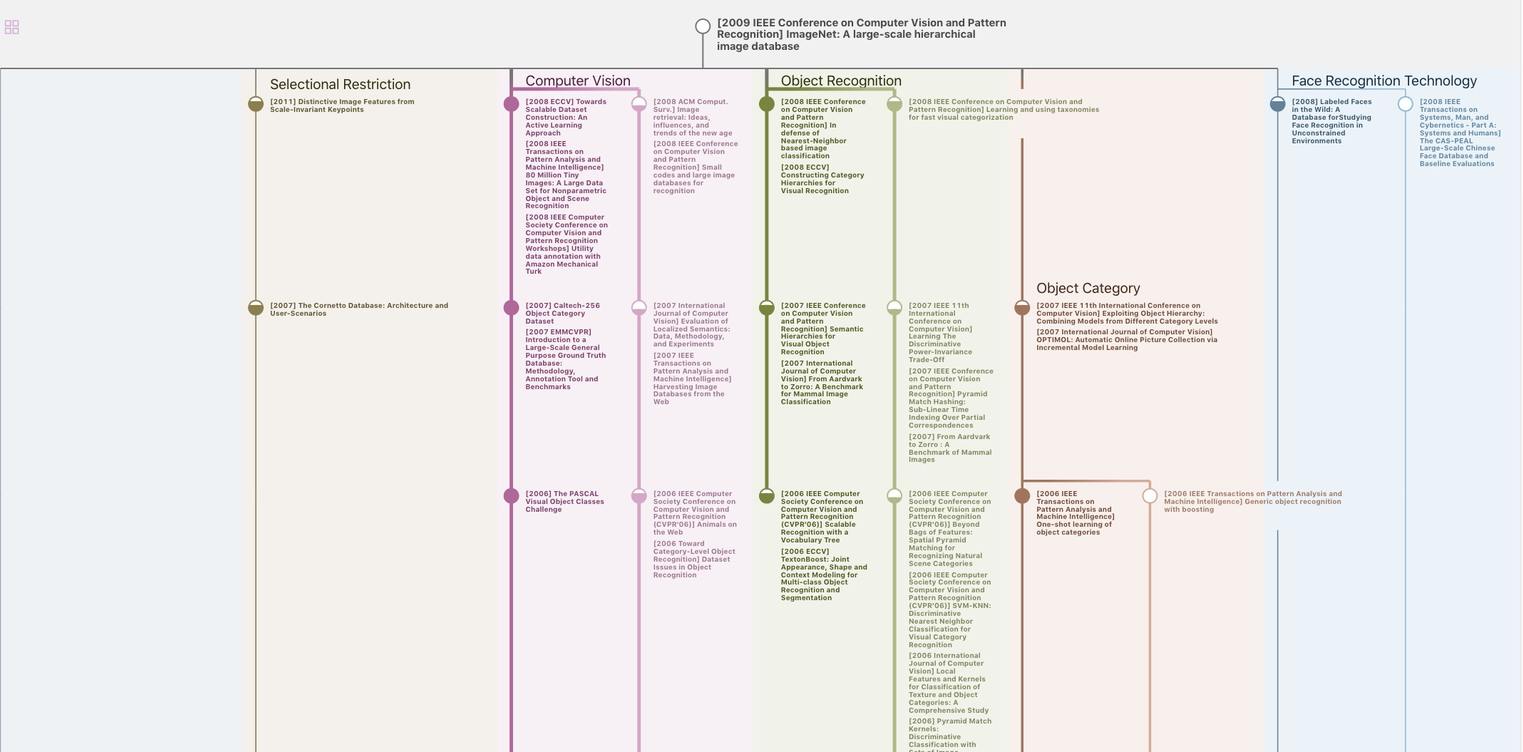
生成溯源树,研究论文发展脉络
Chat Paper
正在生成论文摘要