XAI for Self-supervised Clustering of Wireless Spectrum Activity
CoRR(2023)
摘要
The so-called black-box deep learning (DL) models are increasingly used in classification tasks across many scientific disciplines, including wireless communications domain. In this trend, supervised DL models appear as most commonly proposed solutions to domain-related classification problems. Although they are proven to have unmatched performance, the necessity for large labeled training data and their intractable reasoning, as two major drawbacks, are constraining their usage. The self-supervised architectures emerged as a promising solution that reduces the size of the needed labeled data, but the explainability problem remains. In this paper, we propose a methodology for explaining deep clustering, self-supervised learning architectures comprised of a representation learning part based on a Convolutional Neural Network (CNN) and a clustering part. For the state of the art representation learning part, our methodology employs Guided Backpropagation to interpret the regions of interest of the input data. For the clustering part, the methodology relies on Shallow Trees to explain the clustering result using optimized depth decision tree. Finally, a data-specific visualizations part enables connection for each of the clusters to the input data trough the relevant features. We explain on a use case of wireless spectrum activity clustering how the CNN-based, deep clustering architecture reasons.
更多查看译文
关键词
XAI,explainable AI,self-supervised learning,spectrum sensing,transmission classification
AI 理解论文
溯源树
样例
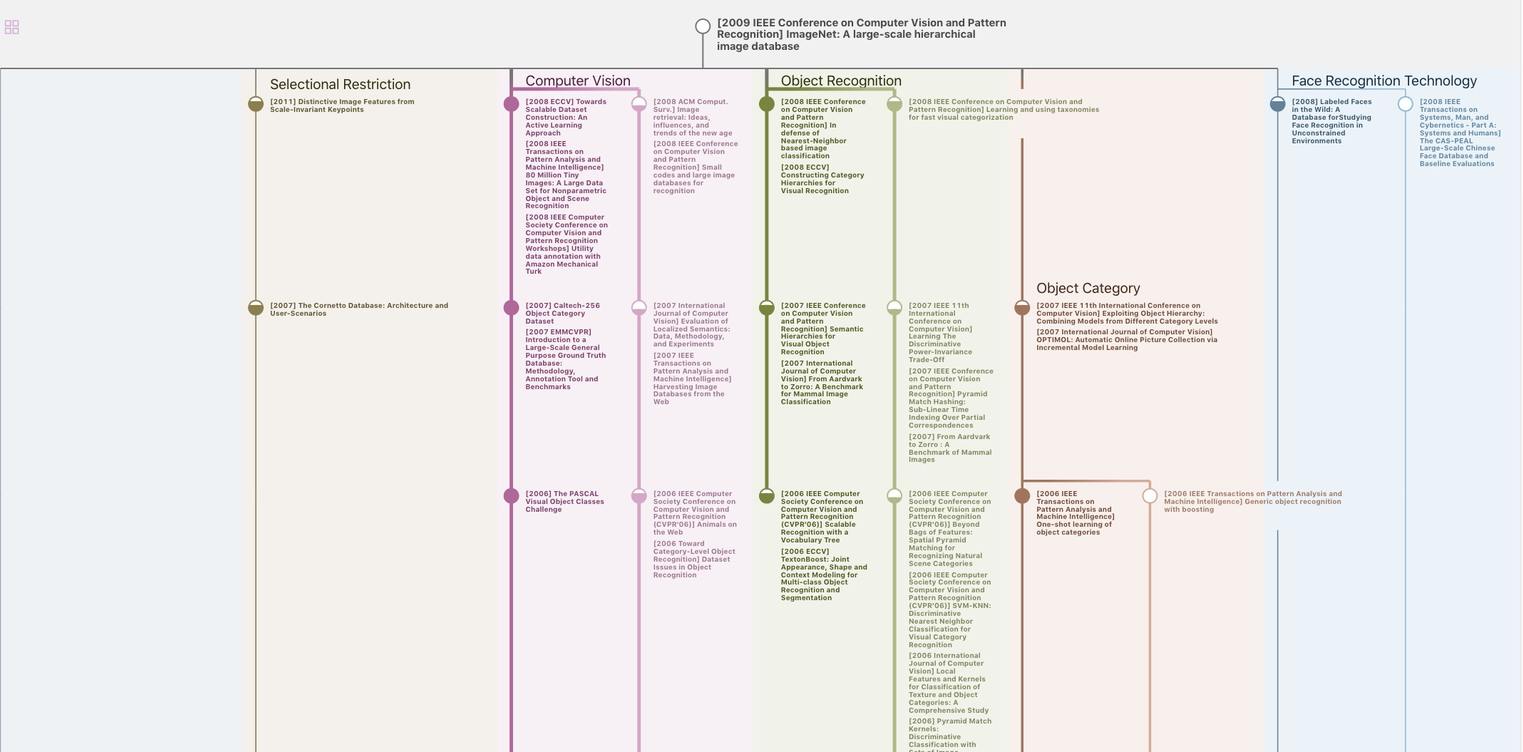
生成溯源树,研究论文发展脉络
Chat Paper
正在生成论文摘要