Distilling Semantic Concept Embeddings from Contrastively Fine-Tuned Language Models
PROCEEDINGS OF THE 46TH INTERNATIONAL ACM SIGIR CONFERENCE ON RESEARCH AND DEVELOPMENT IN INFORMATION RETRIEVAL, SIGIR 2023(2023)
摘要
Learning vectors that capture the meaning of concepts remains a fundamental challenge. Somewhat surprisingly, perhaps, pre-trained language models have thus far only enabled modest improvements to the quality of such concept embeddings. Current strategies for using language models typically represent a concept by averaging the contextualised representations of its mentions in some corpus. This is potentially sub-optimal for at least two reasons. First, contextualised word vectors have an unusual geometry, which hampers downstream tasks. Second, concept embeddings should capture the semantic properties of concepts, whereas contextualised word vectors are also affected by other factors. To address these issues, we propose two contrastive learning strategies, based on the view that whenever two sentences reveal similar properties, the corresponding contextualised vectors should also be similar. One strategy is fully unsupervised, estimating the properties which are expressed in a sentence from the neighbourhood structure of the contextualised word embeddings. The second strategy instead relies on a distant supervision signal from ConceptNet. Our experimental results show that the resulting vectors substantially outperform existing concept embeddings in predicting the semantic properties of concepts, with the ConceptNet-based strategy achieving the best results. These findings are furthermore confirmed in a clustering task and in the downstream task of ontology completion.
更多查看译文
关键词
word embedding,language models,contrastive learning,common-sense knowledge
AI 理解论文
溯源树
样例
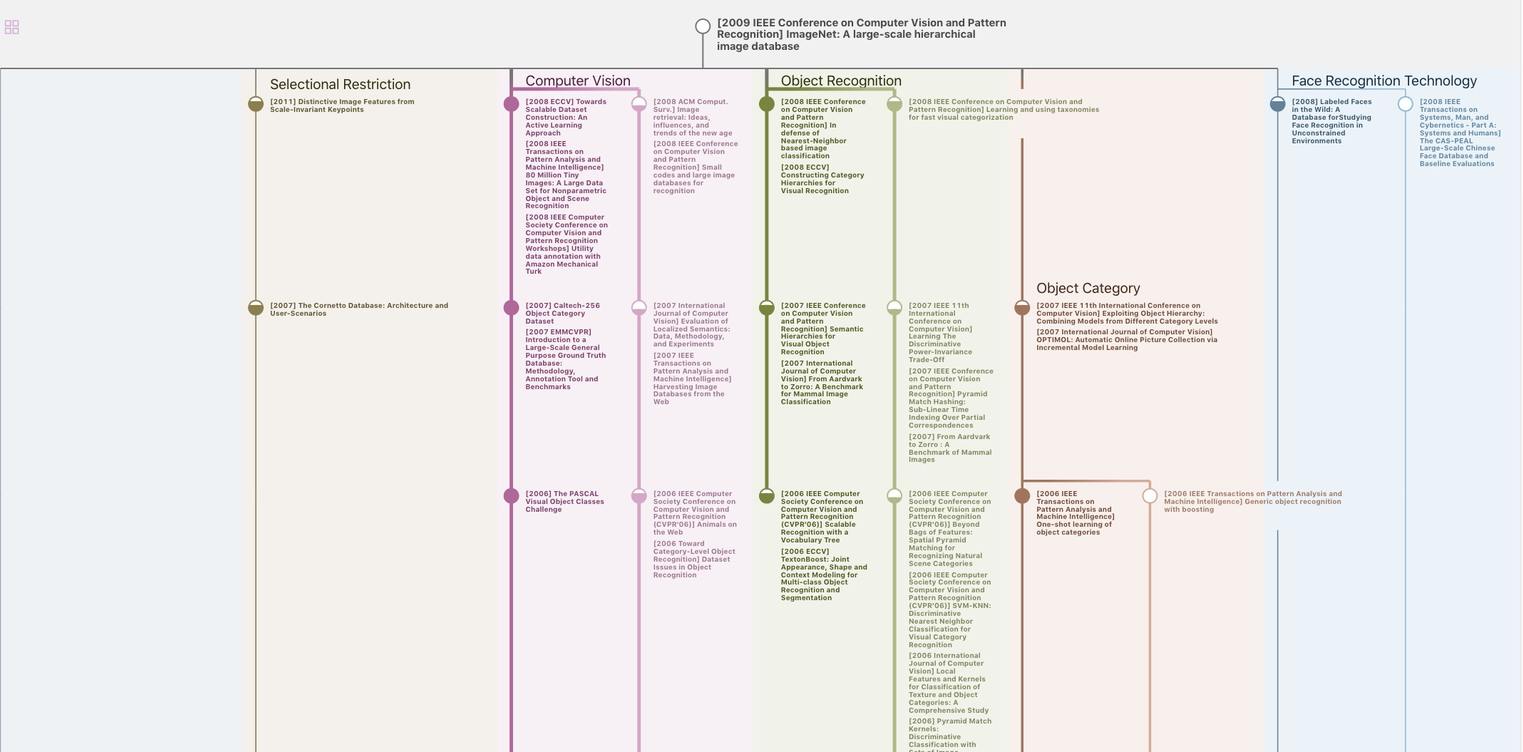
生成溯源树,研究论文发展脉络
Chat Paper
正在生成论文摘要