Achieving Efficient and Privacy-Preserving ($\alpha,\beta$ α , β )-Core Query Over Bipartite Graphs in Cloud
IEEE Transactions on Dependable and Secure Computing(2023)
摘要
Bipartite graphs have been widely adopted in applications such as e-healthcare and recommendation systems thanks to their ability to model various real-world relationships. Meanwhile,
$(\alpha,\beta)$
-core query services over bipartite graphs are generally recognized as a promising approach for finding communities, i.e., sets of closely related vertices, in a bipartite graph. As the bipartite graph grows, the service providers tend to outsource the services to the cloud for flexible and highly reliable computational resources. However, since the cloud is not fully trustable, there are privacy concerns related to the dataset, query requests, and results. Although many schemes have been proposed for privacy-preserving queries over graphs, they cannot be directly adopted to handle accurate
$(\alpha,\beta)$
-core queries over bipartite graphs. Aiming at the challenges, under the two-server setting, this paper constructs two privacy-preserving schemes with different levels of security to handle
$(\alpha,\beta)$
-core queries over the bipartite graph. Specifically, in the proposed schemes, a graph is represented as an index containing an edge table and a node table and further encrypted by a symmetric homomorphic encryption scheme, and then the two servers securely traverse the index. Detailed security analysis shows that both schemes can achieve access pattern privacy, while the security-enhanced one can further protect the structure privacy of the query requests and results. In addition, extensive performance evaluations are conducted, and the results also indicate our proposed schemes are computationally efficient.
更多查看译文
关键词
Community detection,(α,β)-core query,bipartite graph,privacy-preserving
AI 理解论文
溯源树
样例
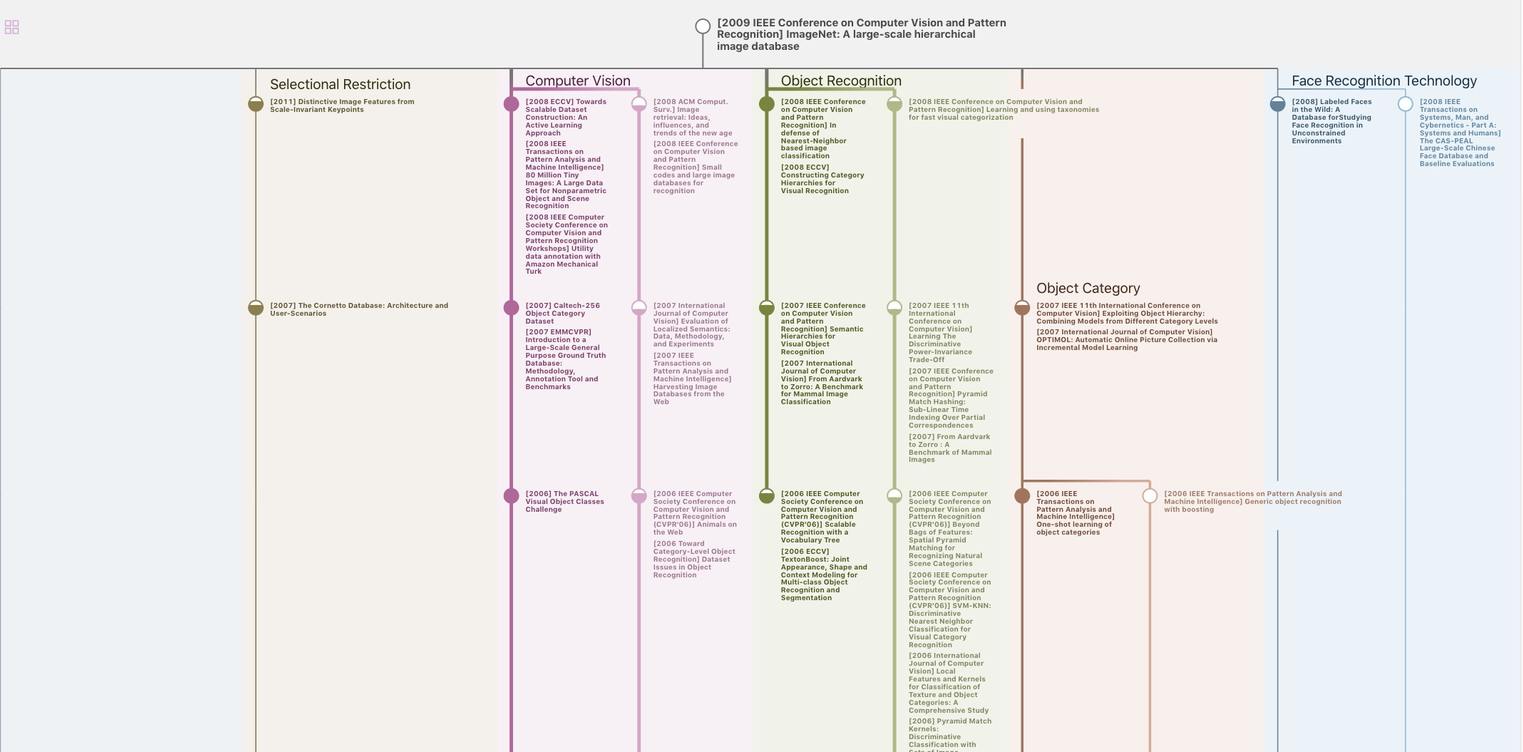
生成溯源树,研究论文发展脉络
Chat Paper
正在生成论文摘要