An Empirical Study on Google Research Football Multi-agent Scenarios
CoRR(2024)
摘要
Few multi-agent reinforcement learning (MARL) researches on Google research football (GRF) focus on the 11-vs-11 multi-agent full-game scenario and to the best of our knowledge, no open benchmark on this scenario has been released to the public. In this work, we fill the gap by providing a population-based MARL training pipeline and hyperparameter settings on multi-agent football scenario that outperforms the bot with difficulty 1.0 from scratch within 2 million steps. Our experiments serve as a reference for the expected performance of independent proximal policy optimization (IPPO), a state-of-the-art multi-agent reinforcement learning algorithm where each agent tries to maximize its own policy independently across various training configurations. Meanwhile, we release our training framework Light-MALib which extends the MALib codebase by distributed and asynchronous implementation with additional analytical tools for football games. Finally, we provide guidance for building strong football AI with population-based training and release diverse pretrained policies for benchmarking. The goal is to provide the community with a head start for whoever experiment their works on GRF and a simple-to-use population-based training framework for further improving their agents through self-play. The implementation is available at https://github.com/Shanghai-Digital-Brain-Laboratory/DB-Football .
更多查看译文
关键词
Multi-agent reinforcement learning (RL),distributed RL system,population-based training,reward shaping,game theory
AI 理解论文
溯源树
样例
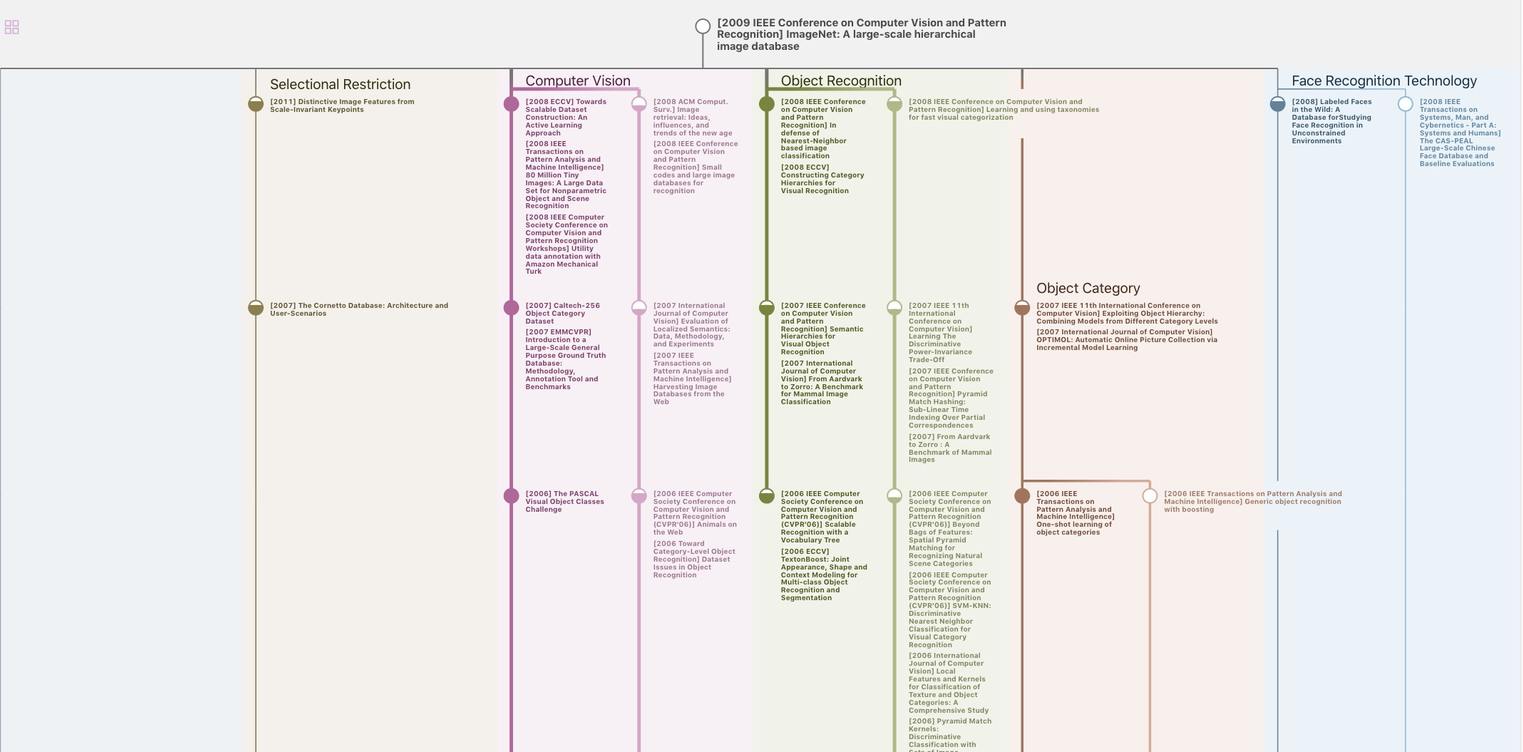
生成溯源树,研究论文发展脉络
Chat Paper
正在生成论文摘要