AdversarialWord Dilution as Text Data Augmentation in Low-Resource Regime
CoRR(2023)
摘要
Data augmentation is widely used in text classification, especially in the low-resource regime where a few examples for each class are available during training. Despite the success, generating data augmentations as hard positive examples that may increase their effectiveness is under-explored. This paper proposes an Adversarial Word Dilution (AWD) method that can generate hard positive examples as text data augmentations to train the low-resource text classification model efficiently. Our idea of augmenting the text data is to dilute the embedding of strong positive words by weighted mixing with unknown-word embedding, making the augmented inputs hard to be recognized as positive by the classification model. We adversarially learn the dilution weights through a constrained min-max optimization process with the guidance of the labels. Empirical studies on three benchmark datasets show that AWD can generate more effective data augmentations and outperform the state-of-the-art text data augmentation methods. The additional analysis demonstrates that the data augmentations generated by AWD are interpretable and can flexibly extend to new examples without further training.
更多查看译文
关键词
adversarial word dilution,text data augmentation,data augmentation,low-resource
AI 理解论文
溯源树
样例
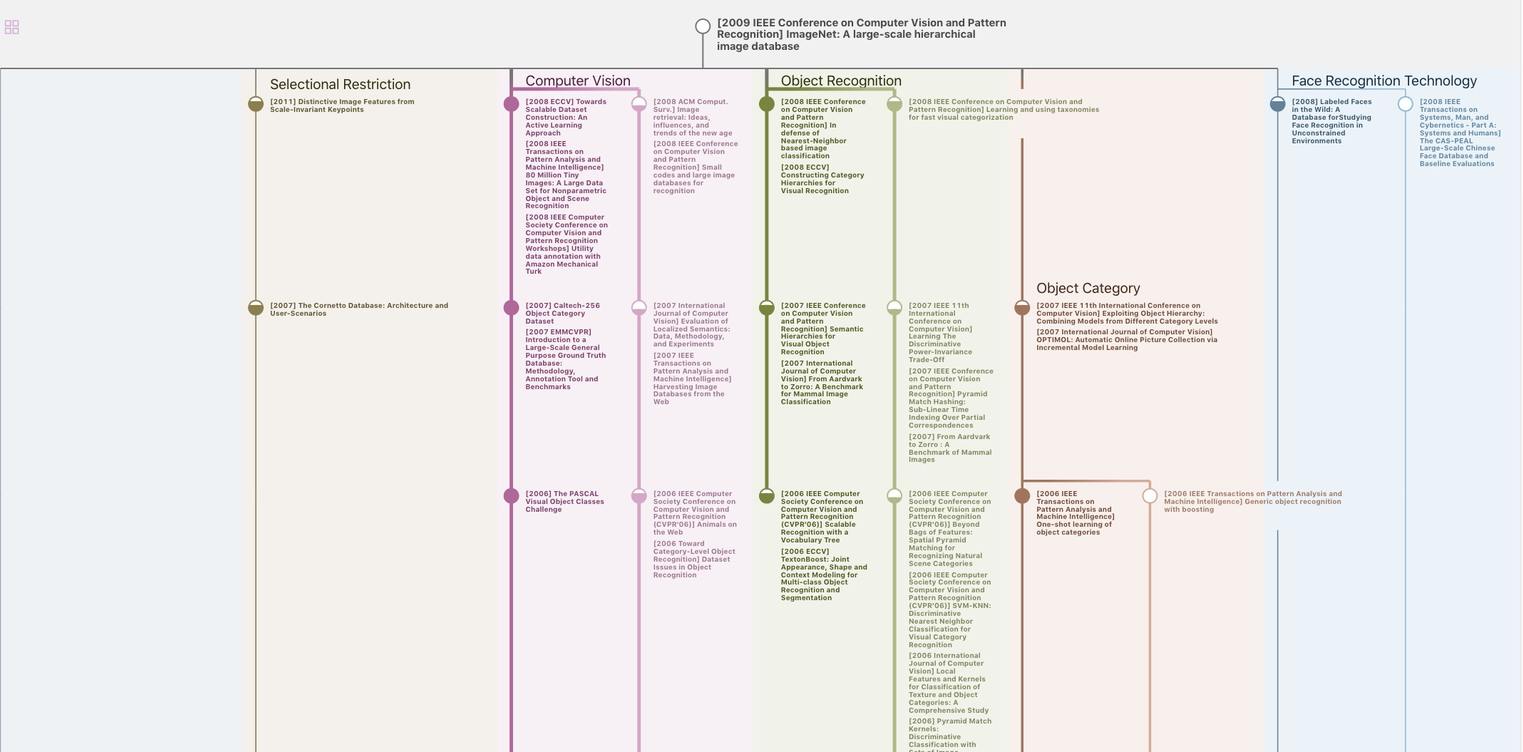
生成溯源树,研究论文发展脉络
Chat Paper
正在生成论文摘要