One-shot neural band selection for spectral recovery
CoRR(2023)
摘要
Band selection has a great impact on the spectral recovery quality. To solve this ill-posed inverse problem, most band selection methods adopt hand-crafted priors or exploit clustering or sparse regularization constraints to find most prominent bands. These methods are either very slow due to the computational cost of repeatedly training with respect to different selection frequencies or different band combinations. Many traditional methods rely on the scene prior and thus are not applicable to other scenarios. In this paper, we present a novel one-shot Neural Band Selection (NBS) framework for spectral recovery. Unlike conventional searching approaches with a discrete search space and a non-differentiable search strategy, our NBS is based on the continuous relaxation of the band selection process, thus allowing efficient band search using gradient descent. To enable the compatibility for se- lecting any number of bands in one-shot, we further exploit the band-wise correlation matrices to progressively suppress similar adjacent bands. Extensive evaluations on the NTIRE 2022 Spectral Reconstruction Challenge demonstrate that our NBS achieves consistent performance gains over competitive baselines when examined with four different spectral recov- ery methods. Our code will be publicly available.
更多查看译文
关键词
Band Selection,Spectral Recovery,Hyperspectral Image Processing
AI 理解论文
溯源树
样例
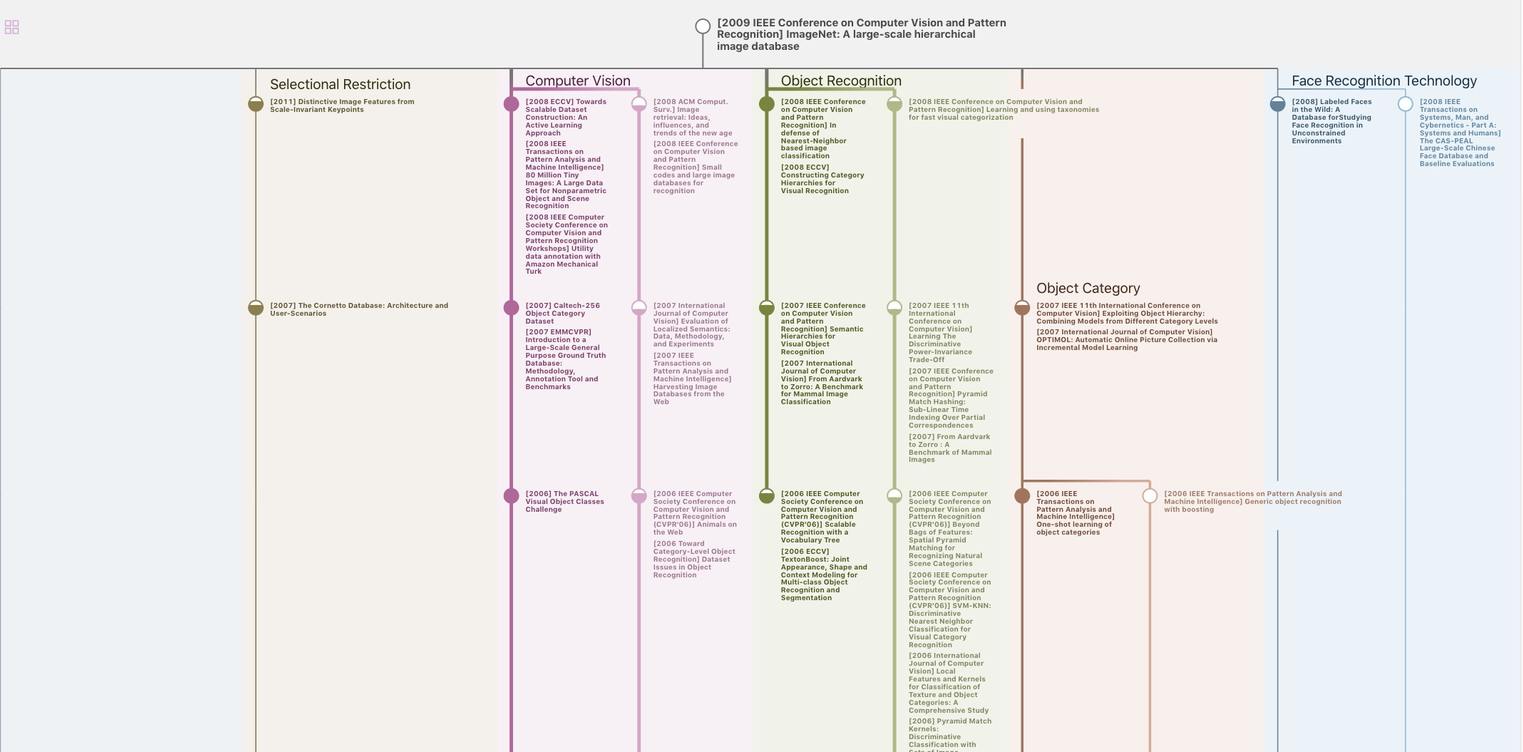
生成溯源树,研究论文发展脉络
Chat Paper
正在生成论文摘要