Progressive Translation: Improving Domain Robustness of Neural Machine Translation with Intermediate Sequences
conf_acl(2023)
摘要
Previous studies show that intermediate supervision signals benefit various Natural Language Processing tasks. However, it is not clear whether there exist intermediate signals that benefit Neural Machine Translation (NMT). Borrowing techniques from Statistical Machine Translation, we propose intermediate signals which are intermediate sequences from the "source-like" structure to the "target-like" structure. Such intermediate sequences introduce an inductive bias that reflects a domain-agnostic principle of translation, which reduces spurious correlations that are harmful to out-of-domain generalisation. Furthermore, we introduce a full-permutation multi-task learning to alleviate the spurious causal relations from intermediate sequences to the target, which results from exposure bias. The Minimum Bayes Risk decoding algorithm is used to pick the best candidate translation from all permutations to further improve the performance. Experiments show that the introduced intermediate signals can effectively improve the domain robustness of NMT and reduces the amount of hallucinations on out-of-domain translation. Further analysis shows that our methods are especially promising in low-resource scenarios.
更多查看译文
关键词
domain robustness,translation,neural machine
AI 理解论文
溯源树
样例
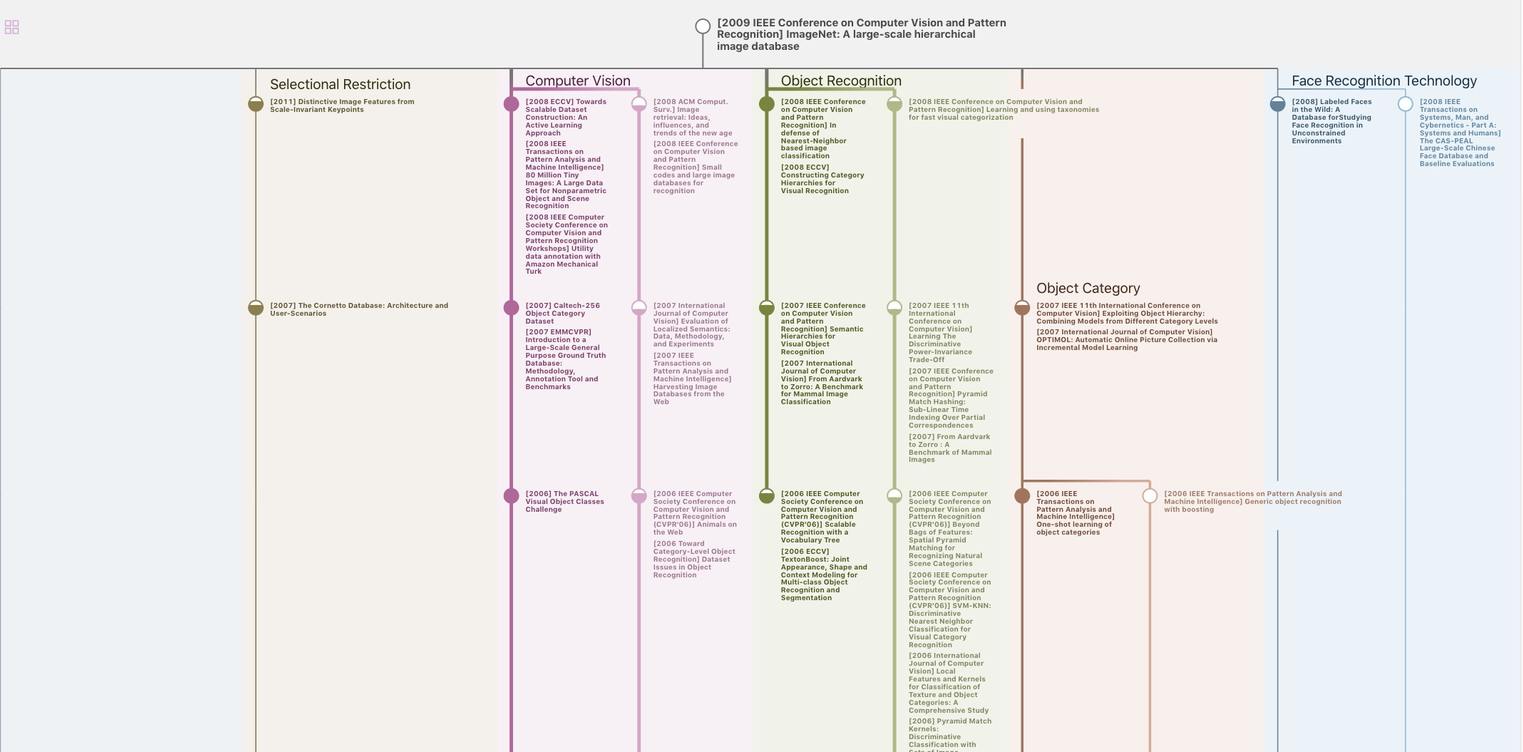
生成溯源树,研究论文发展脉络
Chat Paper
正在生成论文摘要