DualGenerator: Information Interaction-based Generative Network for Point Cloud Completion
CoRR(2023)
摘要
Point cloud completion estimates complete shapes from incomplete point clouds to obtain higher-quality point cloud data. Most existing methods only consider global object features, ignoring spatial and semantic information of adjacent points. They cannot distinguish structural information well between different object parts, and the robustness of models is poor. To tackle these challenges, we propose an information interaction-based generative network for point cloud completion ($\mathbf{DualGenerator}$). It contains an adversarial generation path and a variational generation path, which interact with each other and share weights. DualGenerator introduces a local refinement module in generation paths, which captures general structures from partial inputs, and then refines shape details of the point cloud. It promotes completion in the unknown region and makes a distinction between different parts more obvious. Moreover, we design DGStyleGAN to improve the generation quality further. It promotes the robustness of this network combined with fusion analysis of dual-path completion results. Qualitative and quantitative evaluations demonstrate that our method is superior on MVP and Completion3D datasets. The performance will not degrade significantly after adding noise interference or sparse sampling.
更多查看译文
关键词
point cloud completion,generative network,interaction-based
AI 理解论文
溯源树
样例
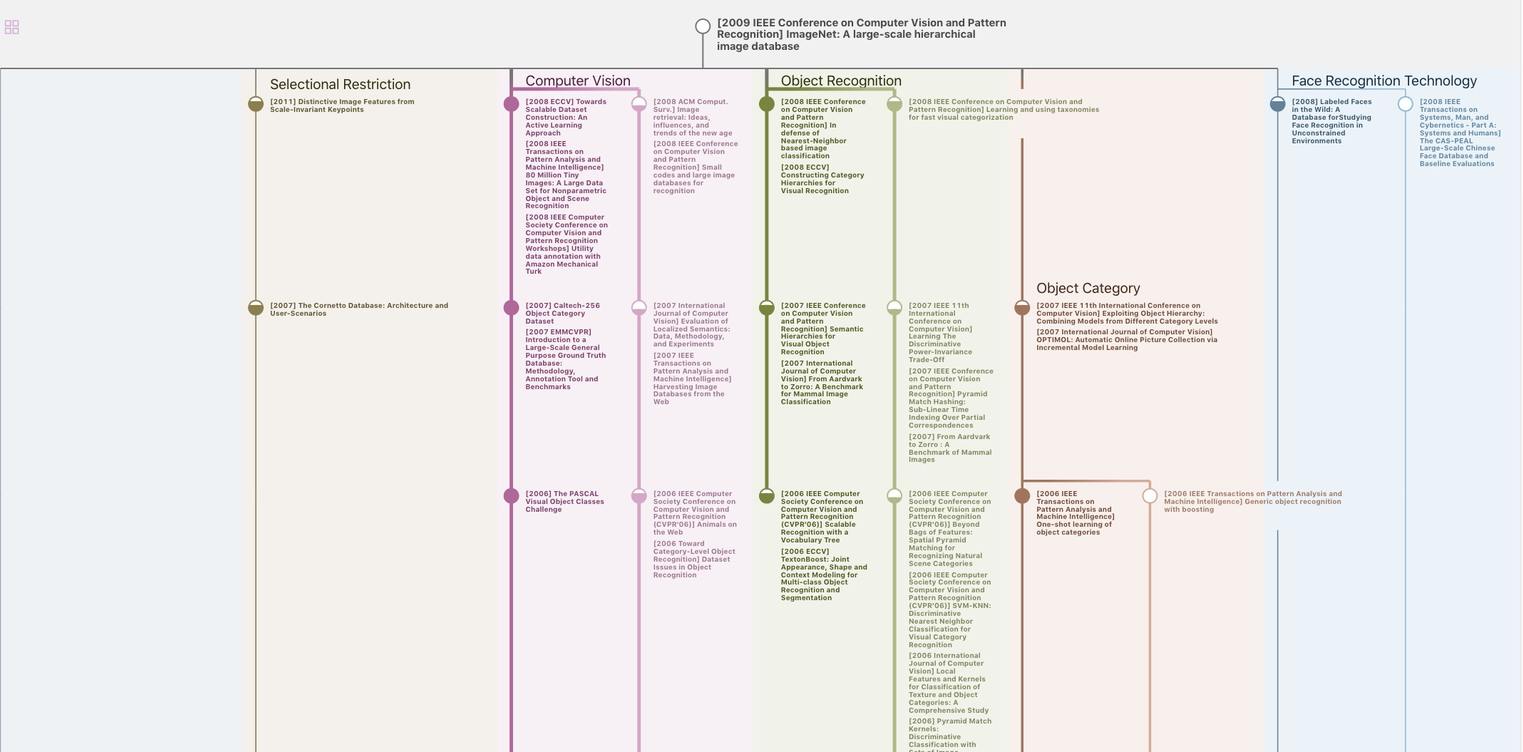
生成溯源树,研究论文发展脉络
Chat Paper
正在生成论文摘要