Denoising Diffusion Models for Plug-and-Play Image Restoration
CoRR(2023)
摘要
Plug-and-play Image Restoration (IR) has been widely recognized as a flexible and interpretable method for solving various inverse problems by utilizing any off-the-shelf denoiser as the implicit image prior. However, most existing methods focus on discriminative Gaussian denoisers. Although diffusion models have shown impressive performance for high-quality image synthesis, their potential to serve as a generative denoiser prior to the plug-and-play IR methods remains to be further explored. While several other attempts have been made to adopt diffusion models for image restoration, they either fail to achieve satisfactory results or typically require an unacceptable number of Neural Function Evaluations (NFEs) during inference. This paper proposes DiffPIR, which integrates the traditional plug-and-play method into the diffusion sampling framework. Compared to plug-and-play IR methods that rely on discriminative Gaussian denoisers, DiffPIR is expected to inherit the generative ability of diffusion models. Experimental results on three representative IR tasks, including super-resolution, image deblurring, and inpainting, demonstrate that DiffPIR achieves state-of-the-art performance on both the FFHQ and ImageNet datasets in terms of reconstruction faithfulness and perceptual quality with no more than 100 NFEs. The source code is available at {\url{https://github.com/yuanzhi-zhu/DiffPIR}}
更多查看译文
关键词
denoising diffusion models,DiffPIR,diffusion sampling framework,discriminative Gaussian denoisers,generative denoiser prior,high-quality image synthesis,image inpainting,image deblurring,implicit image prior,inverse problems,neural function evaluations,NFEs,off-the-shelf denoiser,plug-and-play image restoration,plug-and-play IR methods,super-resolution
AI 理解论文
溯源树
样例
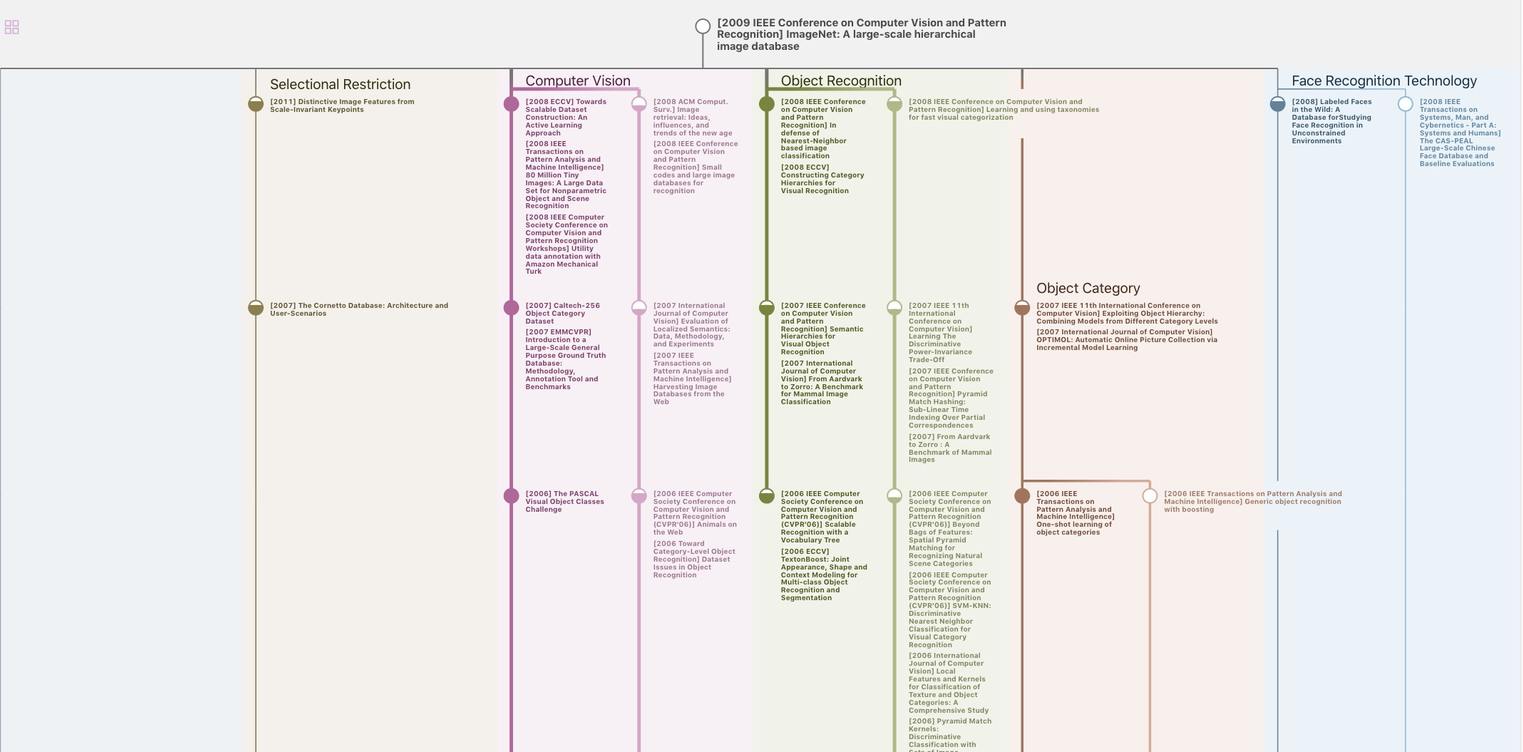
生成溯源树,研究论文发展脉络
Chat Paper
正在生成论文摘要