Multi-label classification with weak labels by learning label correlation and label regularization
Applied Intelligence(2023)
摘要
In conventional multi-label learning, each training instance is associated with multiple available labels. Nevertheless, real-world objects usually exhibit more sophisticated properties such as abundant irrelevant features, incomplete labels, noisy labels, as well as class imbalance. Unfortunately, most existing multi-label learning algorithms only discussed one of them and failed to consider the confounding effects of these factors, which will degrade the accuracy of multi-label classification. In this paper, we propose an integrated multi-label learning framework ML-INC that trains the multi-label model while addressing the aforementioned issues simultaneously. Specifically, we first decompose the observed label matrix into an incomplete ground-truth label matrix and a noisy label matrix by employing the low-rank and sparse decomposition scheme. Secondly, a label confidence matrix is learned to supplement the incomplete label matrix by utilizing the high-order label correlation and the label consistency. Additionally, the low-rank structure is adopted to capture the label correlation. Thirdly, a label regularization matrix is introduced to alleviate the effects of class imbalance in the label matrix, and a sparse constraint is imposed on the feature mapping matrix to select relevant discriminative features. Finally, the Alternating Direction Multiplier Method (ADMM) is employed to handle the optimization problem and comprehensive experiments are conducted to certify the effectiveness of the proposed method.
更多查看译文
关键词
weak labels,multi-label regularization,multi-label correlation,classification
AI 理解论文
溯源树
样例
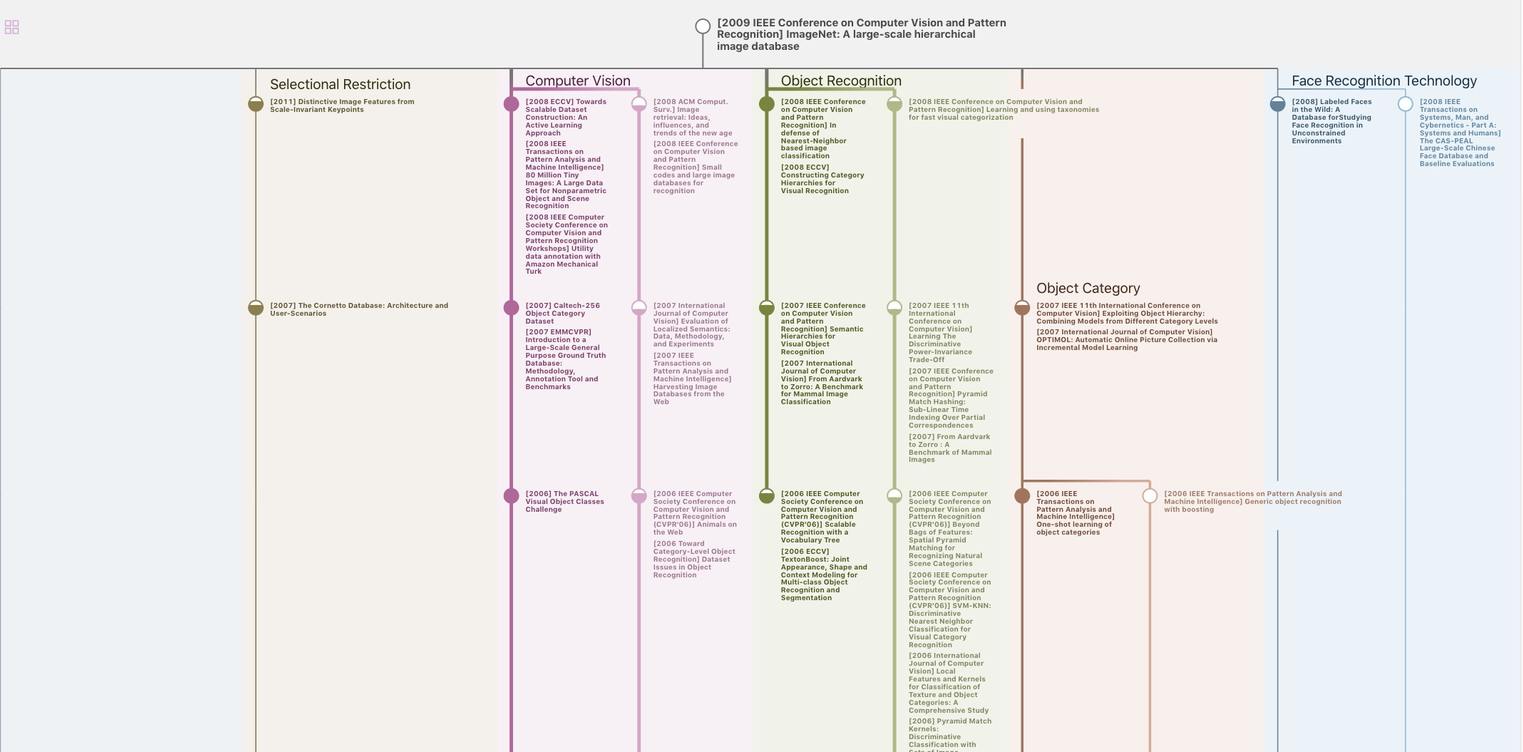
生成溯源树,研究论文发展脉络
Chat Paper
正在生成论文摘要