Learning a Controller for Soft Robotic Arms and Testing its Generalization to New Observations, Dynamics, and Tasks
2023 IEEE International Conference on Soft Robotics (RoboSoft)(2023)
摘要
Recently, learning-based controllers that leverage mechanical models of soft robots have shown promising results. This paper presents a closed-loop controller for dynamic trajectory tracking with a pneumatic soft robotic arm learned via Deep Reinforcement Learning using Proximal Policy Optimization. The control policy was trained in simulation leveraging a dynamic Cosserat rod model of the soft robot. The generalization capabilities of learned controllers are vital for successful deployment in the real world, especially when the encountered scenarios differ from the training environment. We assessed the generalization capabilities of the controller in silico for four tests. The first test involved the dynamic tracking of trajectories that differ significantly in shape and velocity profiles from the training data. Second, we evaluated the robustness of the controller to perpetual external end-point forces for dynamic tracking. For tracking tasks, it was also assessed the generalization to similar materials. Finally, we transferred the control policy without retraining to intercept a moving object with the end-effector. The learned control policy has shown good generalization capabilities in all four tests.
更多查看译文
关键词
Modeling, Control, and Learning for Soft Robots, Learning and Adaptive Systems, Soft Robot Applications.
AI 理解论文
溯源树
样例
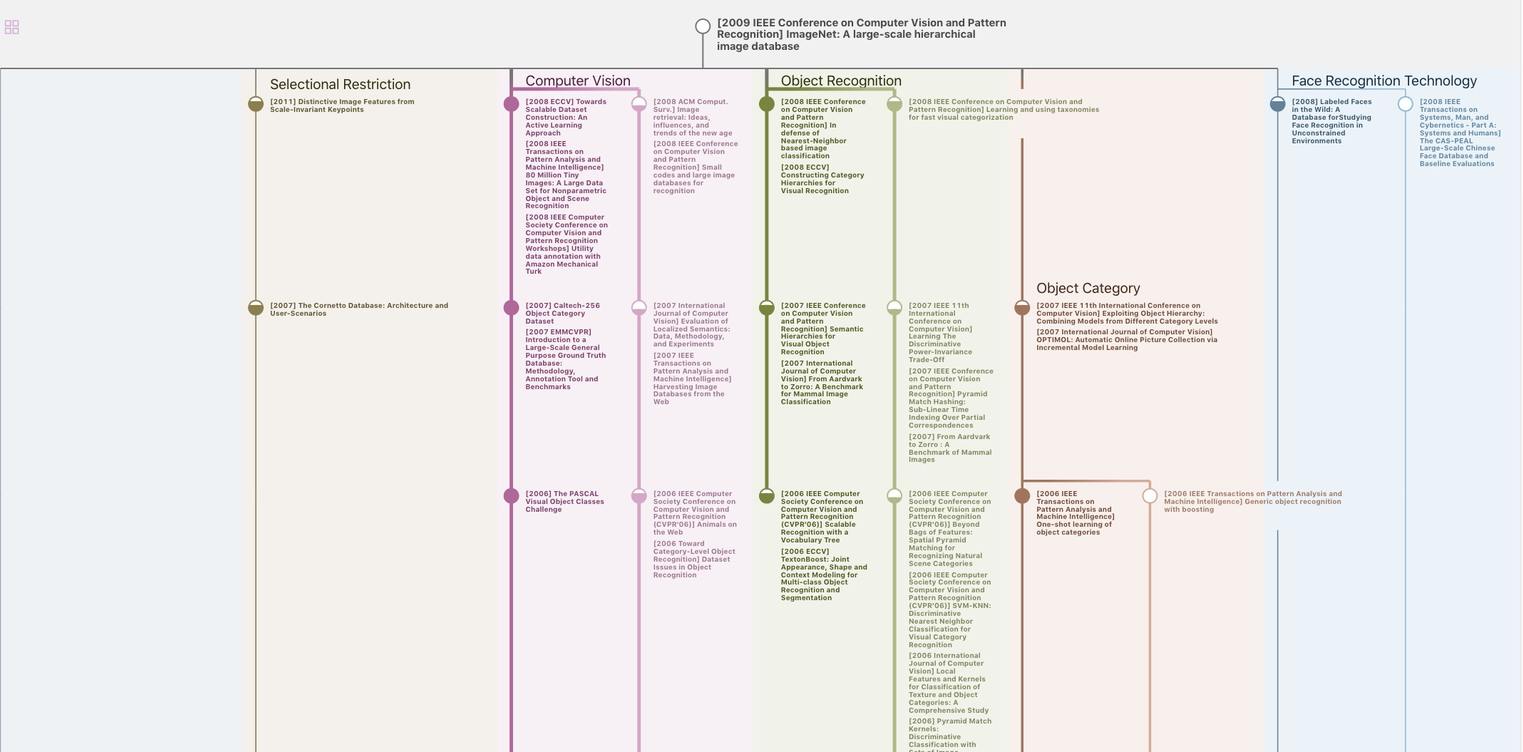
生成溯源树,研究论文发展脉络
Chat Paper
正在生成论文摘要