Elastic modulus estimation of weak rock samples using random forest technique
Bulletin of Engineering Geology and the Environment(2023)
摘要
Many artificial intelligence-based predictive techniques have been developed for assessing elastic modulus ( E ) of rocks using results of simple rock index tests. However, most of them are related to artificial neural networks (ANNs). On the other hand, developing a feasible and easy-to-use model is still of interest more specifically when the datasets of the developed models are based on new experimental data. This study investigates the workability of tree-based techniques including random forest (RF), AdaBoost, extreme gradient boosting, and CatBoost. Utilization of the aforementioned techniques in developing predictive models of E for weak rock samples is relatively new. Nevertheless, for model construction, a suitable database obtained from laboratory experiments on different weak rock types, i.e., marl, siltstone, claystone, and limestone, was prepared. Input parameters of the tree-based model comprises rock index tests which are simple, cheap, and available in any geotechnical laboratory. Then, the aforementioned tree-based models were designed and tuned. Level of performance prediction was assessed using a ranking system which was based on statistical indices such as coefficient of determination (R 2 ), mean absolute error (MAE), and variance account for (VAF). Overall, RF model was able to make a prediction results close to the measured elastic moduli of weak rock samples in laboratory. Additionally, using 30 extra sets of data, the proposed predictive model was validated. R 2 , MAE, and VAF of the validation data were 0.91, 0.597, and 91, respectively which confirm the feasibility of the proposed model in assessing the elastic modulus of weak rocks during site investigation phase.
更多查看译文
关键词
Elastic modulus of rocks,Random forest,Rock index tests,Weak rock,Tree-based models
AI 理解论文
溯源树
样例
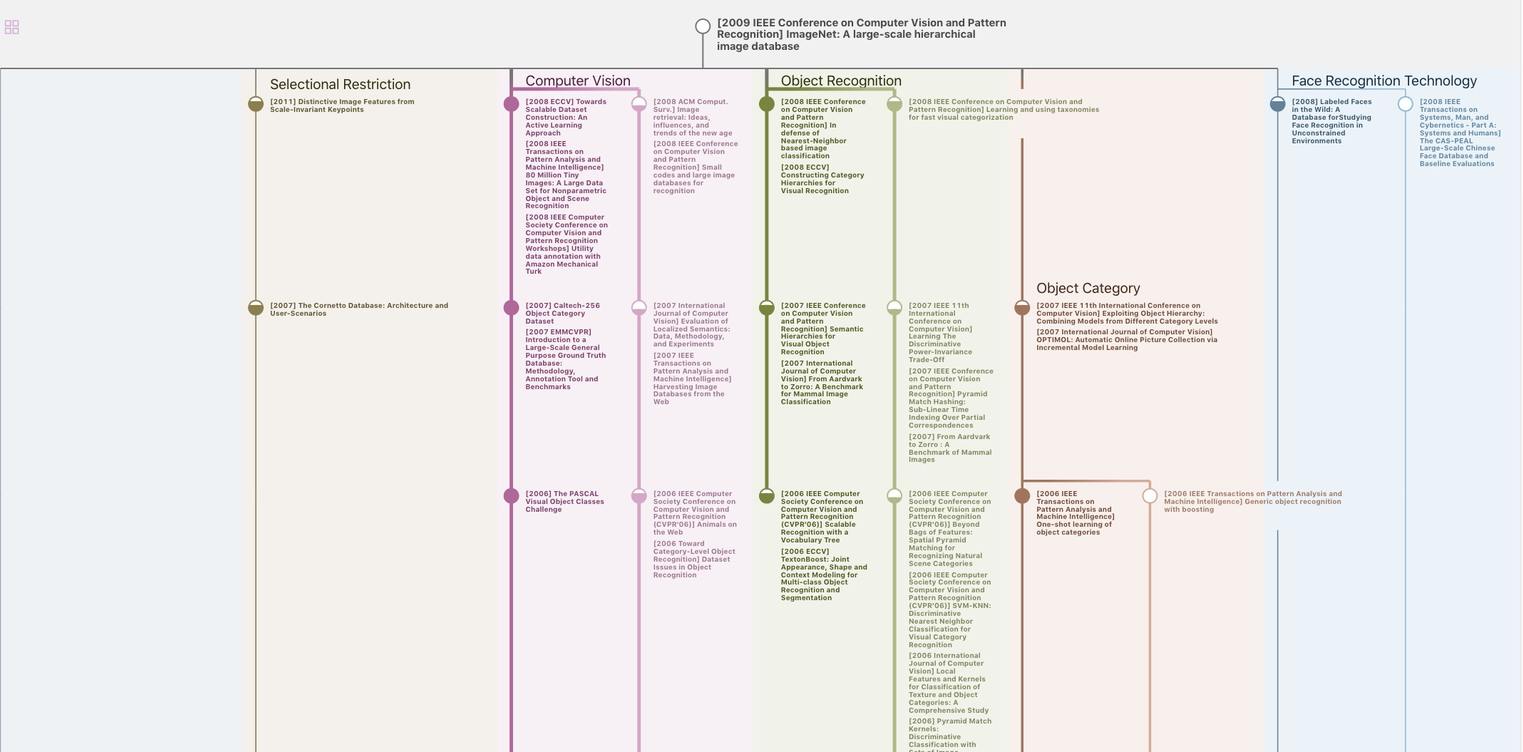
生成溯源树,研究论文发展脉络
Chat Paper
正在生成论文摘要