EDITS: An Easy-to-difficult Training Strategy for Cloud Failure Prediction
COMPANION OF THE WORLD WIDE WEB CONFERENCE, WWW 2023(2023)
摘要
Cloud failures have been a major threat to the reliability of cloud services. Many failure prediction approaches have been proposed to predict cloud failures before they actually occur, so that proactive actions can be taken to ensure service reliability. In industrial practice, existing failure prediction approaches mainly focus on utilizing state-of-the-art time series models to enhance the performance of failure prediction but neglect the training strategy. However, as curriculum learning points out, models perform better when they are trained with data in an order of easy-to-difficult. In this paper, we propose EDITS, a novel training strategy for cloud failure prediction, which greatly improves the performance of the existing cloud failure prediction models. Our experimental results on industrial and public datasets show that EDITS can obviously enhance the performance of cloud failure prediction model. In addition, EDITS also outperforms other curriculum learning methods. More encouragingly, our proposed EDITS has been successfully applied to Microsoft 365 and Azure online service systems, and has obviously reduced financial losses caused by cloud failures.
更多查看译文
AI 理解论文
溯源树
样例
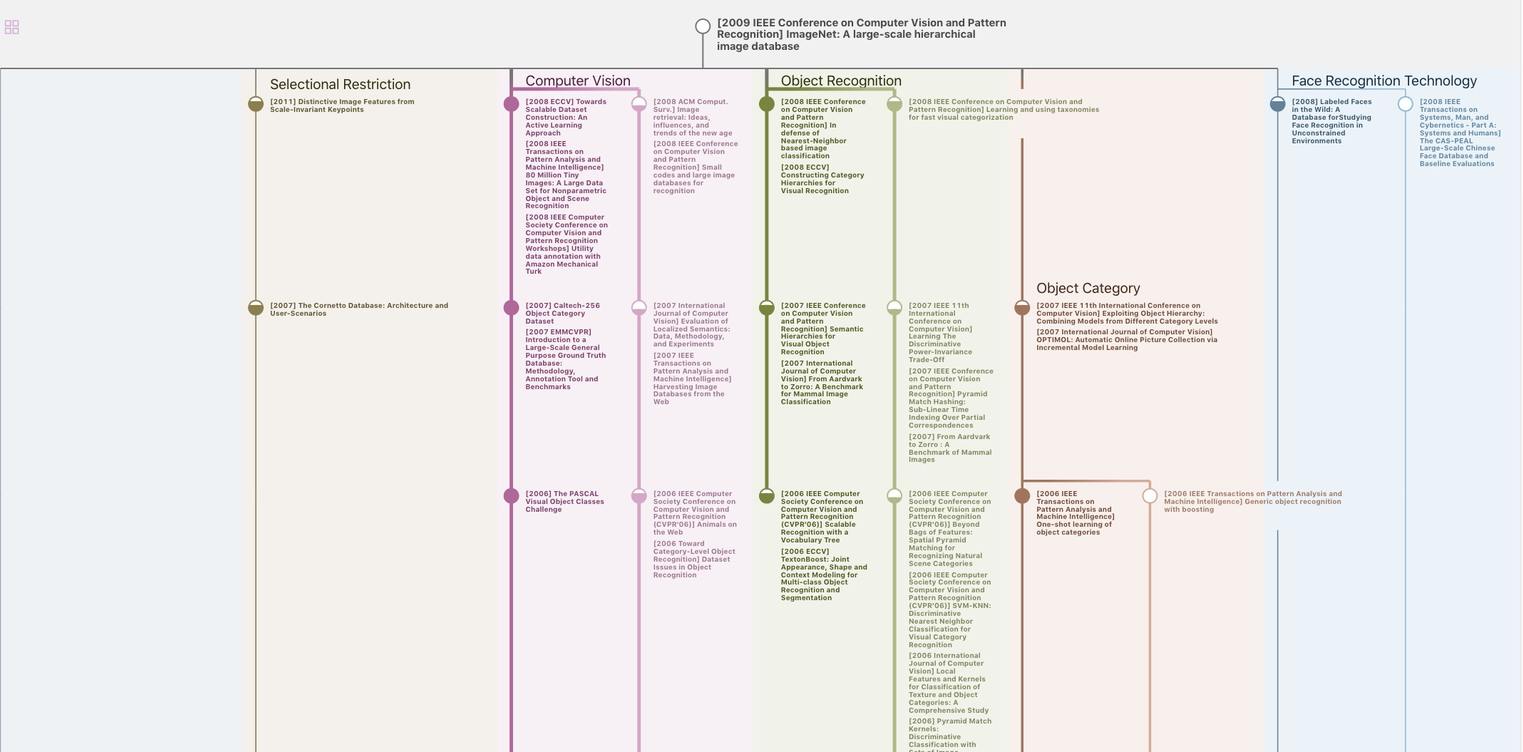
生成溯源树,研究论文发展脉络
Chat Paper
正在生成论文摘要