Long-term electrocardiogram signal quality assessment pipeline based on a frequency-adaptive mean absolute deviation curve
Applied Intelligence(2023)
摘要
Health monitoring is hindered by various types of noise (especially motion artifacts) in electrocardiogram (ECG) collected via wearable devices. The main way to solve this problem is through denoising techniques or signal quality assessment(SQA). When denoising techniques cannot completely suppress motion artifacts, SQA is the most promising approach to address the problem. However, the performance of SQA based on morphological and RR interval features for expressing ECG quality features contaminated by motion artifacts remains unsatisfactory. Here, a frequency-adaptive pipeline based on the mean absolute deviation curve is proposed to achieve a simple and efficient SQA. The greatest advantage of the proposed pipeline is to implement SQA from a new perspective, without considering the influence of motion artifacts or paying attention to the morphological and RR interval features of ECG data. Specifically, the discrete wavelet transform (DWT) is used to capture the abrupt local changes in ECG and noise in a local time window and to form a curve that can reflect the ECG quality distribution. By setting thresholds appropriately (we select two threshold ranges: [0.107,0.143] and [0.324,0.390]), the curve can accurately reflect the ECG quality distribution and label it as three quality levels according to the noise-contamination degrees. On an artificial dataset based on the QT dataset, the F 1 value of our pipeline reaches 97.01%, outperforming the SQA method based on morphology and RR interval features. More importantly, the proposed pipeline achieves SQA without corrupting pathological information, and has great potential for deployment in wearable devices.
更多查看译文
关键词
Mean Absolute Deviation(MAD),E Estimation,Frequency-adaptive,Electrocardiogram(ECG),Signal Quality Assessment(SQA)
AI 理解论文
溯源树
样例
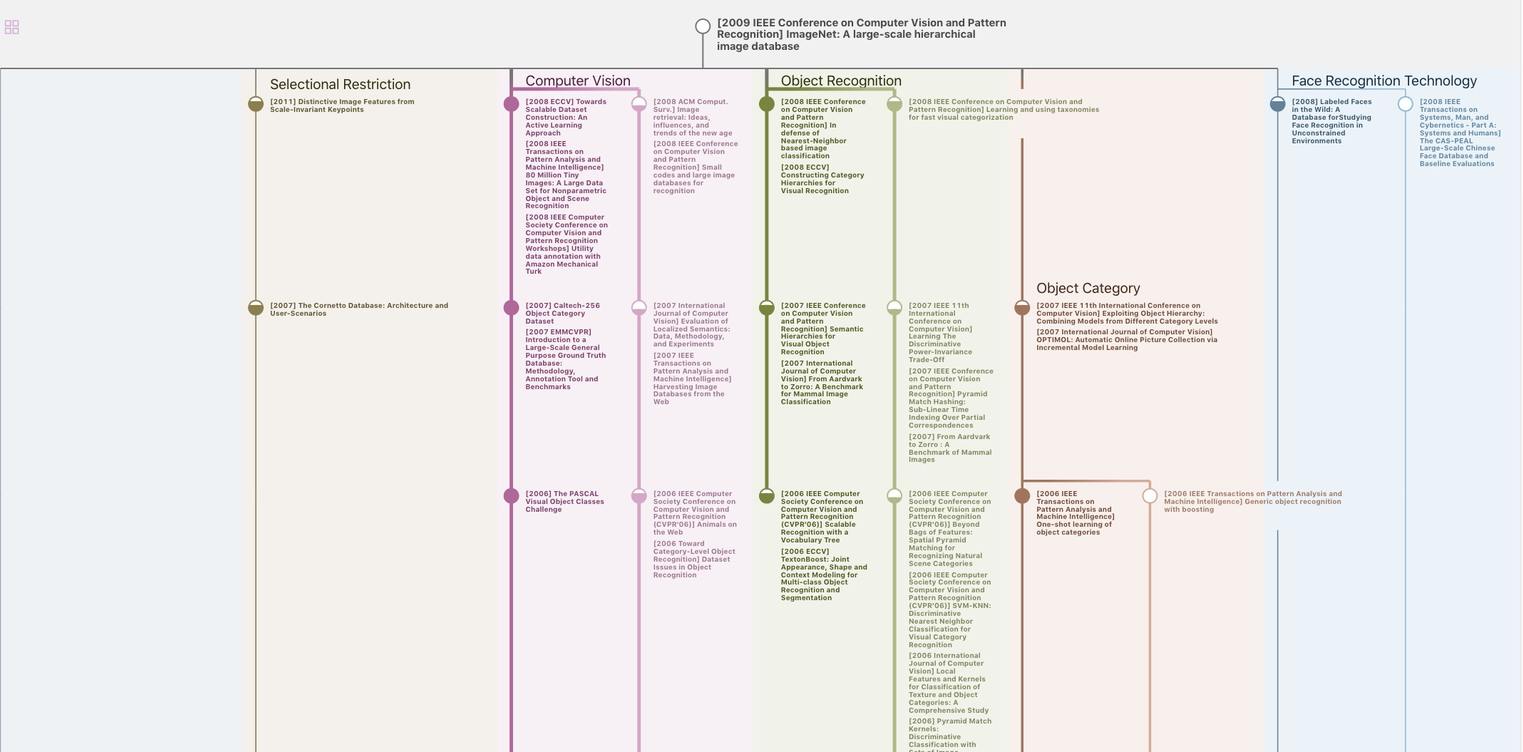
生成溯源树,研究论文发展脉络
Chat Paper
正在生成论文摘要