Deep Learning Provenance Data Integration: a Practical Approach
COMPANION OF THE WORLD WIDE WEB CONFERENCE, WWW 2023(2023)
摘要
A Deep Learning (DL) life cycle involves several data transformations, such as performing data pre-processing, defning datasets to train and test a deep neural network (DNN), and training and evaluating the DL model. Choosing a fnal model requires DL model selection, which involves analyzing data from several training configurations (e.g. hyperparameters and DNN architectures). Tracing training data back to pre-processing operations can provide insights into the model selection step. Provenance is a natural solution to represent data derivation of the whole DL life cycle. However, there are challenges in providing an integration of the provenance of these diferent steps. There are a few approaches to capturing and integrating provenance data from the DL life cycle, but they require that the same provenance capture solution is used along all the steps, which can limit interoperability and fexibility when choosing the DL environment. Therefore, in this work, we present a prototype for provenance data integration using diferent capture solutions. We show use cases where the integrated provenance from pre-processing and training steps can show how data pre-processing decisions infuenced the model selection. Experiments were performed using real-world datasets to train a DNN and provided evidence of the integration between the considered steps, answering queries such as how the data used to train a model that achieved a specifc result was processed.
更多查看译文
关键词
Provenance,Deep Learning,Data Pre-processing
AI 理解论文
溯源树
样例
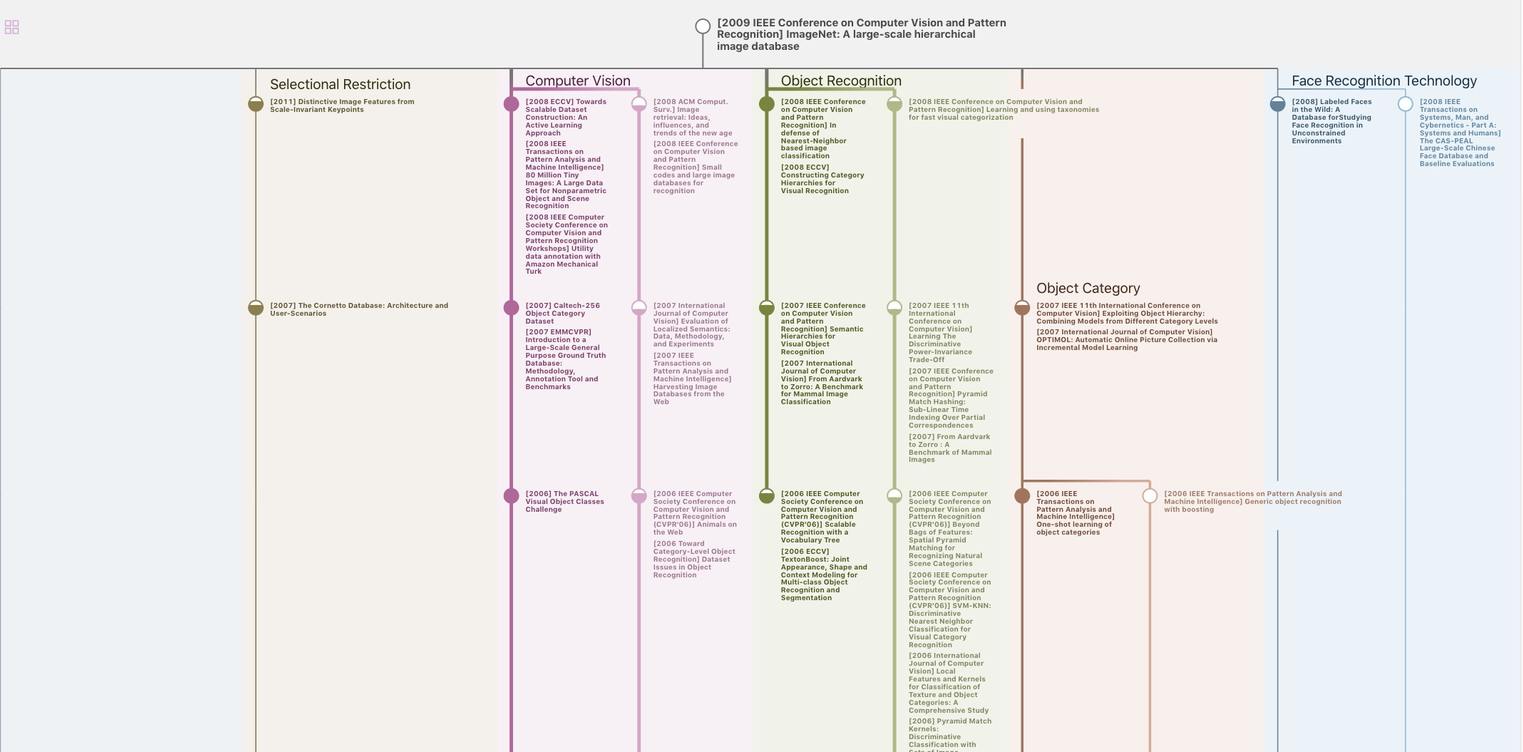
生成溯源树,研究论文发展脉络
Chat Paper
正在生成论文摘要