CLIME: Completeness-Constrained LIME
COMPANION OF THE WORLD WIDE WEB CONFERENCE, WWW 2023(2023)
摘要
We evaluate two popular local explainability techniques, LIME and SHAP, on a movie recommendation task. We discover that the two methods behave very differently depending on the sparsity of the data set, where sparsity is defined by the amount of historical viewing data available to explain a movie recommendation for a particular data instance. We find that LIME does better than SHAP in dense segments of the data set and SHAP does better in sparse segments. We trace this difference to the differing bias-variance characteristics of the underlying estimators of LIME and SHAP. We find that SHAP exhibits lower variance in sparse segments of the data compared to LIME. We attribute this lower variance to the completeness constraint property inherent in SHAP and missing in LIME. This constraint acts as a regularizer and therefore increases the bias of the SHAP estimator but decreases its variance, leading to a favorable bias-variance trade-of especially in high sparsity data settings. With this insight, we introduce the same constraint into LIME and formulate a novel local explainabilty framework called Completeness-Constrained LIME (CLIME) that is superior to LIME and much faster than SHAP.
更多查看译文
关键词
Explainability,personalization,transparency
AI 理解论文
溯源树
样例
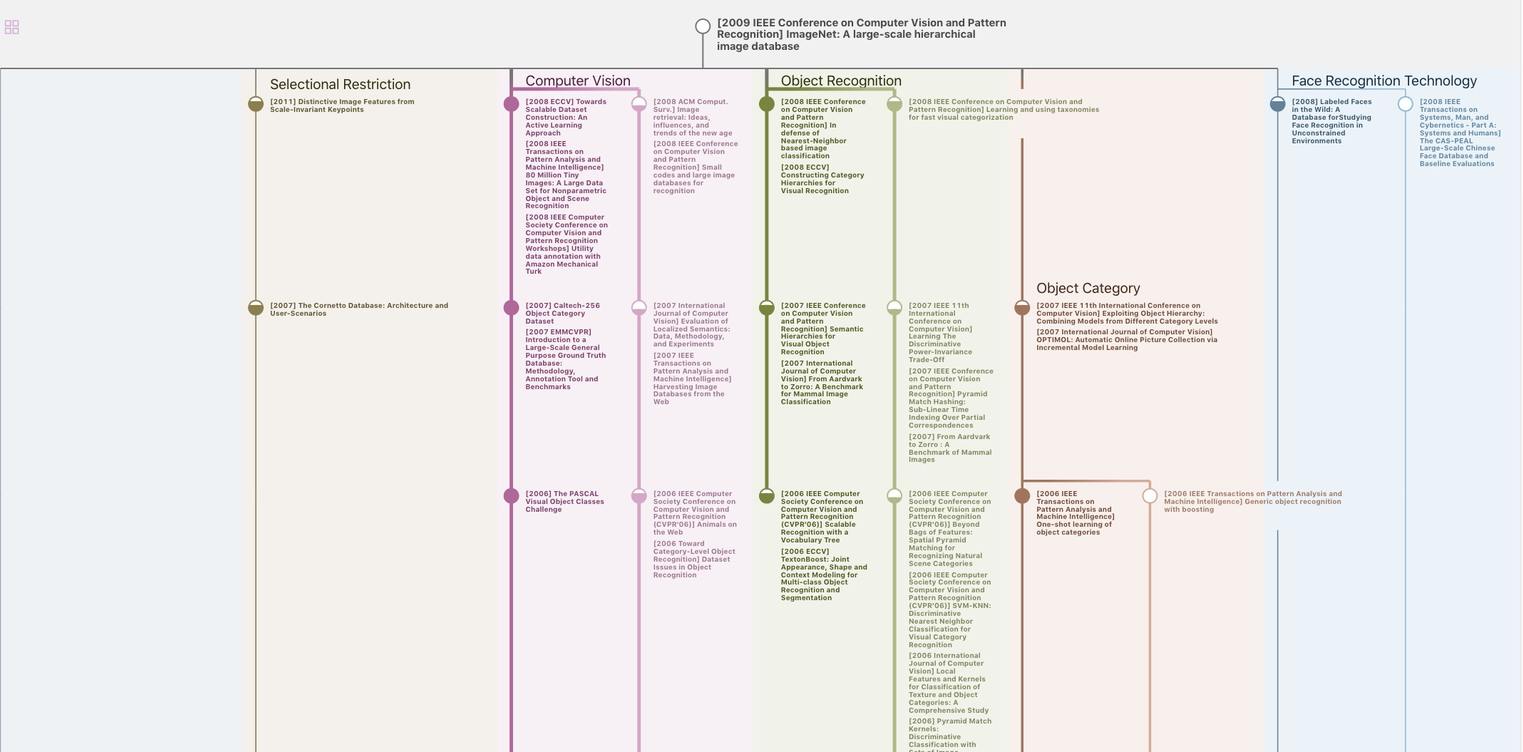
生成溯源树,研究论文发展脉络
Chat Paper
正在生成论文摘要