Analyzing COVID-Related Social Discourse on Twitter using Emotion, Sentiment, Political Bias, Stance, Veracity and Conspiracy Theories
COMPANION OF THE WORLD WIDE WEB CONFERENCE, WWW 2023(2023)
摘要
Online misinformation has become a major concern in recent years, and it has been further emphasized during the COVID-19 pandemic. Social media platforms, such as Twitter, can be serious vectors of misinformation online. In order to better understand the spread of these fake-news, lies, deceptions, and rumours, we analyze the correlations between the following textual features in tweets: emotion, sentiment, political bias, stance, veracity and conspiracy theories. We train several transformer-based classifiers from multiple datasets to detect these textual features and identify potential correlations using conditional distributions of the labels. Our results show that the online discourse regarding some topics, such as COVID-19 regulations or conspiracy theories, is highly controversial and reflects the actual U.S. political landscape.
更多查看译文
关键词
Natural Language Processing,Transformers,BERT,COVID-datasets,Misinformation
AI 理解论文
溯源树
样例
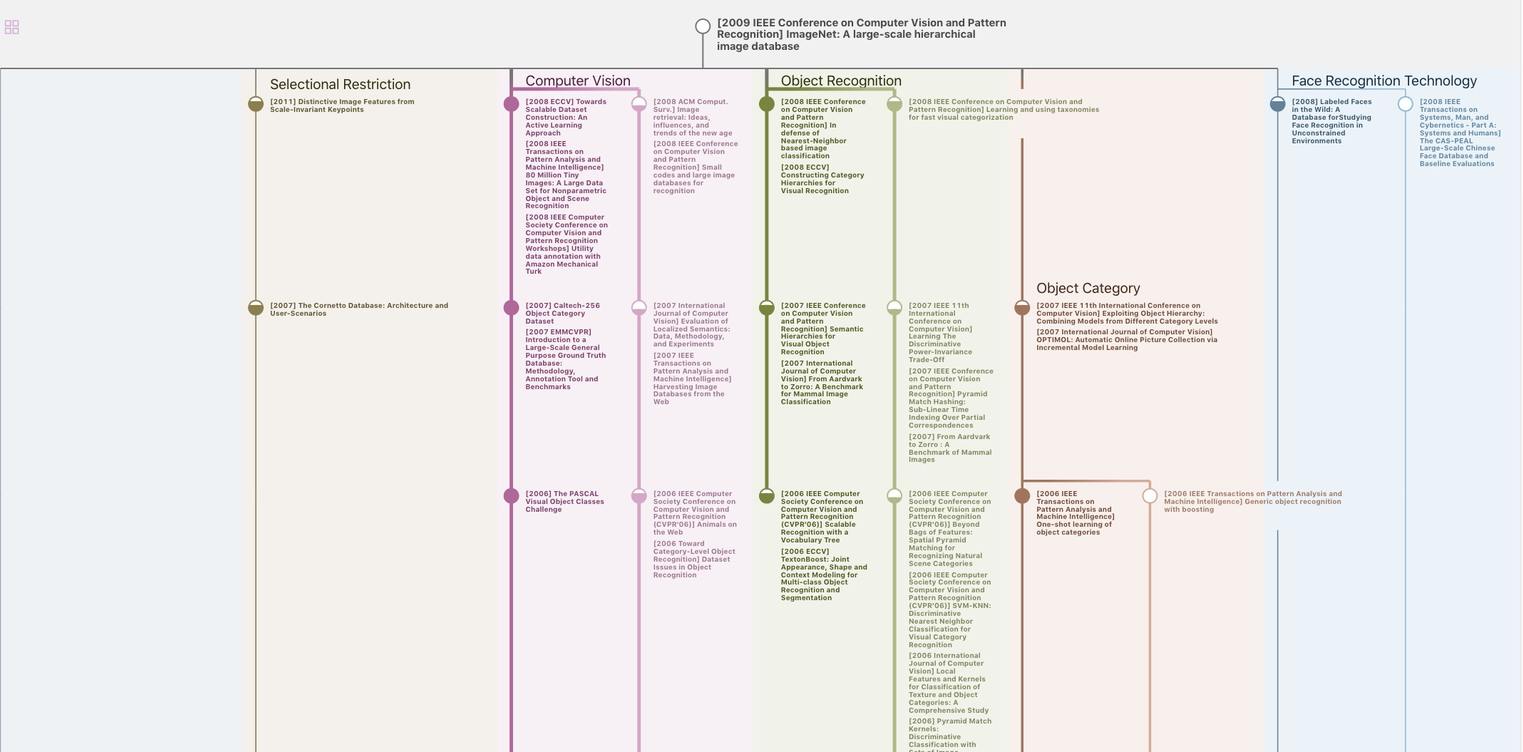
生成溯源树,研究论文发展脉络
Chat Paper
正在生成论文摘要