ReLU soothes the NTK condition number and accelerates optimization for wide neural networks
CoRR(2023)
摘要
Rectified linear unit (ReLU), as a non-linear activation function, is well known to improve the expressivity of neural networks such that any continuous function can be approximated to arbitrary precision by a sufficiently wide neural network. In this work, we present another interesting and important feature of ReLU activation function. We show that ReLU leads to: {\it better separation} for similar data, and {\it better conditioning} of neural tangent kernel (NTK), which are closely related. Comparing with linear neural networks, we show that a ReLU activated wide neural network at random initialization has a larger angle separation for similar data in the feature space of model gradient, and has a smaller condition number for NTK. Note that, for a linear neural network, the data separation and NTK condition number always remain the same as in the case of a linear model. Furthermore, we show that a deeper ReLU network (i.e., with more ReLU activation operations), has a smaller NTK condition number than a shallower one. Our results imply that ReLU activation, as well as the depth of ReLU network, helps improve the gradient descent convergence rate, which is closely related to the NTK condition number.
更多查看译文
关键词
wide neural networks,ntk condition number,neural networks,optimization
AI 理解论文
溯源树
样例
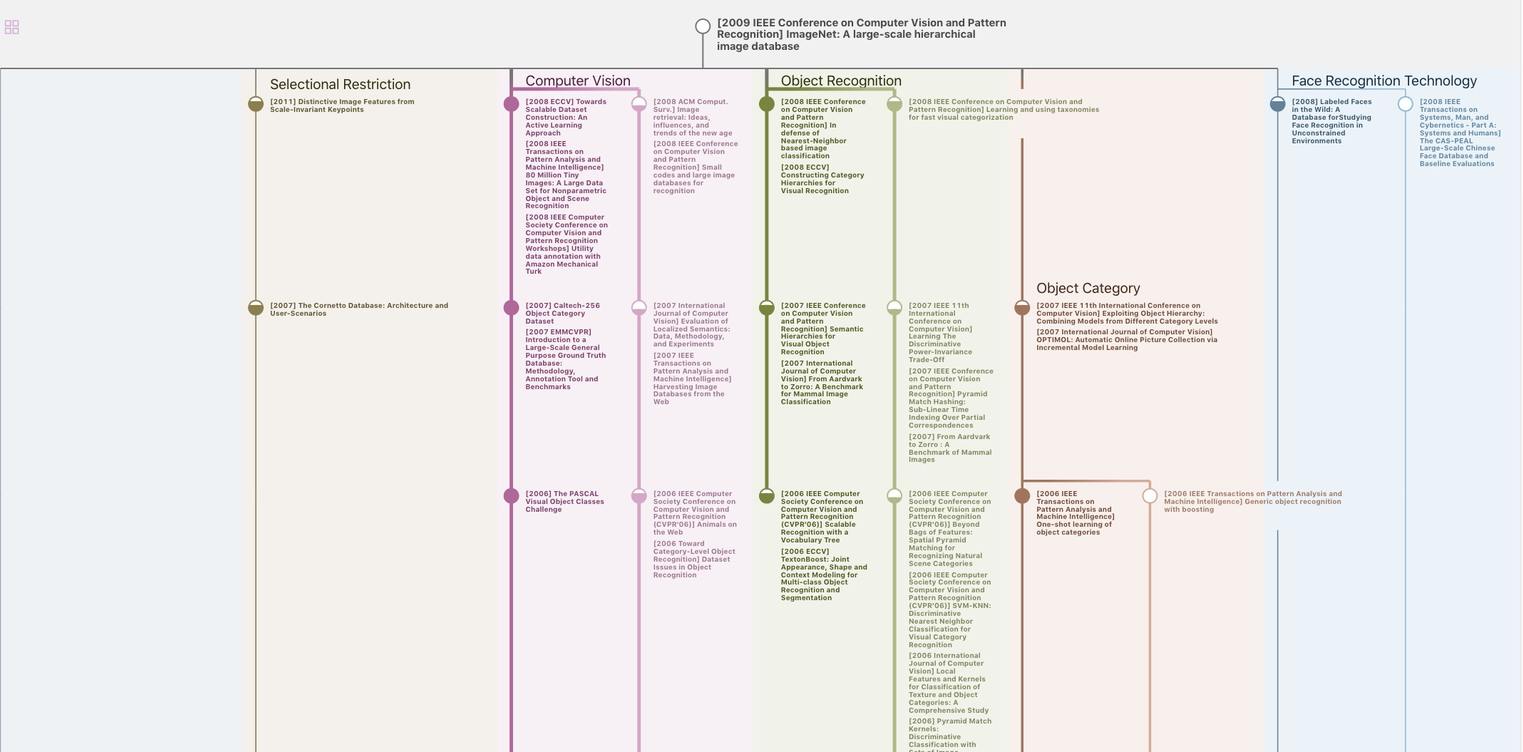
生成溯源树,研究论文发展脉络
Chat Paper
正在生成论文摘要