Evaluating Splitting Approaches in the Context of Student Dropout Prediction
CoRR(2023)
摘要
The prediction of academic dropout, with the aim of preventing it, is one of the current challenges of higher education institutions. Machine learning techniques are a great ally in this task. However, attention is needed in the way that academic data are used by such methods, so that it reflects the reality of the prediction problem under study and allows achieving good results. In this paper, we study strategies for splitting and using academic data in order to create training and testing sets. Through a conceptual analysis and experiments with data from a public higher education institution, we show that a random proportional data splitting, and even a simple temporal splitting are not suitable for dropout prediction. The study indicates that a temporal splitting combined with a time-based selection of the students' incremental academic histories leads to the best strategy for the problem in question.
更多查看译文
关键词
student dropout,splitting approaches,prediction
AI 理解论文
溯源树
样例
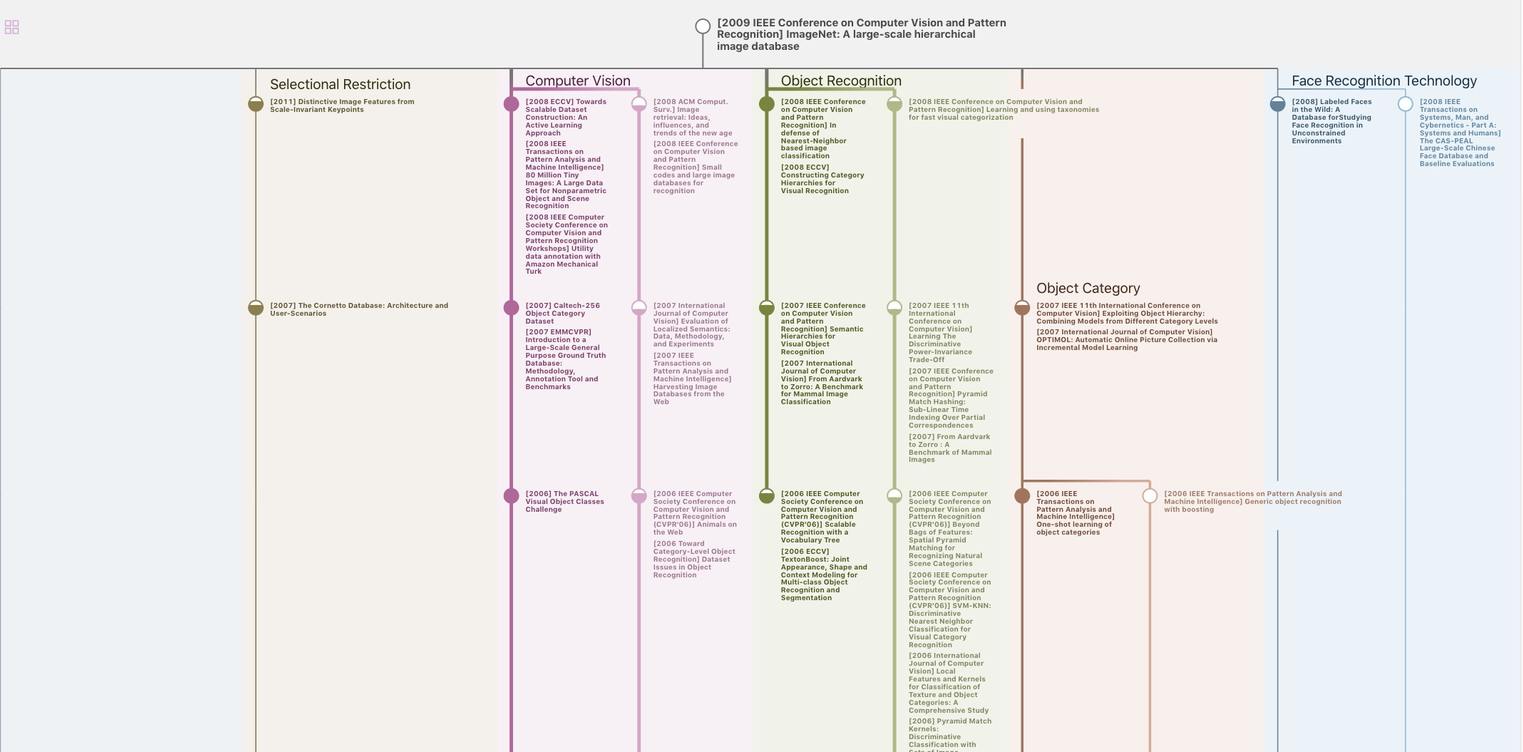
生成溯源树,研究论文发展脉络
Chat Paper
正在生成论文摘要