Nearly Optimal VC-Dimension and Pseudo-Dimension Bounds for Deep Neural Network Derivatives
NeurIPS(2023)
摘要
This paper addresses the problem of nearly optimal Vapnik--Chervonenkis dimension (VC-dimension) and pseudo-dimension estimations of the derivative functions of deep neural networks (DNNs). Two important applications of these estimations include: 1) Establishing a nearly tight approximation result of DNNs in the Sobolev space; 2) Characterizing the generalization error of machine learning methods with loss functions involving function derivatives. This theoretical investigation fills the gap of learning error estimations for a wide range of physics-informed machine learning models and applications including generative models, solving partial differential equations, operator learning, network compression, distillation, regularization, etc.
更多查看译文
关键词
deep neural
AI 理解论文
溯源树
样例
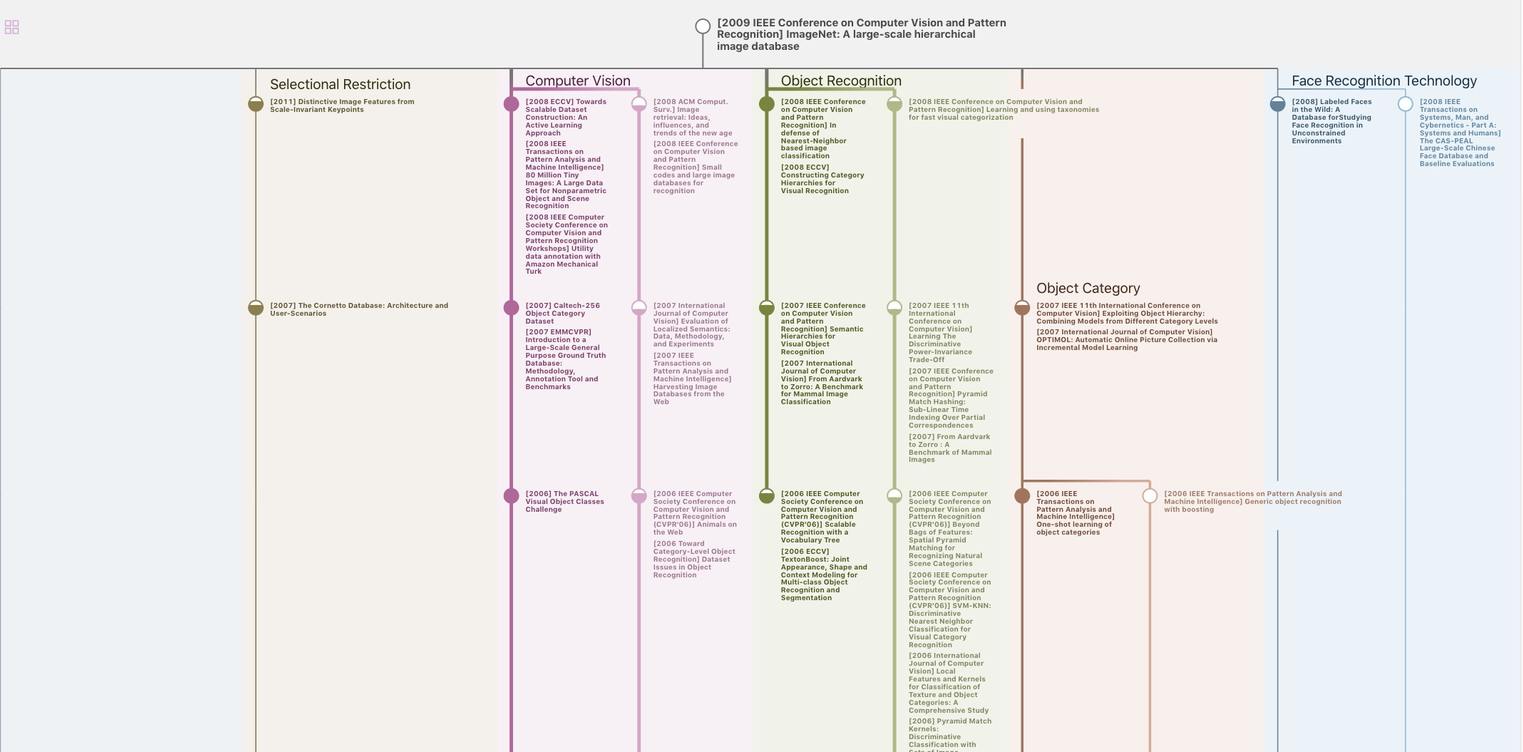
生成溯源树,研究论文发展脉络
Chat Paper
正在生成论文摘要